A framework to generate sparsity-inducing regularizers for enhanced low-rank matrix completion
CoRR(2023)
摘要
Applying half-quadratic optimization to loss functions can yield the corresponding regularizers, while these regularizers are usually not sparsity-inducing regularizers (SIRs). To solve this problem, we devise a framework to generate an SIR with closed-form proximity operator. Besides, we specify our framework using several commonly-used loss functions, and produce the corresponding SIRs, which are then adopted as nonconvex rank surrogates for low-rank matrix completion. Furthermore, algorithms based on the alternating direction method of multipliers are developed. Extensive numerical results show the effectiveness of our methods in terms of recovery performance and runtime.
更多查看译文
关键词
regularizers,completion,matrix,sparsity-inducing,low-rank
AI 理解论文
溯源树
样例
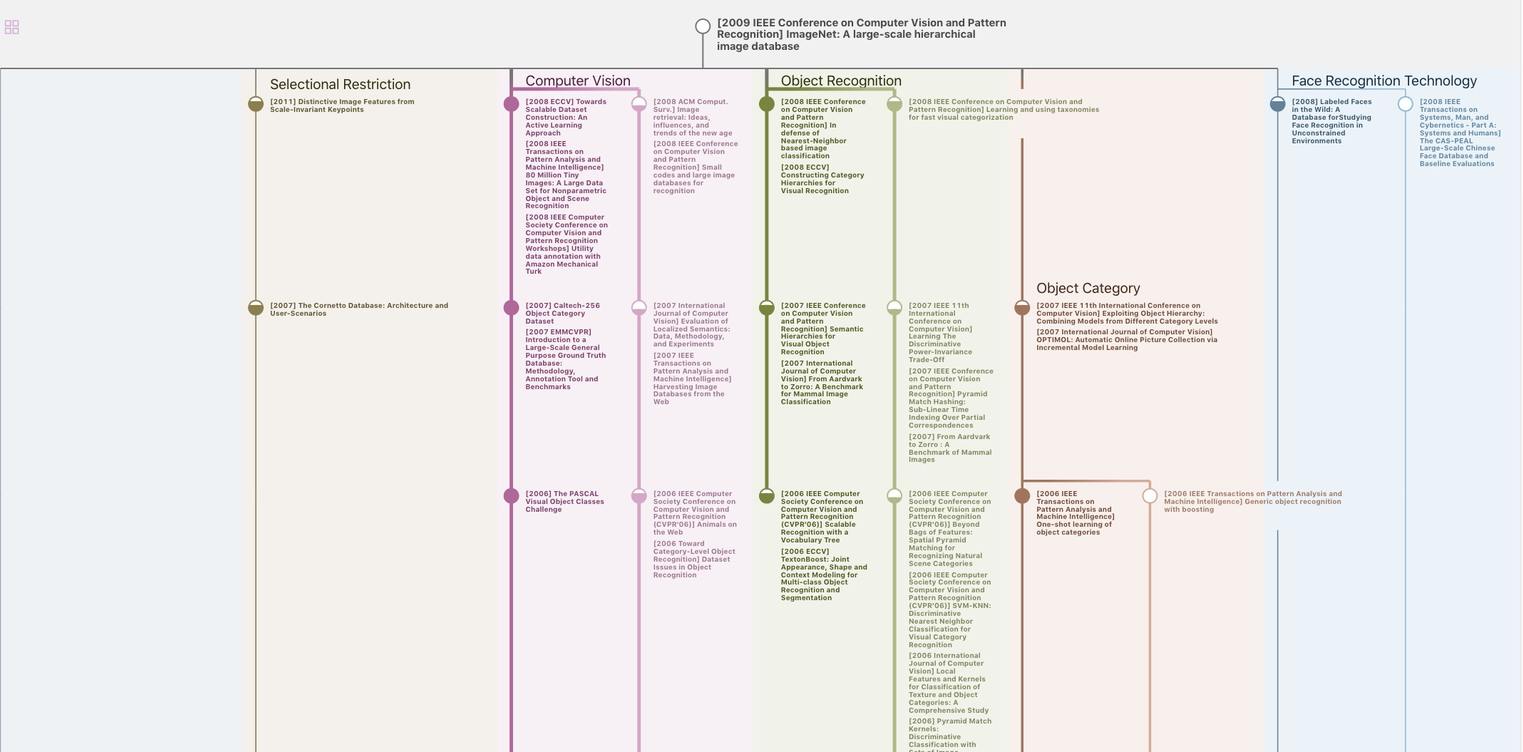
生成溯源树,研究论文发展脉络
Chat Paper
正在生成论文摘要