Identifying underlying patterns in Alzheimer's disease trajectory: a deep learning approach and Mendelian randomization analysis
EClinicalMedicine(2023)
摘要
Background: Alzheimer's disease (AD) is a heterogeneously progressive neurodegeneration disorder with varied rates of deterioration, either between subjects or within different stages of a certain subject. Estimating the course of AD at early stages has treatment implications. We aimed to analyze disease progression to identify distinct patterns in AD trajectory.Methods: We proposed a deep learning model to identify underlying patterns in the trajectory from cognitively normal (CN) to a state of mild cognitive impairment (MCI) to AD dementia, by jointly predicting time-to-conversion and clustering out distinct subgroups characterized by comprehensive features as well as varied progression rates. We designed and validated our model on the ADNI dataset (1370 participants). Prediction of time-to-conversion in AD trajectory was used to validate the expression of the identified patterns. Causality between patterns and time-to-conversion was further inferred using Mendelian randomization (MR) analysis. External validation was performed on the AIBL dataset (233 participants).Findings: The proposed model clustered out patterns characterized by significantly different biomarkers and varied progression rates. The discovered patterns also showed a strong prediction ability, as indicated by hazard ratio (CN -> MCI, HR = 3.51, p < 0.001; MCI -> AD, HR = 8.11, p < 0.001), C-Index (CN -> MCI, 0.618; MCI -> AD, 0.718), and AUC (CN -> MCI, 3 years 0.802, 5 years 0.876; MCI -> AD, 3 years 0.914, 5 years 0.957). In the external validation cohort, our model demonstrated competitive performance on conversion time prediction (CN -> MCI, C-Index = 0.693; MCI -> AD, C-Index = 0.752). Moreover, suggestive associations between CN -> MCI/MCI -> AD patterns with four/three SNPs were mediated and MR analysis indicated a causal link between MCI -> AD patterns and time-to-conversion in the first three years.Interpretation: Our proposed model identifies biologically and clinically meaningful patterns from real-world data and provides promising performance on time-to-conversion prediction in AD trajectory, which could promote the understanding of disease progression, facilitate clinical trial design, and provide potential for decision-making.
更多查看译文
关键词
Alzheimer's disease,Progression pattern,Deep learning,Time-to-conversion prediction,Mendelian randomization
AI 理解论文
溯源树
样例
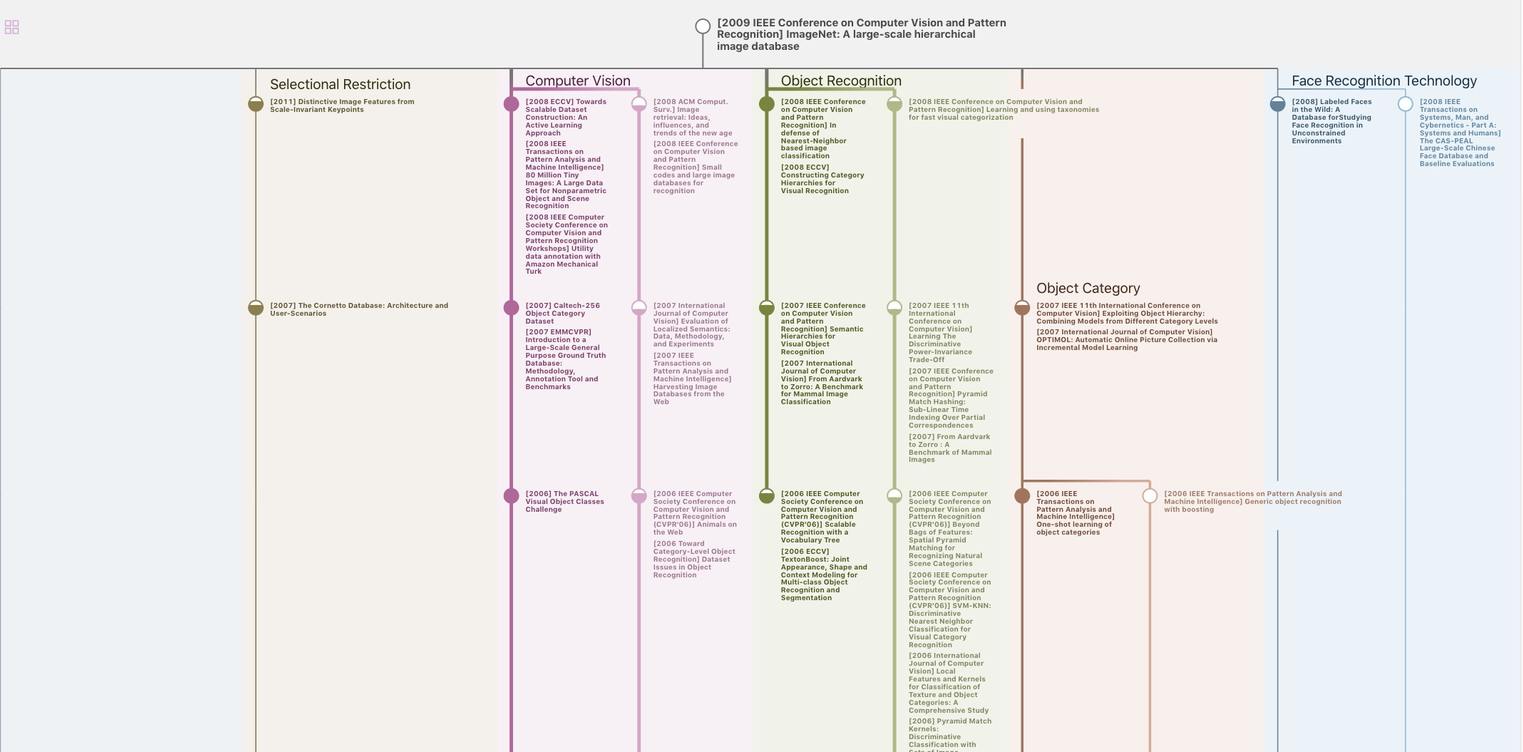
生成溯源树,研究论文发展脉络
Chat Paper
正在生成论文摘要