Sparsistency for Inverse Optimal Transport
ICLR 2024(2023)
摘要
Optimal Transport is a useful metric to compare probability distributions and to compute a pairing given a ground cost. Its entropic regularization variant (eOT) is crucial to have fast algorithms and reflect fuzzy/noisy matchings. This work focuses on Inverse Optimal Transport (iOT), the problem of inferring the ground cost from samples drawn from a coupling that solves an eOT problem. It is a relevant problem that can be used to infer unobserved/missing links, and to obtain meaningful information about the structure of the ground cost yielding the pairing. On one side, iOT benefits from convexity, but on the other side, being ill-posed, it requires regularization to handle the sampling noise. This work presents an in-depth theoretical study of the l1 regularization to model for instance Euclidean costs with sparse interactions between features. Specifically, we derive a sufficient condition for the robust recovery of the sparsity of the ground cost that can be seen as a far reaching generalization of the Lasso's celebrated Irrepresentability Condition. To provide additional insight into this condition, we work out in detail the Gaussian case. We show that as the entropic penalty varies, the iOT problem interpolates between a graphical Lasso and a classical Lasso, thereby establishing a connection between iOT and graph estimation, an important problem in ML.
更多查看译文
关键词
Optimal transport,sparsity,sparsistency,metric learning
AI 理解论文
溯源树
样例
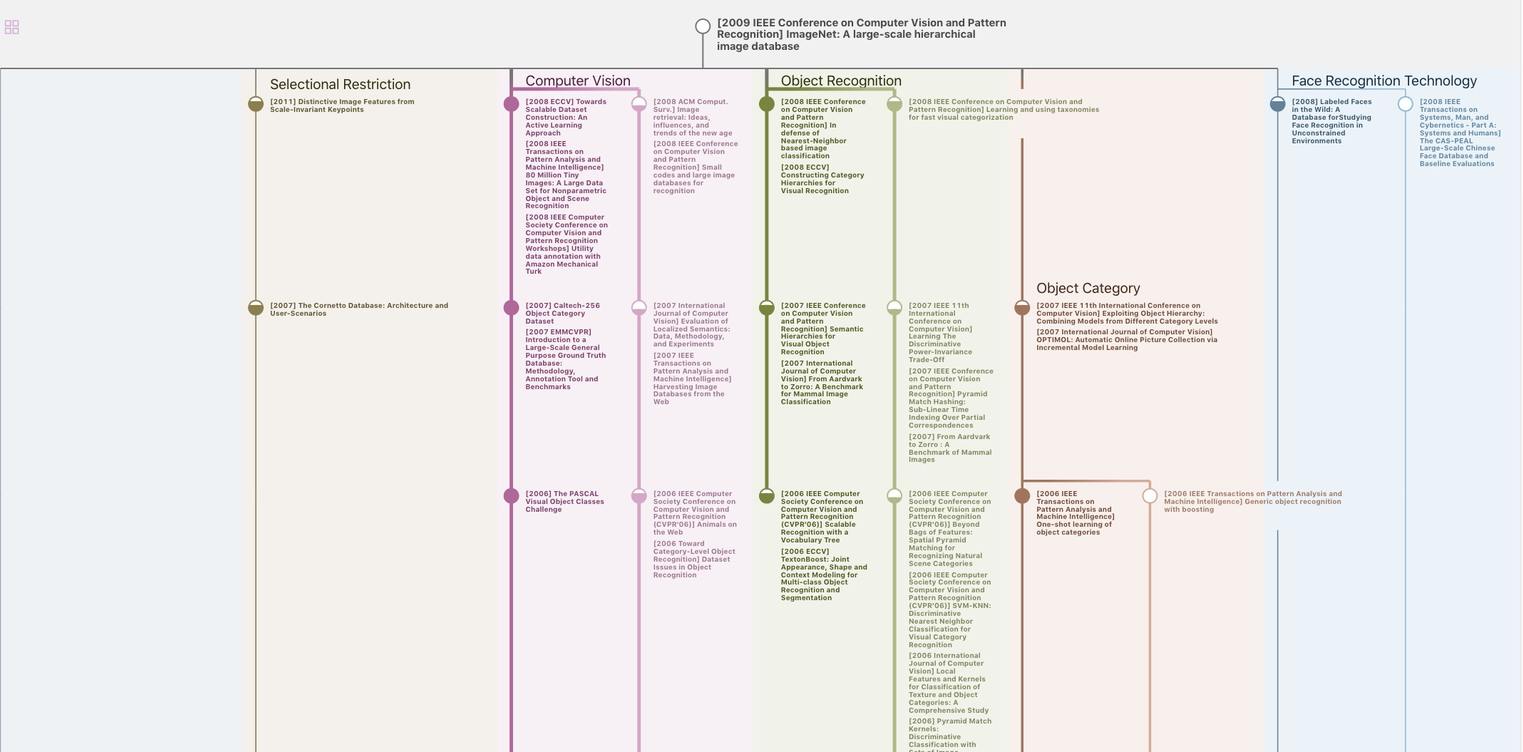
生成溯源树,研究论文发展脉络
Chat Paper
正在生成论文摘要