Optimal Feature Selection via Bayesian Optimisation for Acoustic Condition Monitoring
2023 IEEE 14th International Symposium on Diagnostics for Electrical Machines, Power Electronics and Drives (SDEMPED)(2023)
摘要
Efficient diagnosis of machine faults is important for predictive maintenance and asset management of industrial systems. This paper investigates acoustic techniques as a non-destructive and resource-optimized alternative for machine fault diagnosis. Relevant data analysis techniques usually involve signal time-frequency analysis, feature extraction, and fault classification. However, there are many feature sets that can be extracted, some of which may be redundant or even cause negative effect to the fault classification task. Thus, it is beneficial to select the most informative features for solving a specific task. This paper investigates the use of Bayesian optimization to select the optimal acoustic features, and the method is validated using a laboratory case study for bearing fault diagnosis. This feasibility study helps set up a machine learning framework for further meta-model development that could select optimal features, models and hyperparameters simultaneously.
更多查看译文
关键词
machine fault diagnosis,signal processing,feature extraction,optimal features,Bayesian optimization
AI 理解论文
溯源树
样例
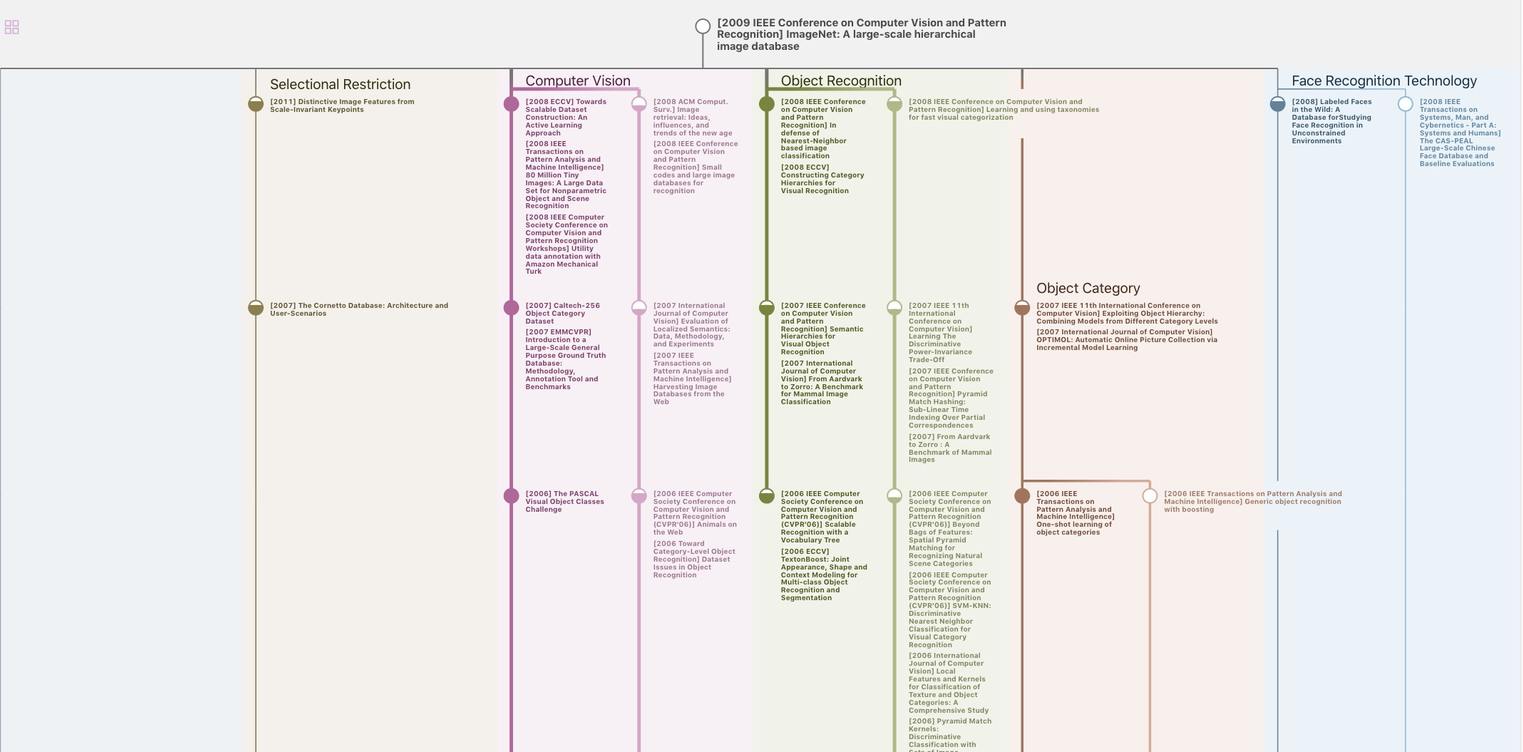
生成溯源树,研究论文发展脉络
Chat Paper
正在生成论文摘要