Interpretable Diagnosis of Glaucoma Based on Attention Mechanism and Embedded Class Activation Map
2023 IEEE 12TH DATA DRIVEN CONTROL AND LEARNING SYSTEMS CONFERENCE, DDCLS(2023)
摘要
Glaucoma is a group of heterogeneous neurodegenerative diseases, which has become the main cause of irreversible blindness worldwide, so the early screening of glaucoma has become crucial. Although the methods of visualizing the feature maps of convolutional layers increase the transparency of deep learning models to a certain extent, these methods often embed high-level connectivity constraints, which leads to the fact that the interpretable diagnosis of glaucoma can only obtain interpretation results from high-dimensional information. The proposed GAMNet based on attention mechanism and embedded class activation map not only solve the problem that the feature information to be concerned under multi-scale cannot be accurately focused by attention, but also release the constraints of high convolutional layer features on the interpretation results of the model from the perspective of fusion features. Firstly, a dynamic receptive field module is introduced on the basis of the multi-scale feature pyramid structure, and the embedded coordinate attention mechanism is used to effectively capture long-distance dependencies, so as to construct a semantically rich dynamic multi-scale feature pyramid for accurate and efficient diagnosis of glaucoma fundus images. Secondly, the hierarchical gradient function of gradient-weighted class activation map is established, and the class activation map after feature fusion is used to provide a more detailed attention map, which provides a decision-making basis for glaucoma diagnosis. GAMNet provides the evidence activation map, compared with Garbor, Wavelet, GRI and Superpixel in the performance of optic disc segmentation, the results show that GAMNet classification is accurate, and provides the evidence activation map. It not only shows diagnostic results, but also has good interpretation performance without high-level connectivity constraints.
更多查看译文
关键词
Glaucoma,Coordinate attention mechanism,Interpretability diagnosis,Class activation map
AI 理解论文
溯源树
样例
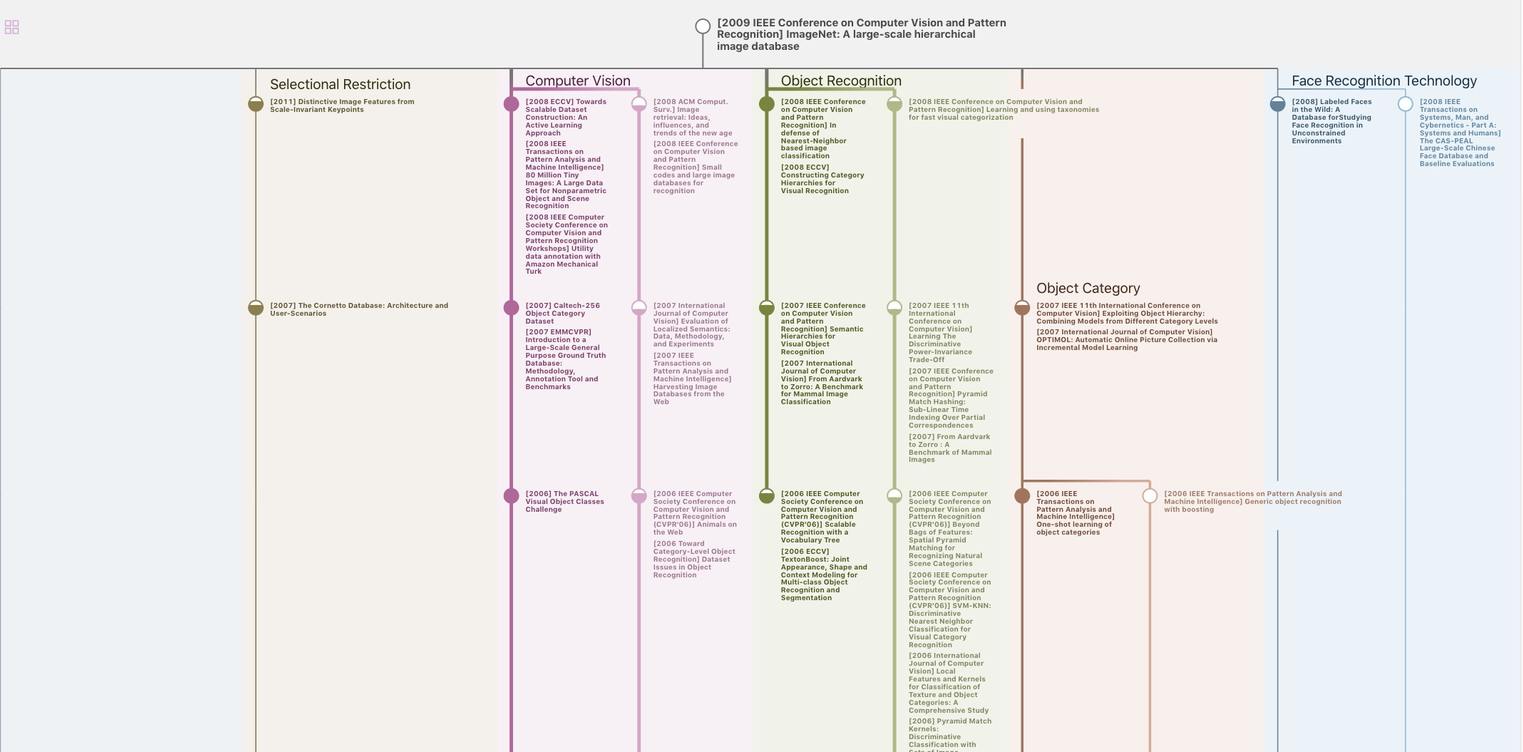
生成溯源树,研究论文发展脉络
Chat Paper
正在生成论文摘要