Enhanced Transfer Learning Strategies for Effective Kidney Tumor Classification with CT Imaging
INTERNATIONAL JOURNAL OF ADVANCED COMPUTER SCIENCE AND APPLICATIONS(2023)
摘要
Kidney tumours (KTs) rank seventh in global tumour prevalence among both males and females, posing a significant health challenge worldwide. Early detection of KT plays a crucial role in reducing mortality rates, mitigating side effects, and effectively treating the tumor. In this context, computer-assisted diagnosis (CAD) offers promising benefits, such as improved test accuracy, cost reduction, and timesaving compared to manual detection, which is known to be laborious and time-consuming. This research investigates the feasibility of employing machine learning (ML) and Fine-tuned Transfer Learning (TL) to improve KT detection. CT images of individuals with and without kidney tumors were utilized to train the models. The study explores three different image dimensions: 32x32, 64x64, and 128x128 pixels, employing the Grey Level Cooccurrence Matrix (GLCM) for feature engineering. The GLCM uses pixel pairs' distance (d) and angle (?) to calculate their occurrence in the image. Various detection approaches, including Random Forest (RF), Support Vector Machine (SVM), Gradient Boosting (GB), and Light Gradient Boosting Model (LGBM), were applied to identify KTs in CT images for diagnostic purposes. Additionally, the study experimented with fine-tuned ResNet-101 and DenseNet-121 models for more effective computer-assisted diagnosis of KT. Evaluation of the efficient diagnostics of fine-tuned ResNet-101 and DenseNet-121 was conducted by comparing their performance with four ML models 121 achieved the highest accuracy of 94.09%, precision of 95.10%, recall of 93.5%, and F1-score of 93.95% when using 32x32 input images. These results outperformed other models and even surpassed state-of-the-art methods. This research classifying KT in CT kidney scans using ML approaches. The use of fine-tuned ResNet-101 and DenseNet-121 shows promising diagnosis of kidney tumors.
更多查看译文
关键词
effective kidney tumor classification,effective imaging,transfer
AI 理解论文
溯源树
样例
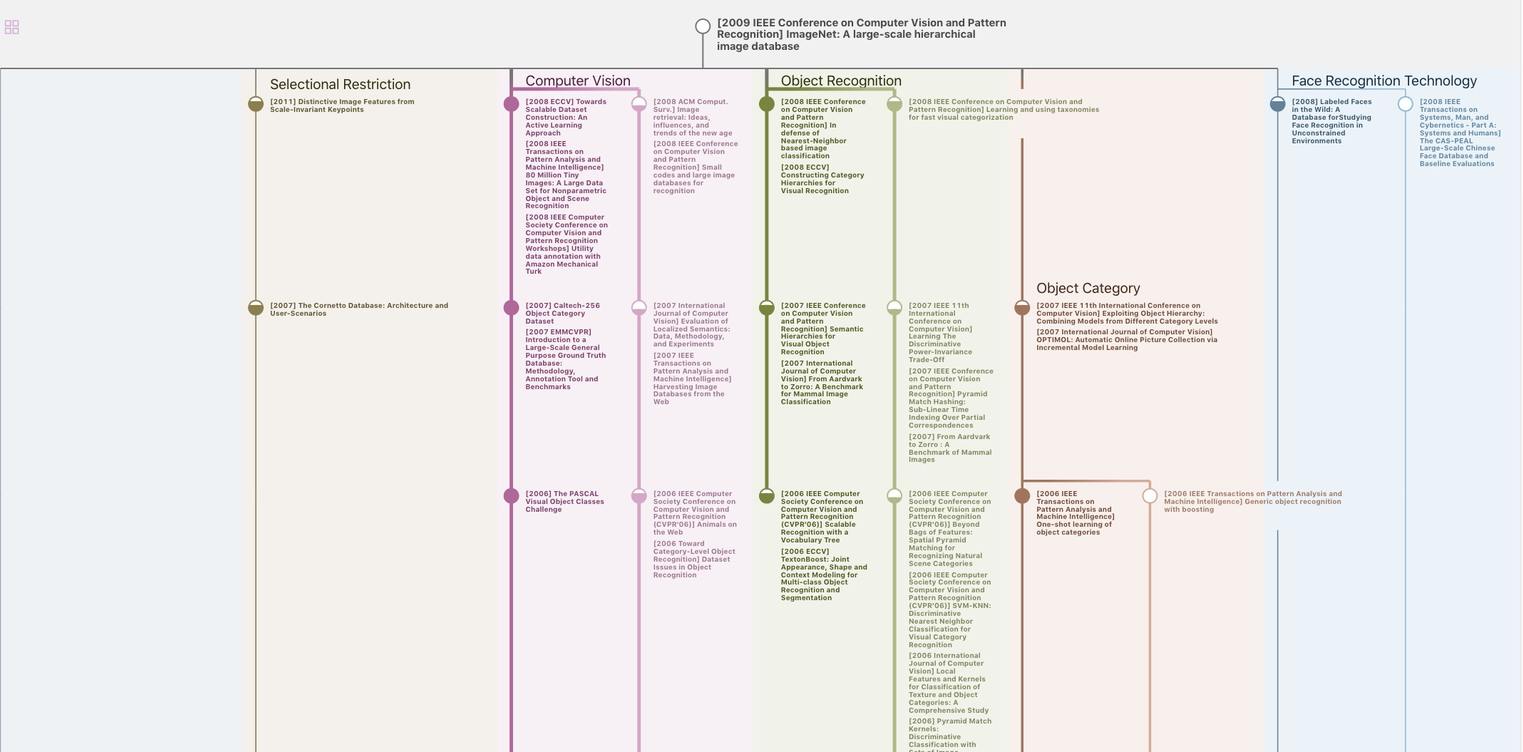
生成溯源树,研究论文发展脉络
Chat Paper
正在生成论文摘要