Predicting prime editing efficiency across diverse edit types and chromatin contexts with machine learning
biorxiv(2023)
摘要
Prime editing is a powerful genome editing technology, but its efficiency varies depending on the pegRNA design and target locus. Existing computational models for predicting prime editing rates are limited by their focus on specific edit types and by omitting the local chromatin environment. In our study, we developed machine learning models that predict prime editing efficiencies across a wide range of edit types up to 15 bp (’PRIDICT2.0’) and in different chromatin contexts (’ePRIDICT’). Both models can be accessed at [www.pridict.it][1].
### Competing Interest Statement
G.S. is a scientific advisor to Prime Medicine.
[1]: http://www.pridict.it
更多查看译文
AI 理解论文
溯源树
样例
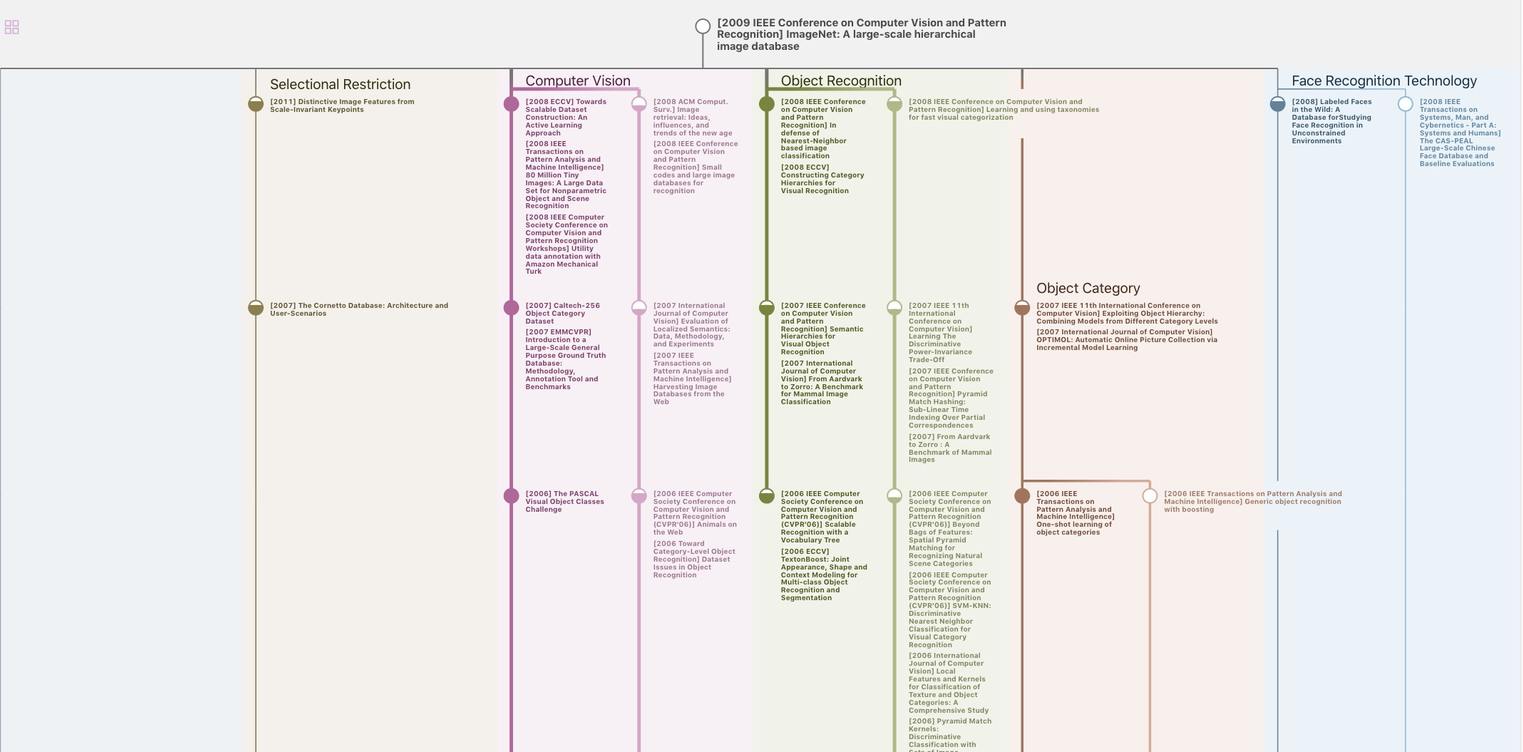
生成溯源树,研究论文发展脉络
Chat Paper
正在生成论文摘要