Effectiveness of deep learning in early-stage oral cancer detections and classification using histogram of oriented gradients
EXPERT SYSTEMS(2023)
摘要
Early detection of oral cancer (OC) improves survival prospects. Artificial intelligence (AI) is gaining popularity in diagnostic medicine. Oral cancer is a primary global health concern, accounting for 177,384 deaths in 2018; most cases occur in low- and middle-income countries. Automated disease identification in the oral cavity may be facilitated by the ability to identify both possibly and definite malignant lesions. This study aimed to examine the evidence currently available on the effectiveness of AI in diagnosing OC. They highlighted the ability of AI to analyse and identify the early stages of OC. Furthermore, radial basis function networks (RBFN) were employed to develop automated systems to generate intricate patterns for this challenging operation. The stochastic gradient descent algorithm (SGDA) selected the model parameters that best matched the predicted and observed results. It can be used. The initial data was collected for this study to evaluate. Two deep learning-based computer vision algorithms have been developed to recognize and categorize oral lesions, which is necessary for the early detection of oral cancer. Several examples of HoG include the Canny edge detector, SIFT (scale invariant and feature transform), and SIFT (scale invariant and feature transform). In computer vision and image processing, it is used to find objects. We investigated the potential uses of deep learning-based computer vision techniques in oral cancer and the viability of an automated system for OC recognition based on photographic images. That made calculations to determine the accuracy, sensitivity, specificity, and receiver operating characteristic curve areas across all validation datasets, including internal, external, and clinical validation (AUC). The RBFN-SDC model outperformed all others. For 1000 data points, the accuracy of the RBFN-SDC model is 99.99%, while the accuracy of the R-CNN, CNN, DCNN, and SVM models is 91.54%, 90.14%, 93.89%, and 94.87%, respectively.
更多查看译文
关键词
artificial intelligence (AI),deep learning,histogram of oriented gradients (HoG),oral cancer (OC),radial basis function networks (RBFN),stochastic gradient descent algorithm (SGDA)
AI 理解论文
溯源树
样例
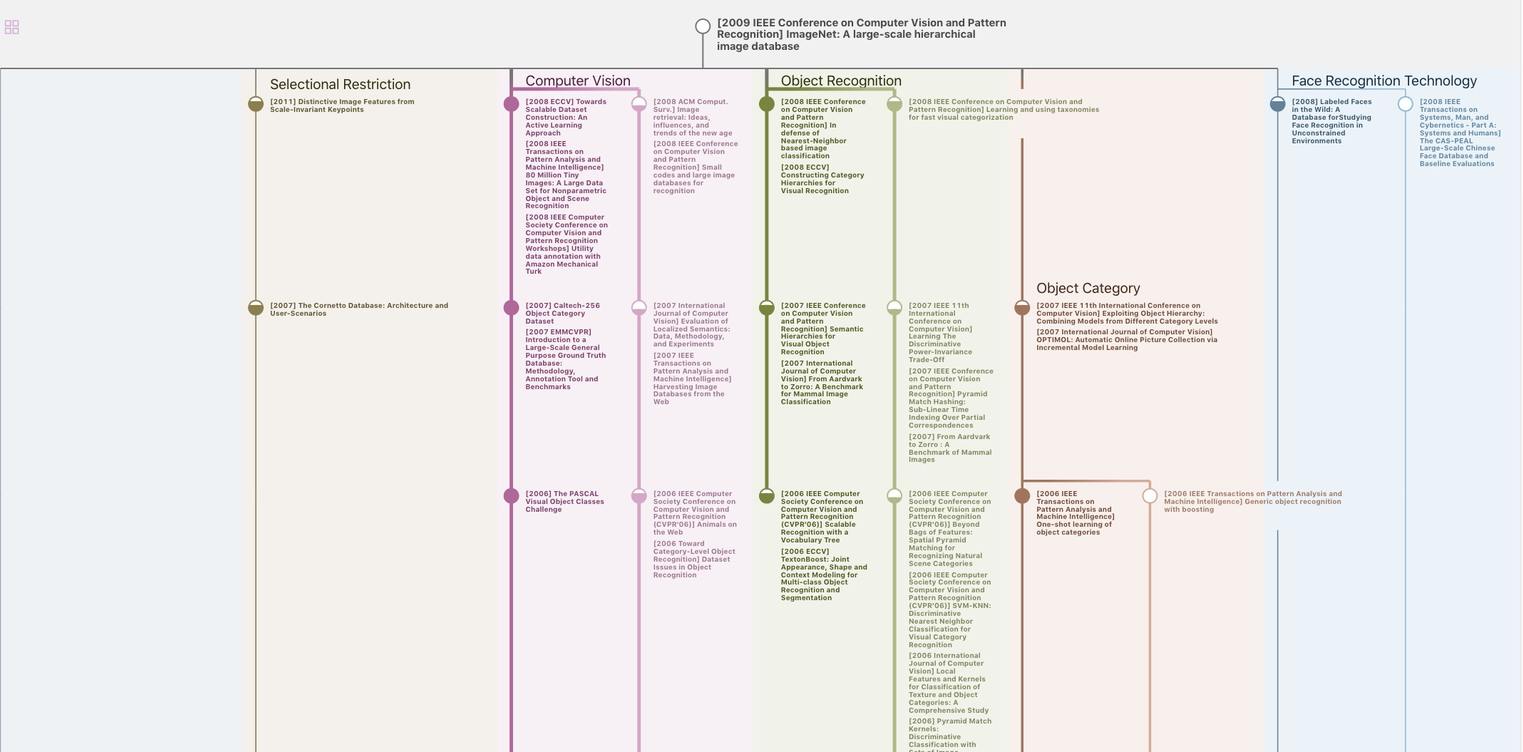
生成溯源树,研究论文发展脉络
Chat Paper
正在生成论文摘要