ASM: Adaptive Sample Mining for In-The-Wild Facial Expression Recognition
PATTERN RECOGNITION AND COMPUTER VISION, PRCV 2023, PT V(2024)
摘要
Given the similarity between facial expression categories, the presence of compound facial expressions, and the subjectivity of annotators, facial expression recognition (FER) datasets often suffer from ambiguity and noisy labels. Ambiguous expressions are challenging to differentiate from expressions with noisy labels, which hurt the robustness of FER models. Furthermore, the difficulty of recognition varies across different expression categories, rendering a uniform approach unfair for all expressions. In this paper, we introduce a novel approach called Adaptive Sample Mining (ASM) to dynamically address ambiguity and noise within each expression category. First, the Adaptive Threshold Learning module generates two thresholds, namely the clean and noisy thresholds, for each category. These thresholds are based on the mean class probabilities at each training epoch. Next, the Sample Mining module partitions the dataset into three subsets: clean, ambiguity, and noise, by comparing the sample confidence with the clean and noisy thresholds. Finally, the Tri-Regularization module employs a mutual learning strategy for the ambiguity subset to enhance discrimination ability, and an unsupervised learning strategy for the noise subset to mitigate the impact of noisy labels. Extensive experiments prove that our method can effectively mine both ambiguity and noise, and outperform SOTA methods on both synthetic noisy and original datasets. The supplement material is available at https://github.com/zzzzzzyang/ASM.
更多查看译文
关键词
Facial Expression Recognition,Noisy Label Learning,Adaptive Threshold Mining
AI 理解论文
溯源树
样例
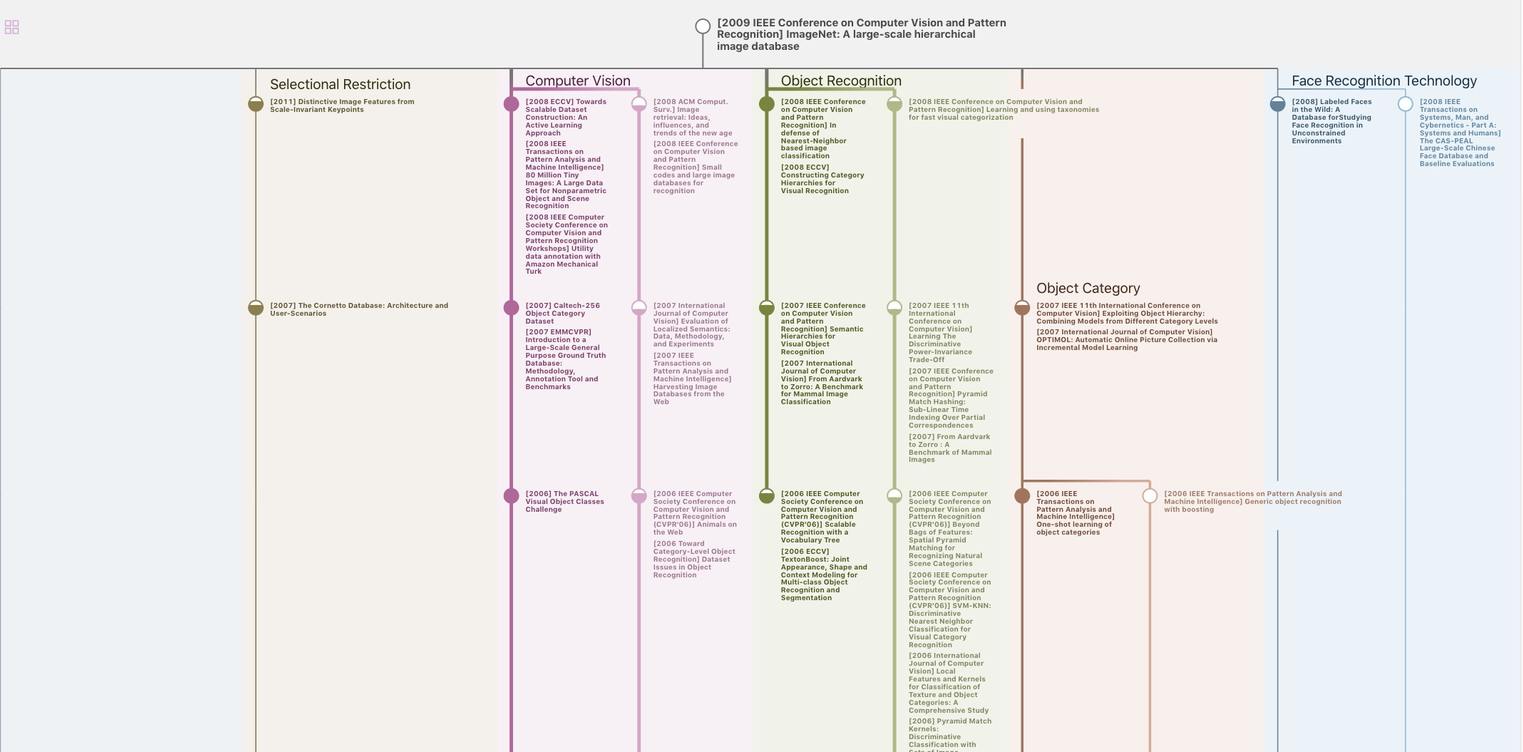
生成溯源树,研究论文发展脉络
Chat Paper
正在生成论文摘要