Boundary-adaptive Kernel Density Estimation: the Case of (near) Uniform Density
JOURNAL OF NONPARAMETRIC STATISTICS(2024)
摘要
We consider nonparametric kernel estimation of density functions in the bounded-support setting having known support [a, b] using a boundary-adaptive kernel function and data-driven bandwidth selection, where a and b are finite and known prior to estimation. We observe, theoretically and in finite sample settings, that when bounds are known a priori this kernel approach is capable of out-performing even correctly specified parametric models, in the case of the uniform distribution. We demonstrate that this result has implications for modelling a range of densities other than the uniform case. Furthermore, when bounds [a, b] are unknown and the empirical support (i.e. [min(xi), max(xi)]) is used in their place, similar behaviour surfaces.
更多查看译文
关键词
Nonparametric,density,boundary,smoothing
AI 理解论文
溯源树
样例
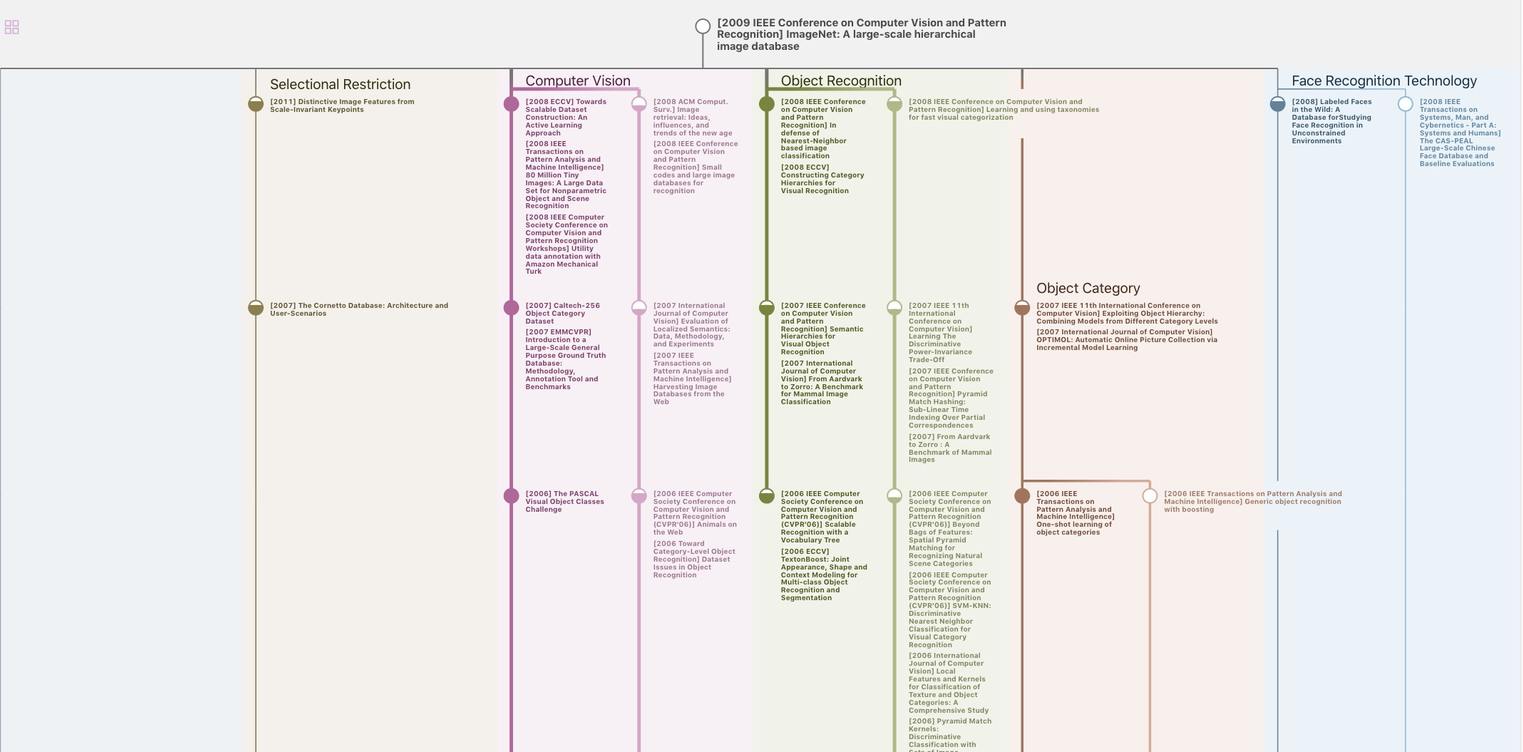
生成溯源树,研究论文发展脉络
Chat Paper
正在生成论文摘要