Tackling the Imbalance Biases in the Code Cloze Test
2023 IEEE 12TH DATA DRIVEN CONTROL AND LEARNING SYSTEMS CONFERENCE, DDCLS(2023)
摘要
Code automation aims to semi-automatically or automatically generate source code of software. However, previous methods fail to tackle the imbalance bias problem. To solve this problem, We propose a model that uses Gradient Harmonizing Mechanism(GHM) technology to alleviate the difference in the amount of data of different categories in the data. The proposed model first calculates the gradient of each sample, that is, the difference between the classification result and the label value, which is used to indicate the difficulty of classification, and then calculates the gradient density. Finally, take the reciprocal of the gradient density as the weight of the loss value calculation function, reconstruct the loss value calculation function, so as to reduce the influence of the data with too large gradient density on model training, so as to alleviating the data imbalance in the data set. The proposed method is evaluated through four experiments on our dataset. The results show that the results obtained by the Code Cloze model(CCM) outperforms the traditional model and the recurrent neural network. Compared with RNN, NBM and SVM models, the average accuracy in all programming languages is improved by 32.9%, 224.7% and 517.9%, respectively.
更多查看译文
关键词
Code cloze test,Imbalance technology,Code automation
AI 理解论文
溯源树
样例
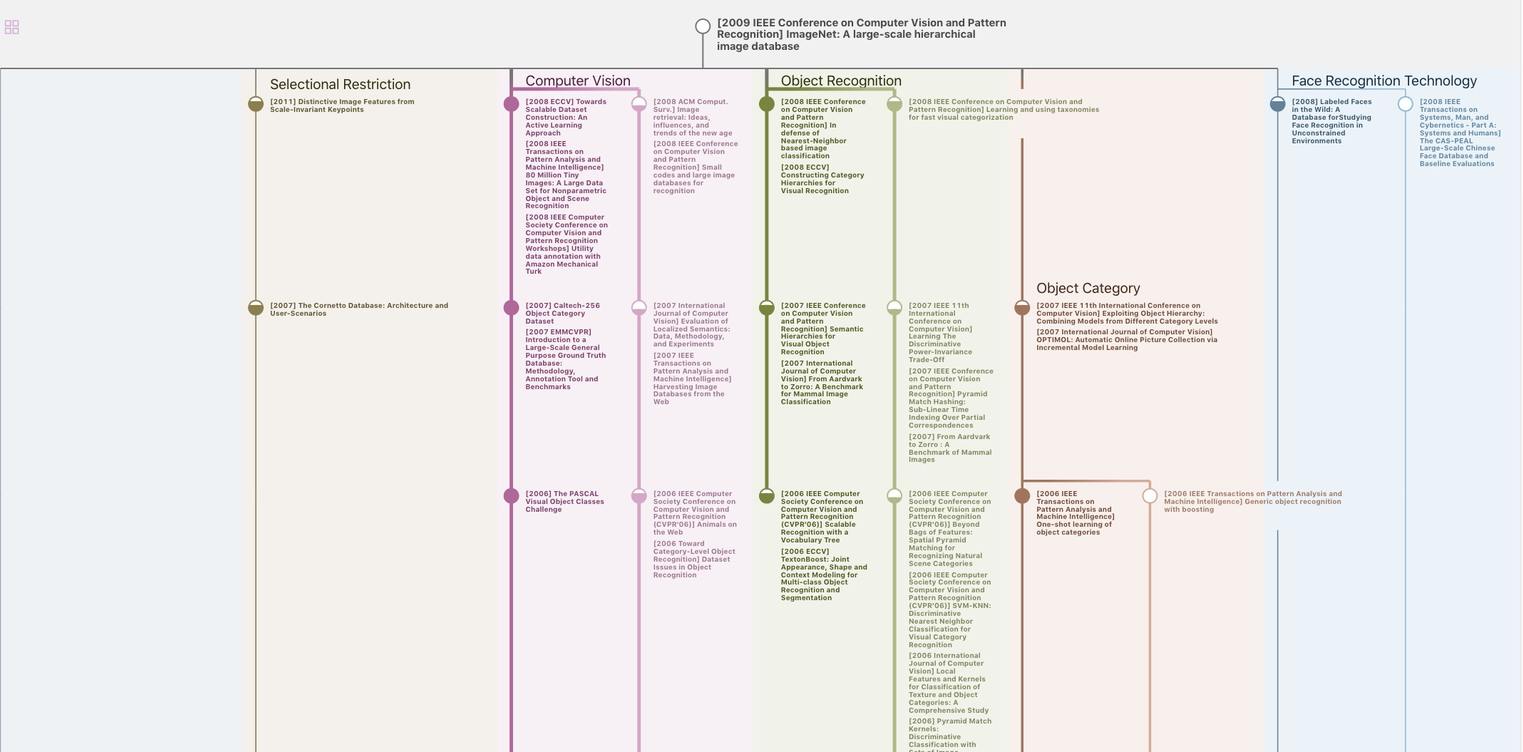
生成溯源树,研究论文发展脉络
Chat Paper
正在生成论文摘要