Tribological performance prediction of WS2 coating under different conditions by machine learning
WEAR(2023)
摘要
Tungsten disulfide (WS2) has great potential as solid lubricating coating on the metal surfaces due to its excellent wear resistance. In this study, a combination of experiments and machine learning (ML) was used to explore the friction process and predict the friction coefficient and wear rate of WS2 coatings. Copper coated with WS2 was prepared by spraying, and the tribological properties of the coating were studied under different loads, velocities, and environment temperatures using both experiments and simulations. Three ML algorithms were employed to investigate the correlations between multiple input parameters and output results. The results showed that the COF and wear rate increased with increasing load, velocity, and environment temperature, and that plastic deformation and adhesive wear were the dominant wear mechanisms under conditions of heavy load, high speed, and high temperature. The Extra Tree (ET) model effectively predicted the tribological properties of the WS2 coating, with a COF accuracy of 0.899, while the Gradient Boosting Regression Tree (GBRT) model achieved excellent accuracy in predicting wear rate, with an accuracy of 0.948. Feature importance analysis indicated that load had the greatest effect on both COF and wear rate.
更多查看译文
关键词
WS2,COF,Wear rate,Machine learning
AI 理解论文
溯源树
样例
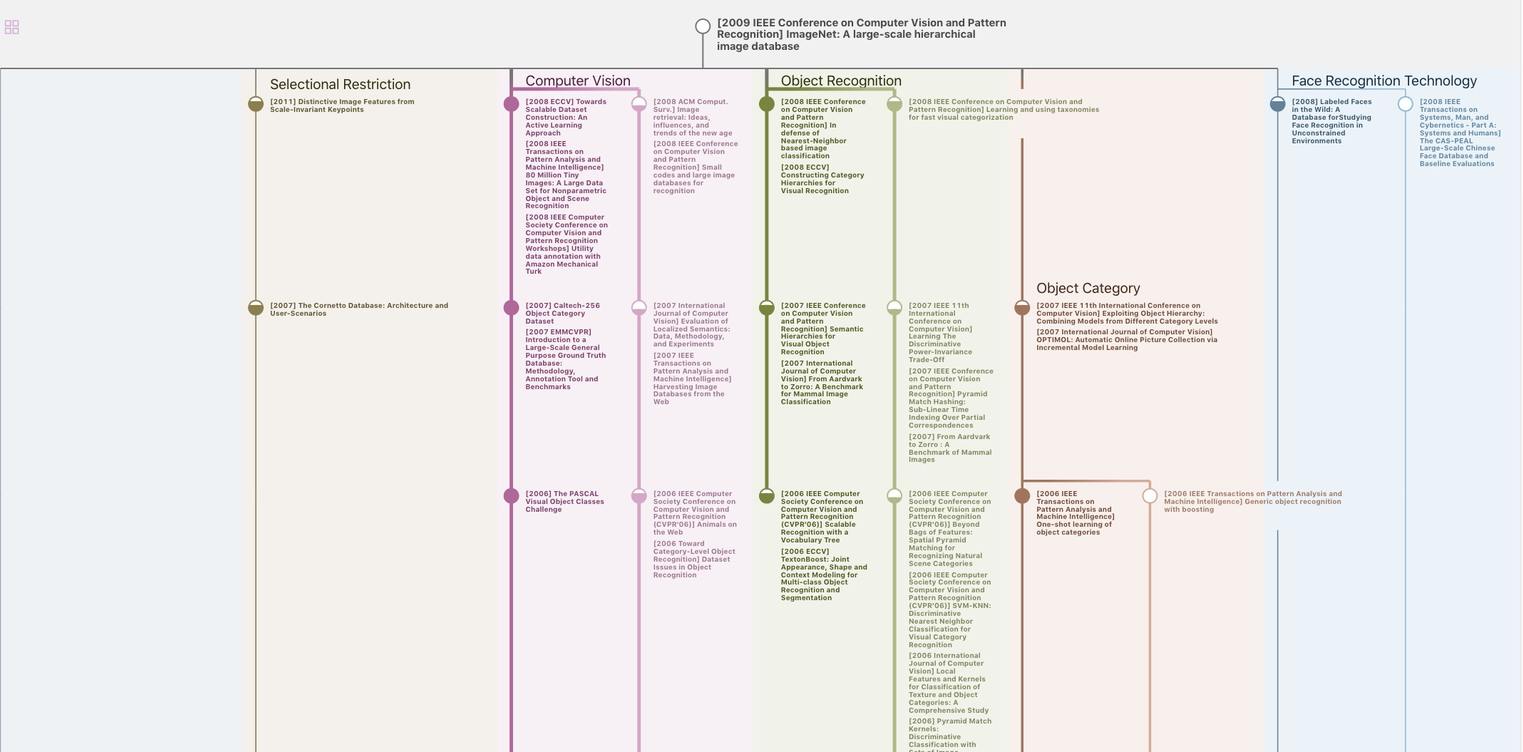
生成溯源树,研究论文发展脉络
Chat Paper
正在生成论文摘要