A separable training algorithm based on nonmonotone trust-region method for Neural ODE
2023 IEEE 12TH DATA DRIVEN CONTROL AND LEARNING SYSTEMS CONFERENCE, DDCLS(2023)
摘要
Neural ordinary differential equations (Neural ODE) interprets deep networks as discretization of dynamical systems, and has shown great promise in the physical science, modeling irregular time series, and mean field games. The Neural ODE comsumes a long time training process, which is arguably one of the main stumbling blocks towards their widespread adoption. To improve the convergence speed of training, in this parper, we formulate the training task as a separable nonlinear optimization problem, and propose a separable training algorithm based on a nonmonotone trust-region method. The proposed algorithm uses the variable projection strategy to reduce the dimension of variables by solving a subproblem and then the trust-region method is used to optimize the reduced function. To accelerate the convergence speed, we introduce the nonmonotone strategy to make the update of trust-region radius elastic and employ the adaptive technology that uses the gradient information of the objective function to update the radius. Numerical results confirm the effectiveness of the proposed algorithm.
更多查看译文
关键词
Neural ODE,nonmonotone trust-region,adaptive radius,variable projection
AI 理解论文
溯源树
样例
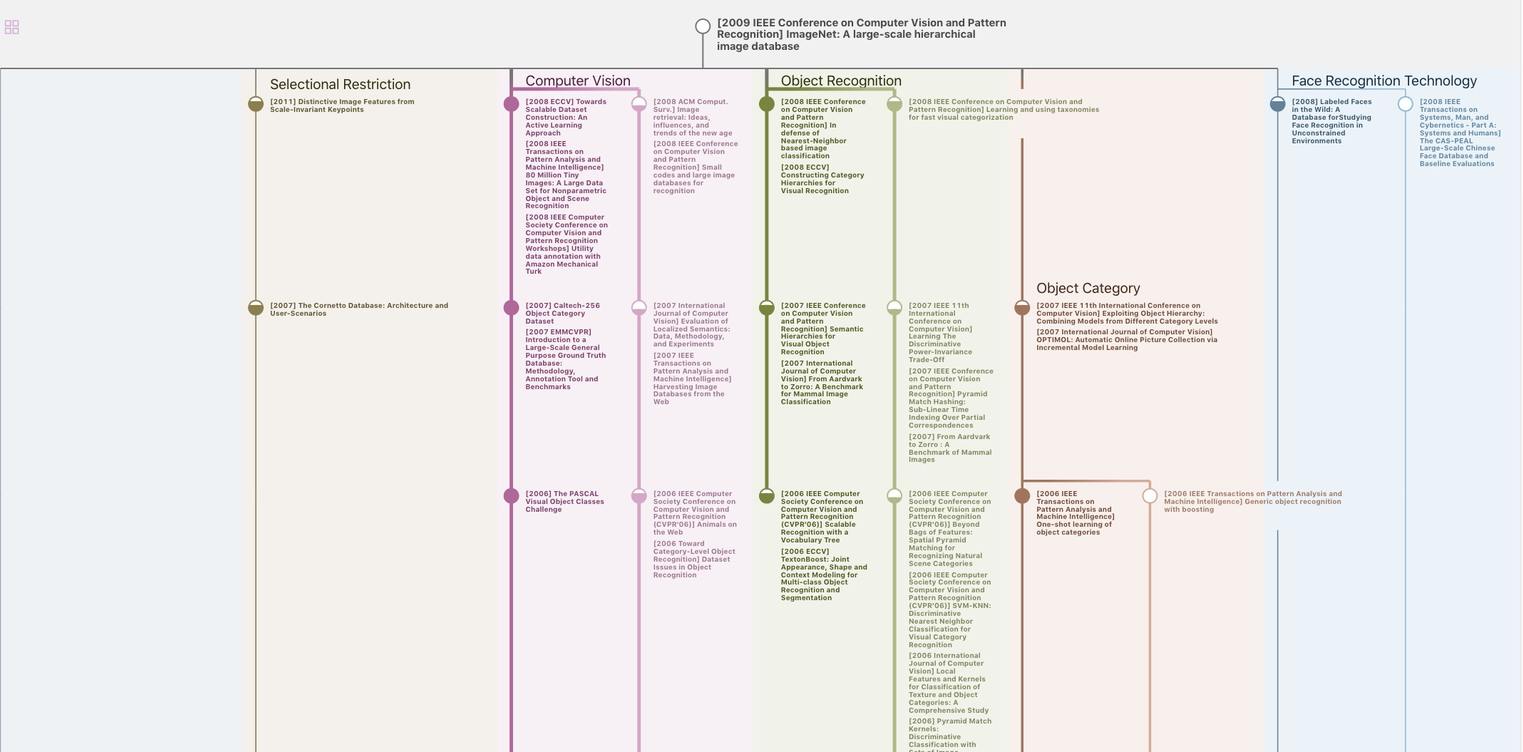
生成溯源树,研究论文发展脉络
Chat Paper
正在生成论文摘要