Low-rank Tensor Restoration for ERP extraction
BIOMEDICAL SIGNAL PROCESSING AND CONTROL(2024)
摘要
Event-related potential (ERP) data is essentially multi-dimensional, with correlated data in some spaces. Therefore, matrix and vector analysis results in structural information loss. Tensor decomposition can be used to explore the shared structural information of ERP signal among related conditions. Perceiving the decaying trends of the singular value changes of unfolding matrices indicate that they are low-rank matrices. Based on this assumption, in this work, a low-rank tensor restoration (LTR) method is proposed. An operator splitting method known as the alternating direction method of multipliers (ADMM) is adapted to tackle the proposed optimization problem with orthogonality and sparsity constraints. Accordingly, the problem is solved in a sequential fashion by computing the unconstrained and orthogonality constrained quadratic sub-problems with closed-form solutions. The algorithm is examined under three application areas, namely, noise removal, feature extraction and subject-to-subject transfer learning. The empirical evaluations on real P300-based ERP dataset demonstrate the robustness and effectiveness of the proposed method.
更多查看译文
关键词
Event related potentials,Low-rank tensor decomposition,ADMM,Multi-mode analysis
AI 理解论文
溯源树
样例
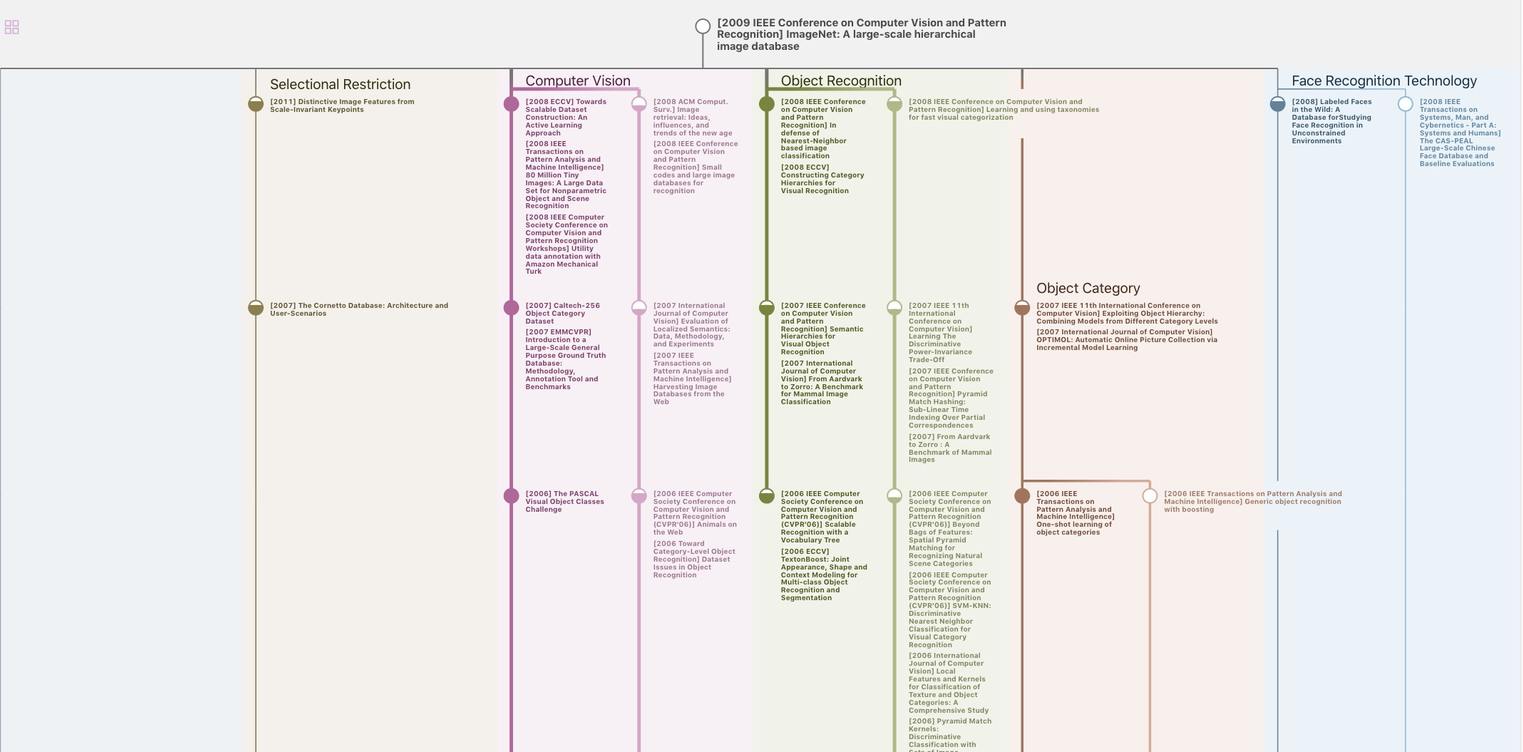
生成溯源树,研究论文发展脉络
Chat Paper
正在生成论文摘要