Focal Distillation From High-Resolution Data to Low-Resolution Data for 3D Object Detection
IEEE TRANSACTIONS ON INTELLIGENT TRANSPORTATION SYSTEMS(2023)
摘要
LiDAR-based 3D object detection plays an essential role in autonomous driving. Although the detector trained on high-resolution data has much better performance than the same detector trained on low-resolution data, the high-resolution LiDAR cannot be widely used due to its high price. In this work, we propose a new distillation method called Focal Distillation to bridge the gap between high-resolution detector (teacher model) and low-resolution detector (student model). It consists of three essential components: focal classification distillation (FCD), focal regression distillation (FRD) and focal feature distillation (FFD). Taking the low-resolution data as input, the student model can learn discriminative features and produce more accurate results with the assistance of the teacher model trained on high-resolution data. We conducted extensive experiments to validate the effectiveness of Focal Distillation. Evaluated on the KITTI validation set, a typical SECOND model trained with Focal Distillation outperformed its non-distilled counterpart by 3.37%, 7.52%, 11.35% mAP on the category Car, Pedestrian, and Cyclist of moderate level, respectively. Moreover, the remarkable improvements observed on different models and different datasets further demonstrate the generalization ability of our proposed method.
更多查看译文
关键词
3D object detection,knowledge distillation,3D point cloud analysis
AI 理解论文
溯源树
样例
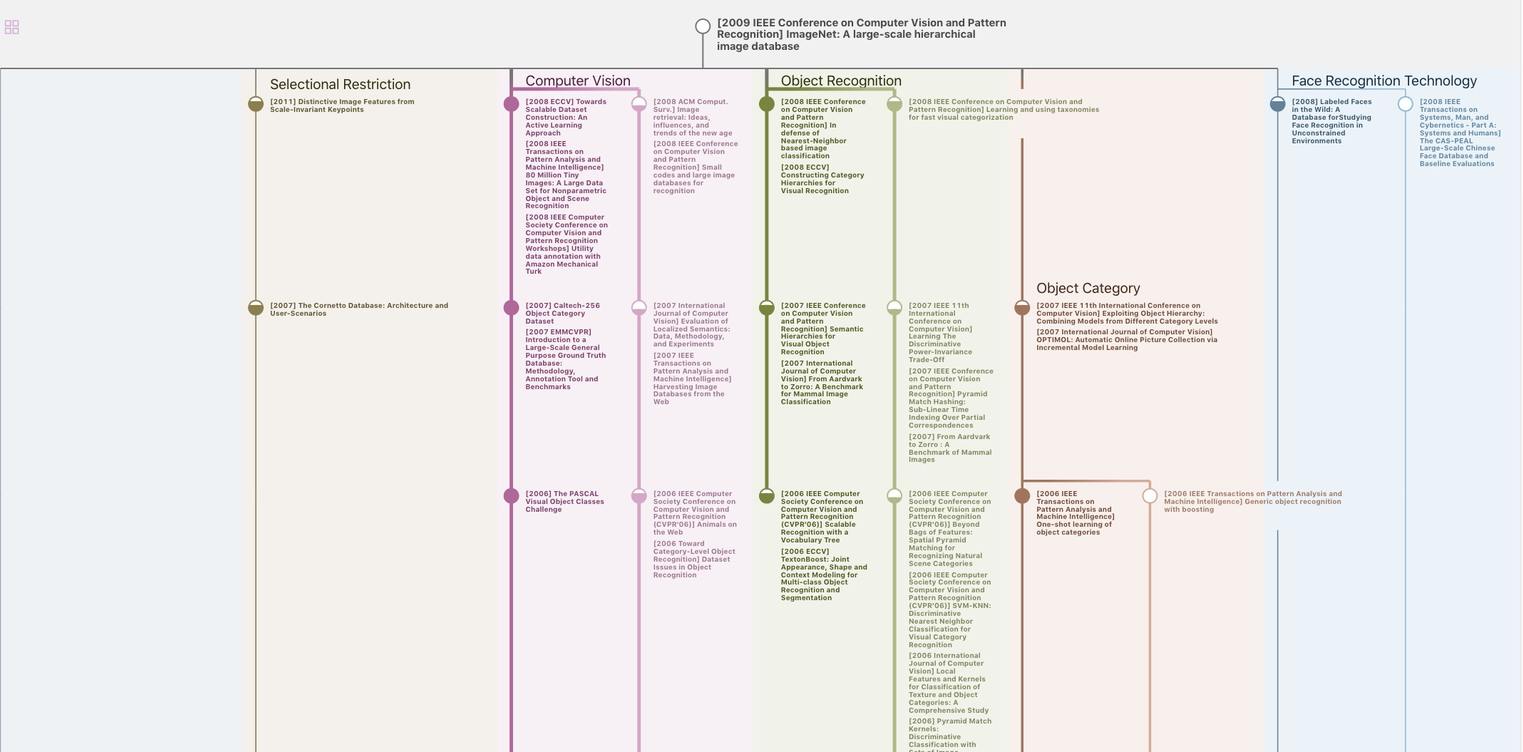
生成溯源树,研究论文发展脉络
Chat Paper
正在生成论文摘要