Total-variation regularized U-Net for wildfire burned area mapping based on Sentinel-1 C-Band SAR backscattering data
ISPRS JOURNAL OF PHOTOGRAMMETRY AND REMOTE SENSING(2023)
摘要
Previous studies have shown that Synthetic Aperture Radar (SAR) is able to detect burned areas, serving as a key data source for monitoring active wildfires in situations where optical sensors are hindered by dense smoke or cloud cover. Radar remote sensing provides rich and useful data on historical burned areas, which are critical for large-scale wildfire burned area mapping. This study aims to unveil the potential inherent in Sentinel-1 C-Band SAR data and to investigate the impact of reference masks on wildfire burned area mapping. SAR images frequently exhibit disruptive speckle noise, which manifests as isolated pixels or small regions, thereby hindering the seamless identification of burned areas through SAR data. To mitigate noise and enhance the connectivity of SAR-based burned area delineation, we propose a novel approach: a Total-Variation (TV) regularized U-Net model, tailored to learn from noisy pseudo masks derived from Sentinel-1 C-Band SAR backscattering data. To validate its efficacy, we assembled a dataset encompassing 16 geographically diverse wildfire events, incorporating SAR-based kMap, a SAR-based binary label (designated as SARREF), and an optical-based binary label (referred to as OptREF). The kMap, serving as a relative indicator of SAR backscattering change, is defined as the divergence between post-fire SAR backscattering and the temporal average, normalized by the temporal standard deviation of pre-fire SAR time series. Utilizing SAR-based kMap as input and SARREF or OptREF as labels, we systematically explored the impact of TV regularization on SAR-based burned area mapping. Our experimental findings highlight several key insights: 1) Vanilla U-Net considerably elevates the F1 score from 0.61 to 0.66 when compared to threshold-based approaches; 2) The TV -regularized U-Net exhibits a notable capacity for enhancing both accuracy and the connectivity of SAR-based burned areas. When trained with noisy SARREF, it achieves a substantial F1 score of 0.69 and an IoU score of 0.53. 3) In scenarios involving OptREF, the vanilla U-Net attains peak performance with the highest F1 (0.75) and IoU (0.61) scores, with the expected outcome of TV regularization yielding no additional enhancement.
更多查看译文
关键词
Wildfire,Burned area,SAR,Deep learning,Segmentation,U-Net,Total variation,Sentinel-1
AI 理解论文
溯源树
样例
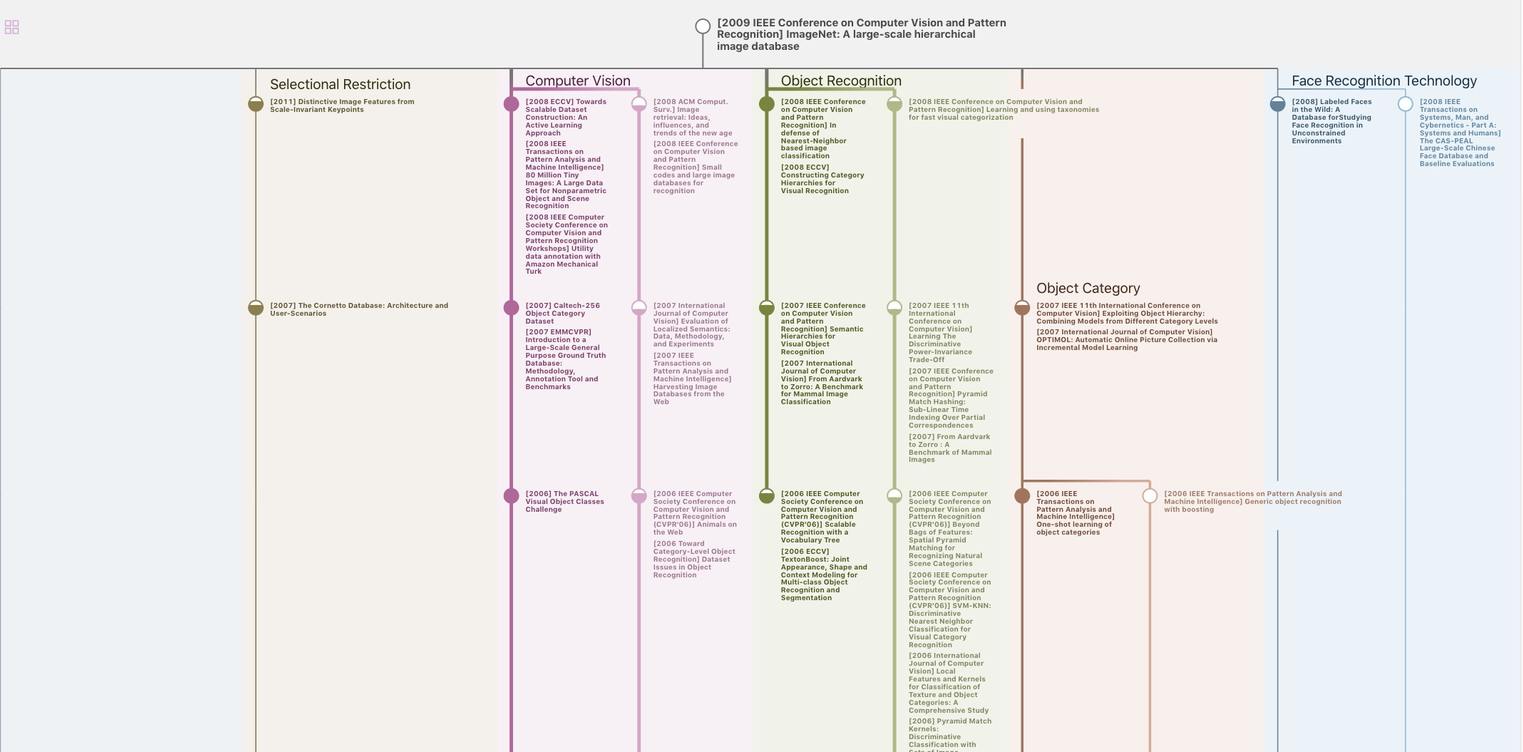
生成溯源树,研究论文发展脉络
Chat Paper
正在生成论文摘要