Using Deep Learning for Flexible and Scalable Earthquake Forecasting
GEOPHYSICAL RESEARCH LETTERS(2023)
摘要
Seismology is witnessing explosive growth in the diversity and scale of earthquake catalogs. A key motivation for this community effort is that more data should translate into better earthquake forecasts. Such improvements are yet to be seen. Here, we introduce the Recurrent Earthquake foreCAST (RECAST), a deep‐learning model based on recent developments in neural temporal point processes. The model enables access to a greater volume and diversity of earthquake observations, overcoming the theoretical and computational limitations of traditional approaches. We benchmark against a temporal Epidemic Type Aftershock Sequence model. Tests on synthetic data suggest that with a modest‐sized data set, RECAST accurately models earthquake‐like point processes directly from cataloged data. Tests on earthquake catalogs in Southern California indicate improved fit and forecast accuracy compared to our benchmark when the training set is sufficiently long (>10 4 events). The basic components in RECAST add flexibility and scalability for earthquake forecasting without sacrificing performance.
更多查看译文
关键词
earthquake,forecasting,machine learning,RECAST,ETAS,seismology
AI 理解论文
溯源树
样例
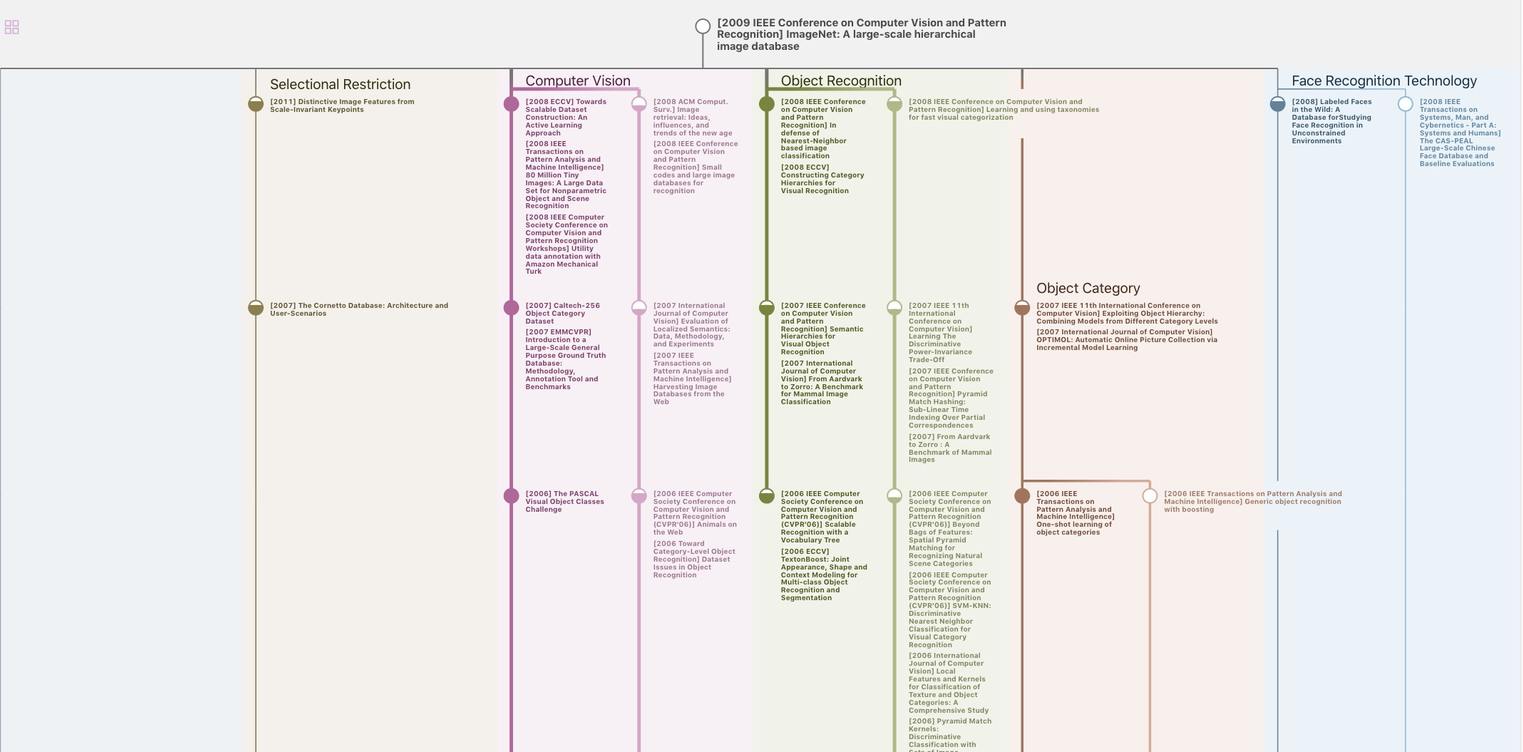
生成溯源树,研究论文发展脉络
Chat Paper
正在生成论文摘要