TableStrRec: framework for table structure recognition in data sheet images
INTERNATIONAL JOURNAL ON DOCUMENT ANALYSIS AND RECOGNITION(2023)
摘要
Billions of documents in data sheet format are shared between various organizations across the globe on a daily basis. The essential information in these documents is presented in tabular format. Extracting and assimilating this information can help organizations make data-driven decisions. Solutions for detecting tables in document images have been well explored. Thus, in this work, we propose TableStrRec, a deep learning-based approach to recognize the structure of such detected tables by detecting rows and columns. TableStrRec comprises two Cascade R-CNN architectures, each with a deformable backbone and Complete IOU loss to improve their detection performance. One architecture detects and classifies rows as regular rows (rows without a merged cell) and irregular rows (groups of regular rows that share a merged cell). The second architecture detects and classifies columns as regular columns (columns without a merged cell) and irregular columns (groups of regular columns that share a merged cell). Both architectures work in parallel to provide the results in a single inference. We show that utilizing TableStrRec to detect four classes of objects improves the table structure recognition performance on three public test sets. We achieve 90.5% and 89.6% weighted average F1 scores on the ICDAR2013 test set for rows and columns, respectively. On the TabStructDB test set, we achieve 72.7% and 78.5% weighted average F1 score for rows and columns, respectively. We also evaluate the proposed method under the FinTabNet dataset using the structure-only TEDS score, achieving 98.34
更多查看译文
关键词
Deep learning, Deformable convolutional neural networks, Image processing, Document analysis, Table structure recognition, Row and column detection, Page object detection
AI 理解论文
溯源树
样例
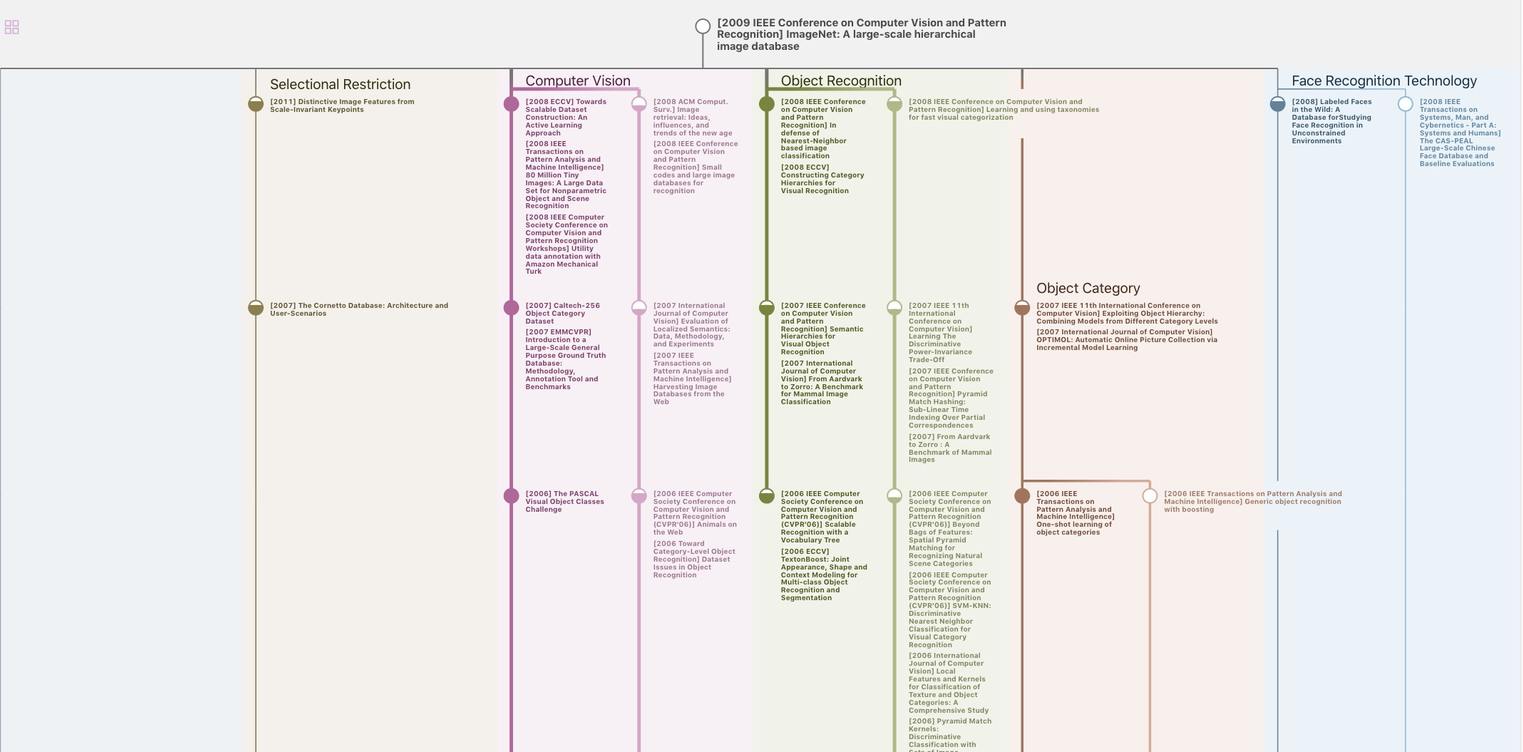
生成溯源树,研究论文发展脉络
Chat Paper
正在生成论文摘要