SFS feature selection with decision tree classifier for massive open online courses (MOOCs) recommendation
JOURNAL OF COMPUTERS IN EDUCATION(2023)
摘要
Online courses have recently gained popularity among millions of students worldwide, primarily due to their accessibility, affordability, and flexibility in accessing high-quality educational content from renowned institutions and universities. Nevertheless, MOOC courses' diversity and standard adjustments make it harder for learners to distinguish the best ones that match their profile and needs. In this context, we developed a Machine Learning model that uses learners' profile information and their interaction history with MOOC platforms to predict and recommend relevant MOOCs. One of the large-scale datasets is used to perform this work, which contains 16 courses and 16 features. Data preprocessing is conducted to clean, scale, and prepare the data for training to avoid having suboptimal results, along with an undersampling to adjust the distribution of samples in classes to prevent poor generalization and bias models. In fact, dealing with a large set of attributes often needs to be diminished to increase the model's performance and reduce the risk of noise and the model's overfitting. In this way, we applied the Sequential Forward Selection (SFS) to select the appropriate features for the target classes. The resulting subset of features was then used to train the model using the Decision Tree classifier. As a result, this combination provided noticeable results compared to existing works, with an accuracy of 82.8%. The proposed model can serve as a reliable tool for assisting learners in online education by providing personalized recommendations for MOOCs based on their individual profiles.
更多查看译文
关键词
MOOCS,Online Learning,Recommender systems,Machine Learning,Feature selection
AI 理解论文
溯源树
样例
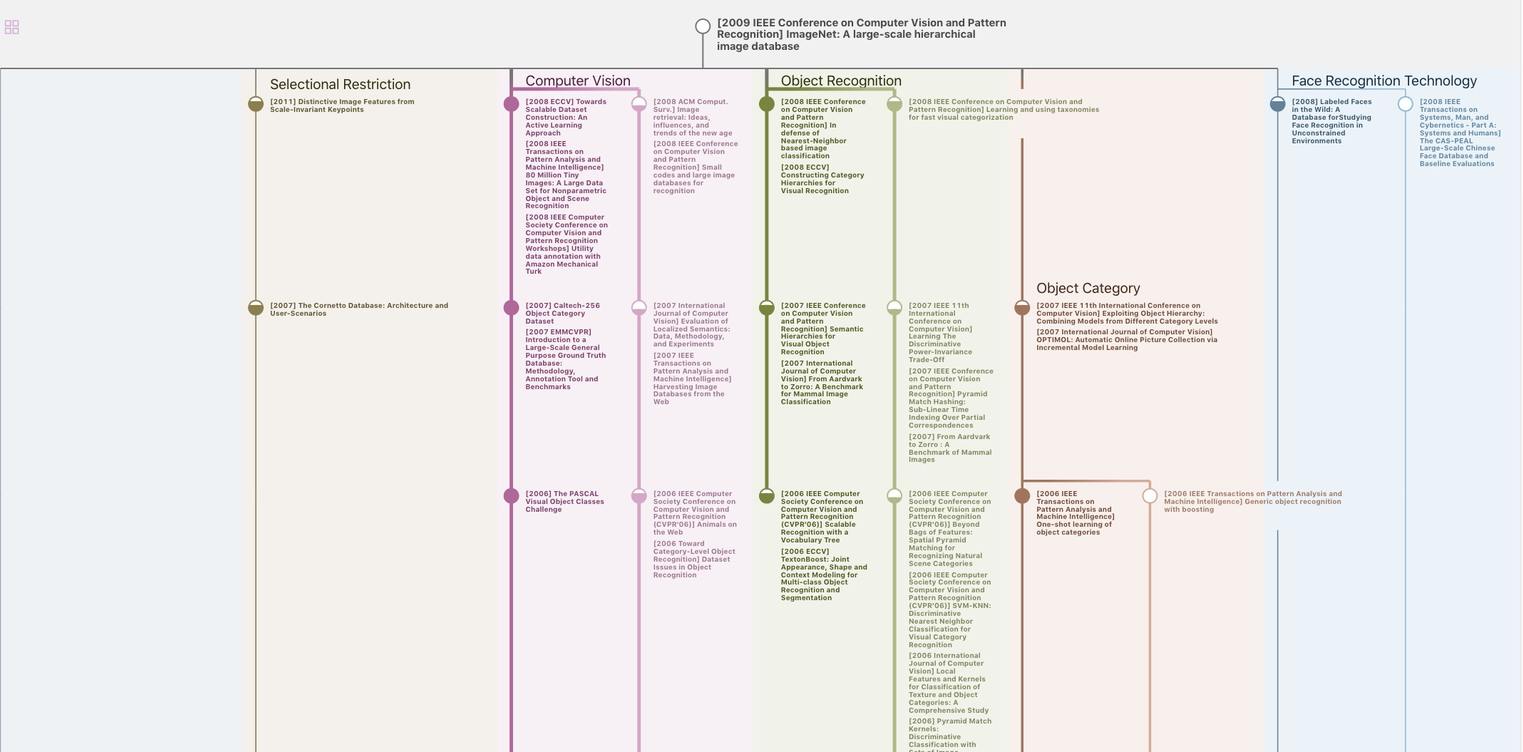
生成溯源树,研究论文发展脉络
Chat Paper
正在生成论文摘要