Intelligent Design of Hairpin Filters Based on Artificial Neural Network and Proximal Policy Optimization
APPLIED SCIENCES-BASEL(2023)
摘要
Microstrip filters are widely used in high-frequency circuit design for signal frequency selection. However, designing these filters often requires extensive trial and error to achieve the desired performance metrics, leading to significant time costs. In this work, we propose an automated design flow for hairpin filters, a specific type of microstrip filter. We employ artificial neural network (ANN) modeling techniques to predict the circuit performance of hairpin filters, and leverage the efficiency of low-cost models to deploy reinforcement learning agents. Specifically, we use the proximal policy optimization (PPO) reinforcement learning algorithm to learn abstract design actions for the filters, allowing us to achieve automated optimization design. Through simulation results, we demonstrate the effectiveness of the proposed approach. By optimizing the geometric dimensions, we significantly improve the performance metrics of hairpin filters, and the trained agent successfully meets our specified design goals within 5 to 15 design steps. This work serves as a conceptual validation attempt to apply reinforcement learning techniques and pre-trained ANN models to automate MMIC filter design. It exhibits clear advantages in terms of time-saving and performance efficiency when compared to other optimization algorithms.
更多查看译文
关键词
hairpin filter, reinforcement learning, proximal policy optimization
AI 理解论文
溯源树
样例
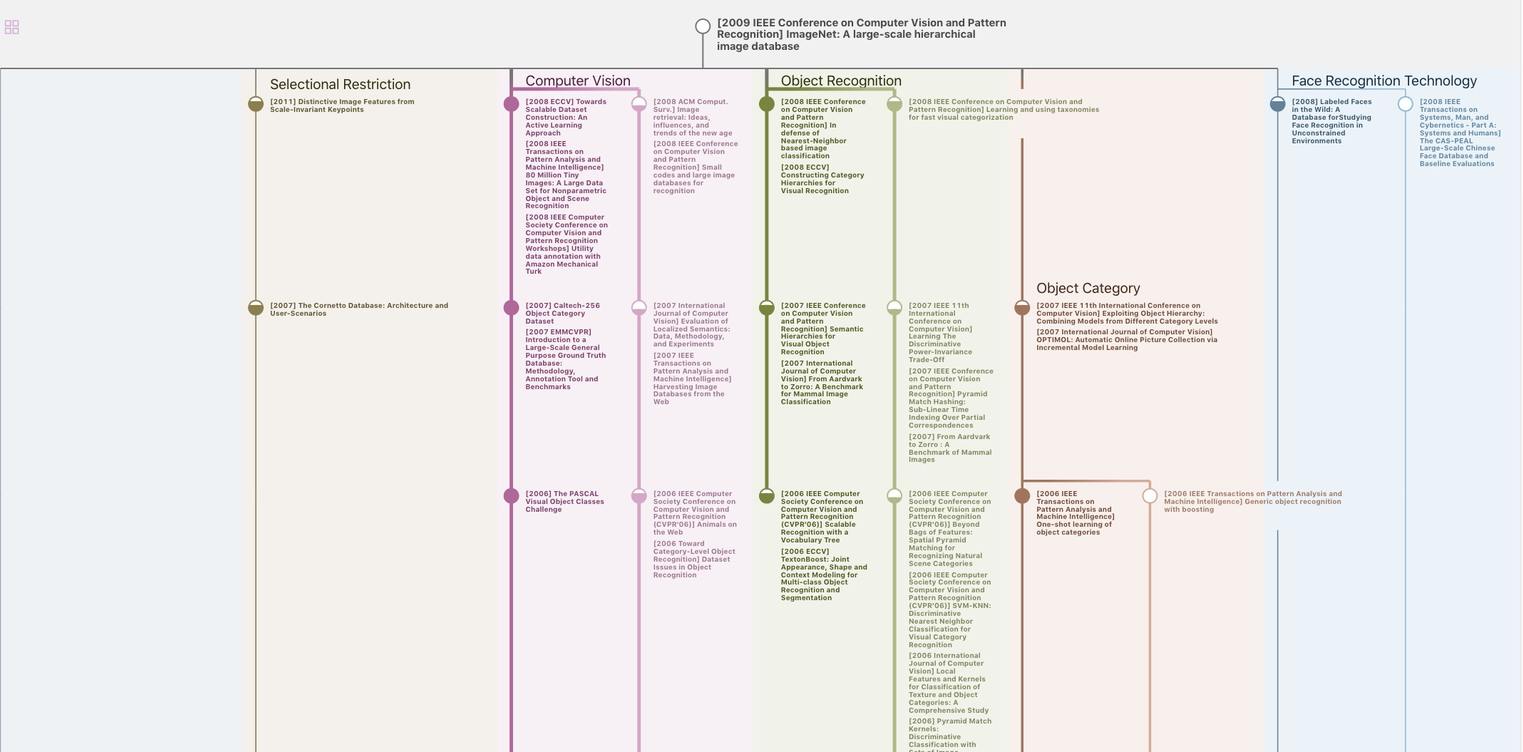
生成溯源树,研究论文发展脉络
Chat Paper
正在生成论文摘要