An Intra-Class Ranking Metric for Remote Sensing Image Retrieval
Remote sensing(2023)
摘要
With the rapid development of internet technology in recent years, the available remote sensing image data have also been growing rapidly, which has led to an increased demand for remote sensing image retrieval. Remote sensing images contain rich visual and semantic features, and have high variability and complexity. Therefore, remote sensing image retrieval needs to fully utilize the information in the images to perform feature extraction and matching. Metric learning has been widely used in image retrieval as it can train embedding spaces with high discriminability. However, existing deep metric learning methods learn embedding spaces with high discriminability by maximizing the differences between classes, while ignoring inherent intra-class differences during the learning process. In this paper, we design a new sample generation mechanism to generate samples from positive samples that meet the boundary constraints, thus obtaining quantifiable intra-class differences from real positive samples. Based on the sample generation relationship, we use a self-supervised approach to design an intra-class ranking loss function, which improves the discriminability of the generated embedding space for samples of the same class and maintains their ranking relationship in the embedding space. Moreover, this loss function can be easily combined with existing deep metric learning methods. Our aim is to help the network to better extract features and further improve the performance of remote sensing image retrieval through the sample generation mechanism and intra-class ranking loss. Finally, we conduct extensive experiments on multiple remote-sensing image datasets using multiple evaluation metrics such as mAP@K, which demonstrate that using the sample-generated intra-class ranking loss function can effectively improve the performance of remote sensing image retrieval.
更多查看译文
关键词
deep metric learning,loss function,image retrieval,self-supervised learning,sample generation
AI 理解论文
溯源树
样例
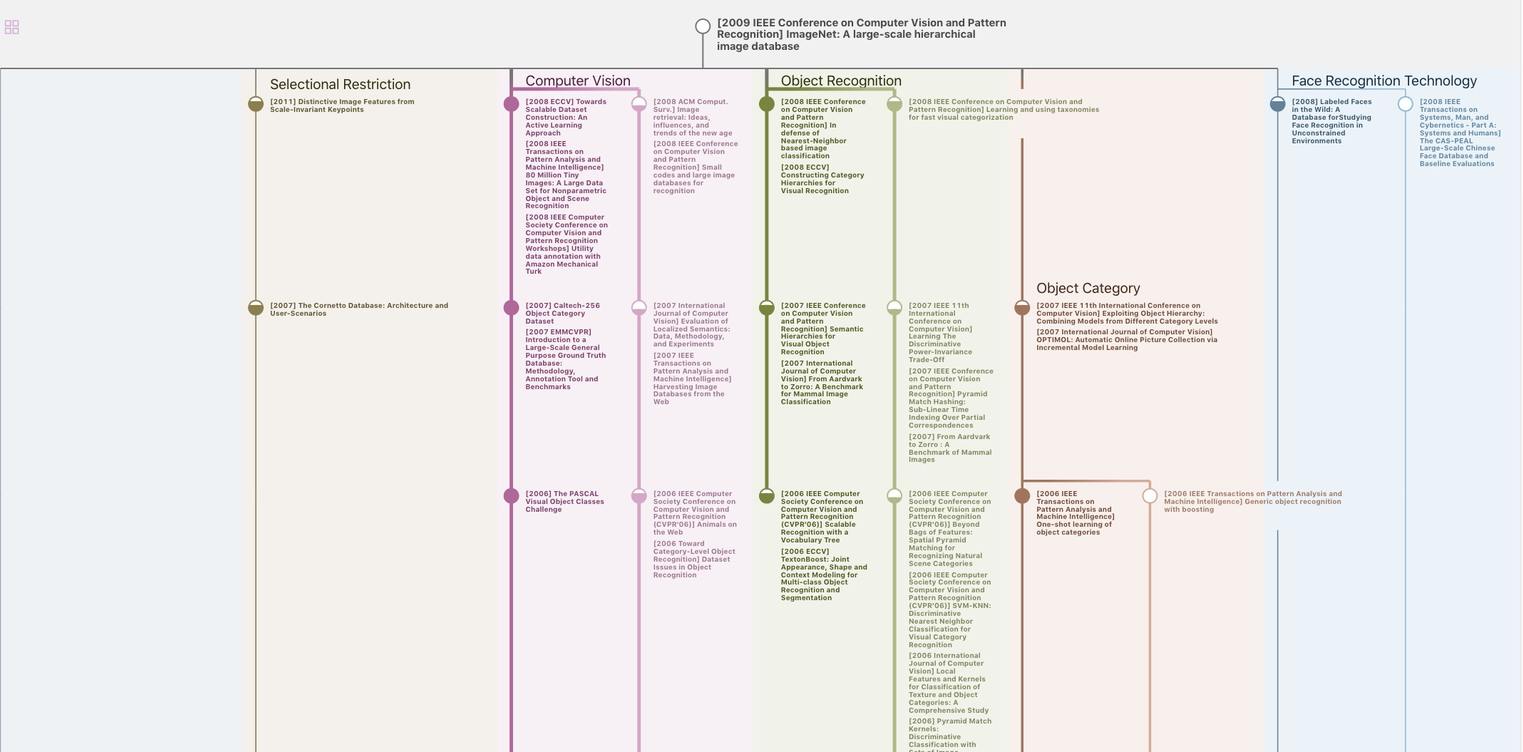
生成溯源树,研究论文发展脉络
Chat Paper
正在生成论文摘要