Active Learning to Minimize the Risk from Future Epidemics
2023 IEEE CONFERENCE ON ARTIFICIAL INTELLIGENCE, CAI(2023)
摘要
For any future epidemics (e.g., Covid-19), typical deep learning (DL) models are of no use as they require a large amount of data for training. Moreover, data collection (with annotations) typically takes months (and even years) in public healthcare. In such a context, active learning (or human/expert-in-the-loop) is the must, where a machine can learn from the first day with the minimum possible labeled data. In unsupervised learning, we propose to build pre-trained DL models that iteratively learn independently over time, where human/expert intervenes/mentors only when it makes mistakes and for limited data. To validate such a concept, deep features are used to classify data into two clusters (0/1: Covid-19/non-Covid-19) on two different image datasets: Chest X-ray (CXR) and Computed Tomography (CT) scan of sizes 4,714 and 10,000 CTs, respectively. Using pre-trained DL models and unsupervised learning, in our active learning framework, we received the highest AUC of 0.99 and 0.94 on CXR and CT scan datasets, respectively. Our results are comparable with the fully trained (on large data) state-of-the-art DL models.
更多查看译文
关键词
Active learning,future epidemics,Covid-19,CT scans,Chest X-rays
AI 理解论文
溯源树
样例
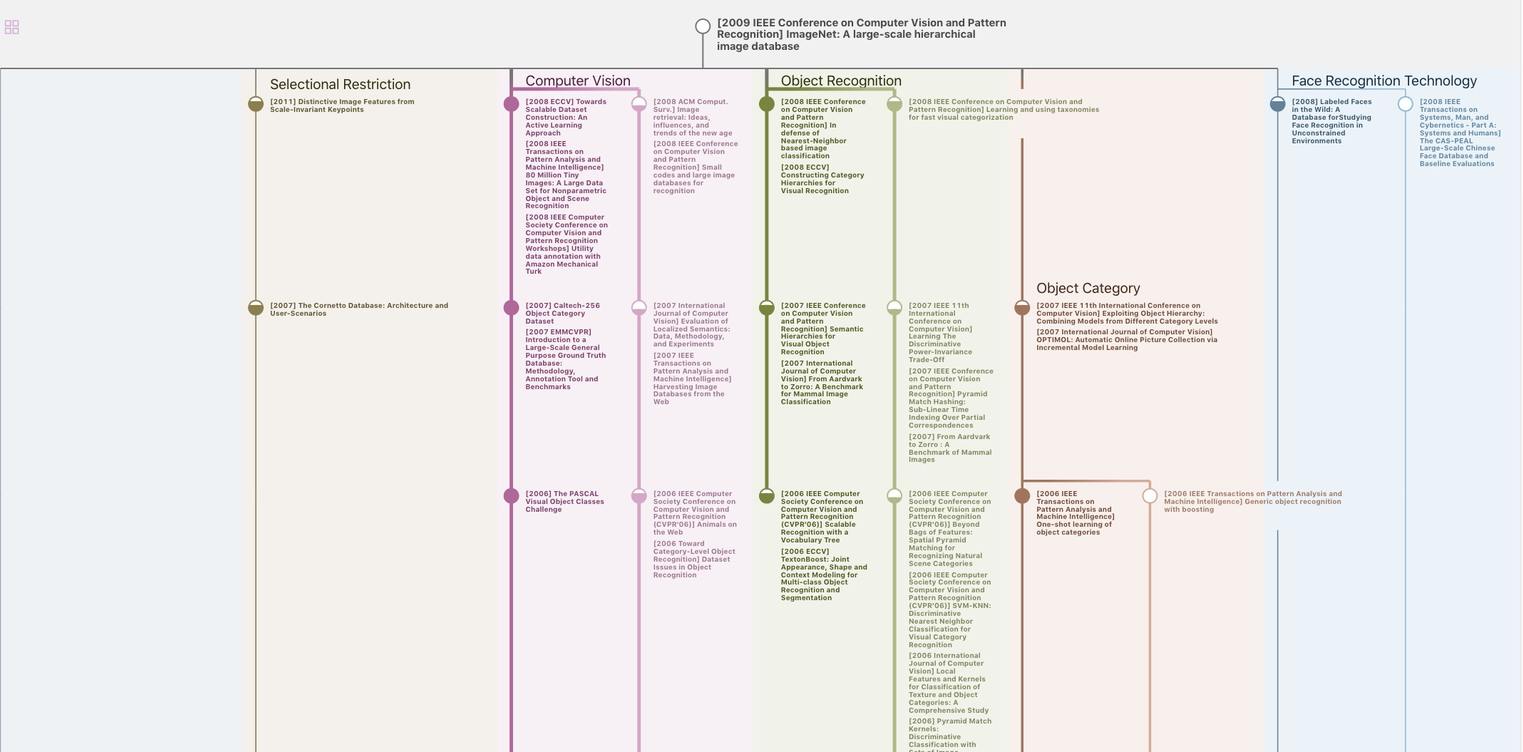
生成溯源树,研究论文发展脉络
Chat Paper
正在生成论文摘要