CGS-Net:Classification-guided Segmentation Network for Improved Gland Segmentation
2023 IEEE 12TH DATA DRIVEN CONTROL AND LEARNING SYSTEMS CONFERENCE, DDCLS(2023)
摘要
The diagnosis of colorectal cancer depends on the analysis of pathological images, and it is of great significance to accurately segment the shape of glands in pathological images. Accurate gland segmentation is extremely challenging, such as adhesion between adjacent glands, huge morphological differences between benign and malignant glands and so on. This paper proposes a classification-guided segmentation network (CGS-NET), which uses the characteristics related to benign and malignant glands after classification to improve the segmentation accuracy of glands. A local feature attention module and a multi-feature fusion module are introduced to enhance the encoder features and fuse the outputs of different scales, respectively. Experiments show that the proposed method can segment different types of glands well, and its performance on the 2015 MICCAI Gland Challenge is better than some existing methods compared in this paper.
更多查看译文
关键词
Gland image Segmentation,Classification-guided Segmentation,Attention,Feature Fusion
AI 理解论文
溯源树
样例
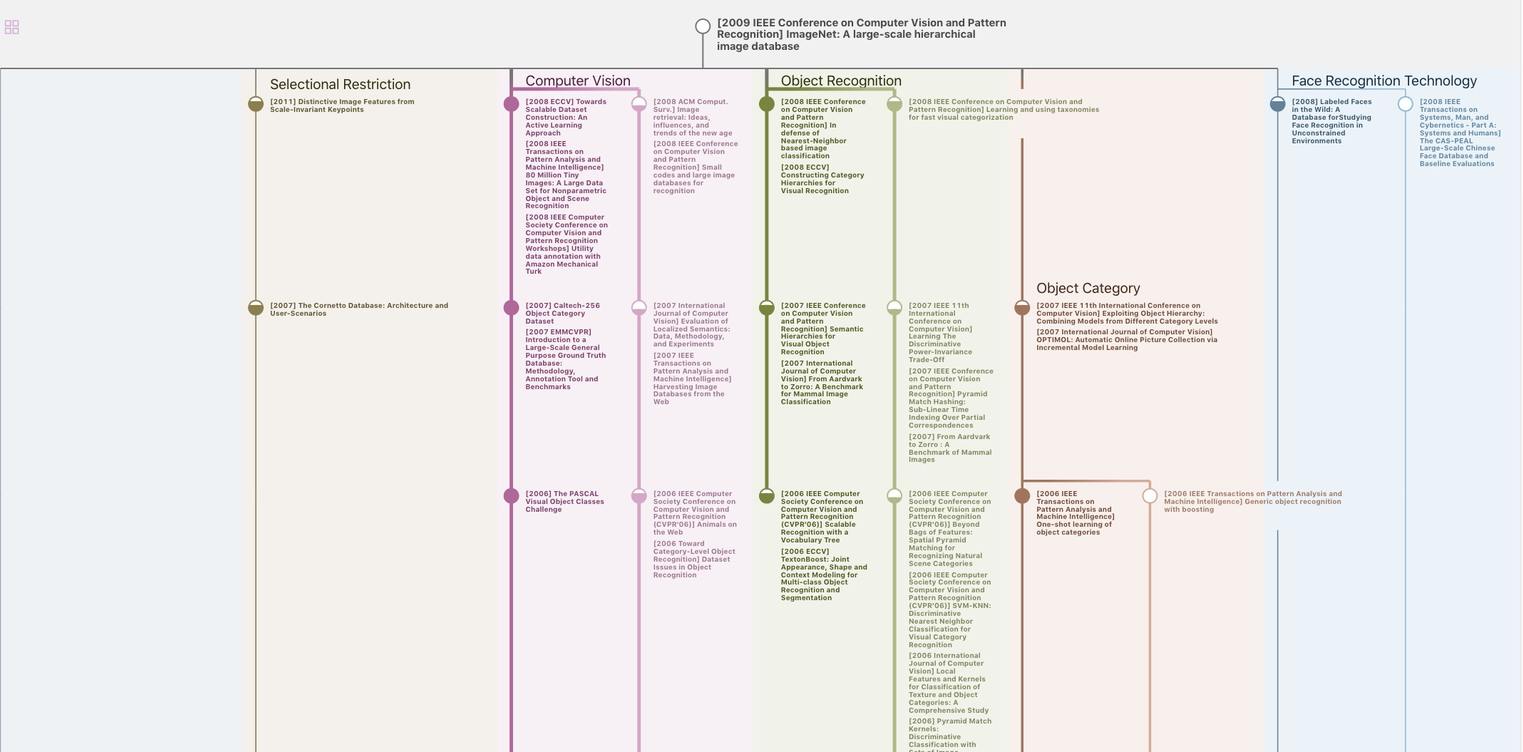
生成溯源树,研究论文发展脉络
Chat Paper
正在生成论文摘要