Transfer learning-based fault detection in wind turbine blades using radar plots and deep learning models
ENERGY SOURCES PART A-RECOVERY UTILIZATION AND ENVIRONMENTAL EFFECTS(2023)
摘要
Faults in wind turbine blades are considered a critical issue that can affect the safety and performance of wind turbines. The proposed research aimed to monitor wind turbine blades and identify fault conditions using a transfer learning approach. The study utilized one good and four faulty blade conditions: bend, hub-blade loose connection, erosion, and pitch angle twist. Vibration signals for each blade condition were collected and converted as radar plots that were fed and analyzed using pre-trained deep learning models including ResNet-50, AlexNet, VGG-16, and GoogleNet. Hyperparameters including optimizer, train-test split ratio, batch size, epochs, and learning rate were examined to determine the optimal configuration for each network. The study's core findings indicate that ResNet-50 outperformed all other models, achieving an impressive accuracy rate of 99.00%. The other models achieved lower accuracy rates, with AlexNet achieving 96.70%, GoogleNet achieving 97.00%, and VGG-16 achieving 95.00%. These findings highlight the potential of using deep learning models for wind turbine monitoring and fault detection, which could significantly improve the efficiency and reliability of wind turbines.
更多查看译文
关键词
Wind turbine blade, condition monitoring, deep learning, transfer learning, fault identification
AI 理解论文
溯源树
样例
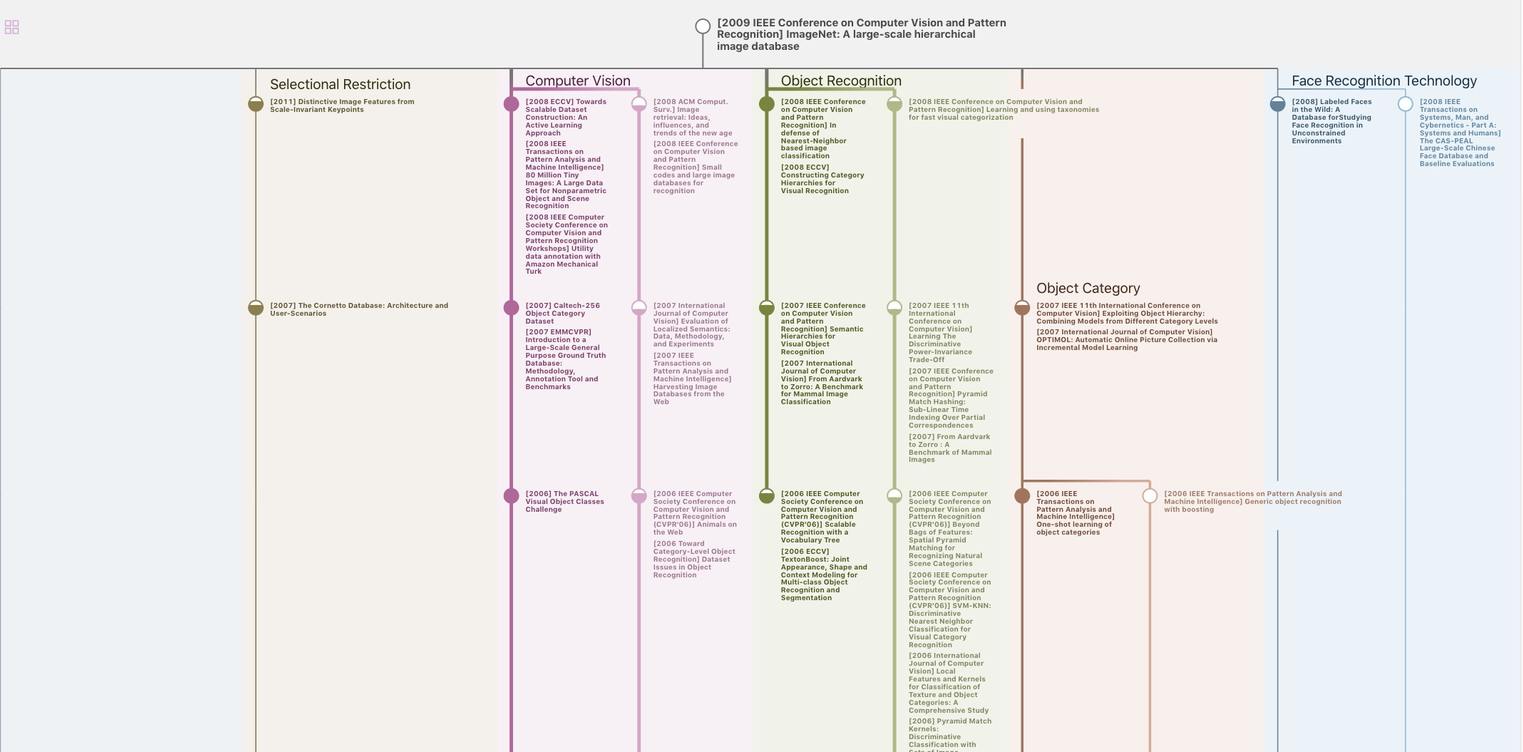
生成溯源树,研究论文发展脉络
Chat Paper
正在生成论文摘要