Energy consumption prediction and optimization of industrial robots based on LSTM
JOURNAL OF MANUFACTURING SYSTEMS(2023)
摘要
Due to wide distribution and low energy efficiency, the energy-saving of industrial robots draws more and more attention, and a large number of methods have emerged to predict or optimize the energy consumption (EC) of robots. However, many dynamic and electrical parameters are unavailable due to the commercial limitations of industrial robots, which constrains the application of those model-based methods. Therefore, this paper proposes a data-driven method for the prediction and optimization of robot EC. Initially, the cause-and-effect relationship between robot EC and joint motion variables, such as the joint position, velocity, and acceleration, is qualitatively analyzed based on the influence of the capacitive and inductive components in the drive system. And a deep neural network based on long short-term memory (LSTM) is proposed to reveal the nonlinear mapping between the industrial robot EC and the joint motion variables, which can predict EC without the parameters of the industrial robot. Based on the proposed neural network, the adaptive genetic algorithm is adopted to optimize the time-variant scaling function, which can optimize the scaled trajectory to reduce EC without hardware modification. To validate the accuracy and efficacy of the proposed method, experiments are conducted on a KUKA KR60-3 six degree-of-freedom (DOF) industrial robot. The results demonstrate that the proposed neural network can predict EC with a mean absolute percentage error less than 4.21% and the proposed method reduces the EC by 22.35%.
更多查看译文
关键词
Industrial robots,Data-driven,LSTM,Time scaling,Energy optimization
AI 理解论文
溯源树
样例
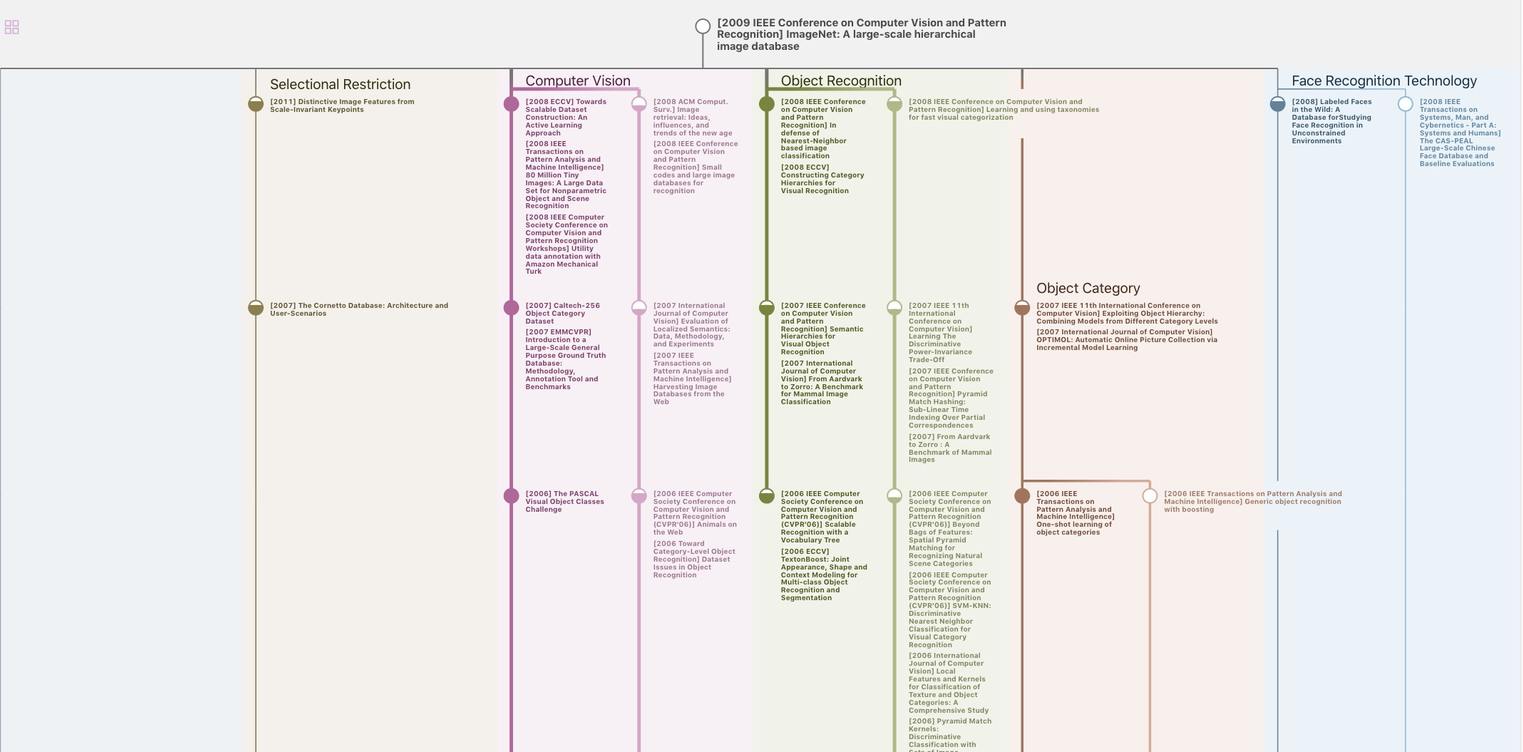
生成溯源树,研究论文发展脉络
Chat Paper
正在生成论文摘要