Machine learning and postoperative complications: Determining risk before surgery in patients with cancer
JOURNAL OF CLINICAL ONCOLOGY(2023)
摘要
6575 Background: In patients with advanced cancer who need unplanned surgery, shared decision making is complex. Surgical risk assessment with high accuracy and in real time may help improve prediction of postoperative outcomes. We previously trained and tested an explainable machine learning model (MLM) to estimate postoperative Clavien-Dindo grade 3 or higher (CD 3+) complication risk in cancer inpatients undergoing unplanned surgery. In this prospective validation study, we report the performance of the MLM Surgical Risk Score (SRS) in the prediction of postoperative outcomes as well as determine association of the risk score with hospital length of stay and mortality. Methods: A MLM SRS for cancer inpatients requiring an unplanned, same-hospitalization major surgery was generated using real-time patient specific EHR data. The SRS was integrated into surgeon preoperative workflow. Postoperative outcomes including complications, length of hospital stay, and 30-day mortality were reported. The SRS was dichotomized as high or low based on prior validation threshold for postoperative CD3+ complication development. Model performance was assessed with area under receiver operating characteristic (AUROC) and area under precision-recall curves (AUPRC). Differences in outcomes between high and low-risk SRS groups were studied using Mann-Whitney U test and Fisher's exact test. Results: During the study period, 135 cancer inpatients were assessed by surgeons for 166 major unplanned operations. Of the 166 operations, 126 were completed and 40 were cancelled. The SRS was high in 81 (49%) and low in 85 (51%). The most common cancer diagnosis was colorectal (n=39, 28.8%). Operations performed with the highest frequencies were for enteral access (e.g.gastrostomy) followed by exploratory laparotomy and enterectomy. CD 3+ complications were identified in 31% of the completed operations (n=39/126) and included death (n = 10); abscess (n=4); postoperative hemorrhage (n=3); and anastomotic leak (n=2). The SRS predicted CD 3+ complications with a precision of47% and recall of 73%, resulting in an AUROC of 0.75 and AUPRC of 0.58. When comparing patients with high-risk to low-risk SRS, median overall LOS was 10 vs 7 days (p=0.013), median LOS after operation was 9 vs 6 days (p=0.003), 30-day mortality was 19.0% vs 5.3% (p=0.015), and 30-day postoperative mortality was 13.2% vs 3.3% (p=0.04). Conclusions: In this prospective study, preoperative SRS generated from a trained MLM on real-time EHR data demonstrated an excellent ability to predict individual risk of postoperative complications. In addition, patients with high SRS have longer postoperative LOS and increased mortality. By improving discrimination of risk of postoperative complications and informing on treatment outcomes, integration of the MLM SRS into the consenting process may enable better informed decision making in cancer inpatients.
更多查看译文
关键词
postoperative complications,surgery,cancer,machine learning
AI 理解论文
溯源树
样例
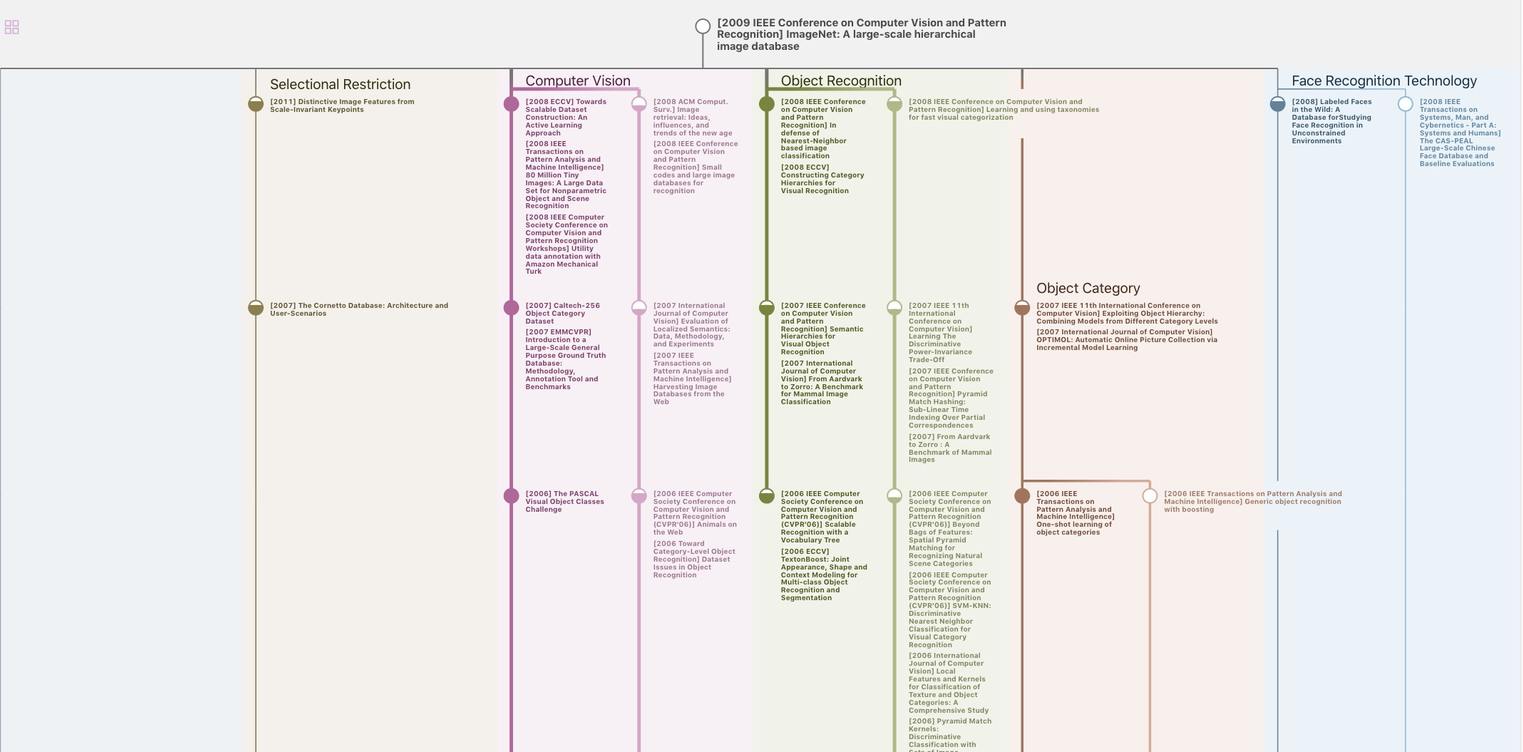
生成溯源树,研究论文发展脉络
Chat Paper
正在生成论文摘要