SRLoRa: Neural-enhanced LoRa Weak Signal Decoding with Multi-gateway Super Resolution
PROCEEDINGS OF THE 2023 INTERNATIONAL SYMPOSIUM ON THEORY, ALGORITHMIC FOUNDATIONS, AND PROTOCOL DESIGN FOR MOBILE NETWORKS AND MOBILE COMPUTING, MOBIHOC 2023(2023)
关键词
Internet-of-Things,Low-Power Wide Area Networks,LoRa,Machine Learning
AI 理解论文
溯源树
样例
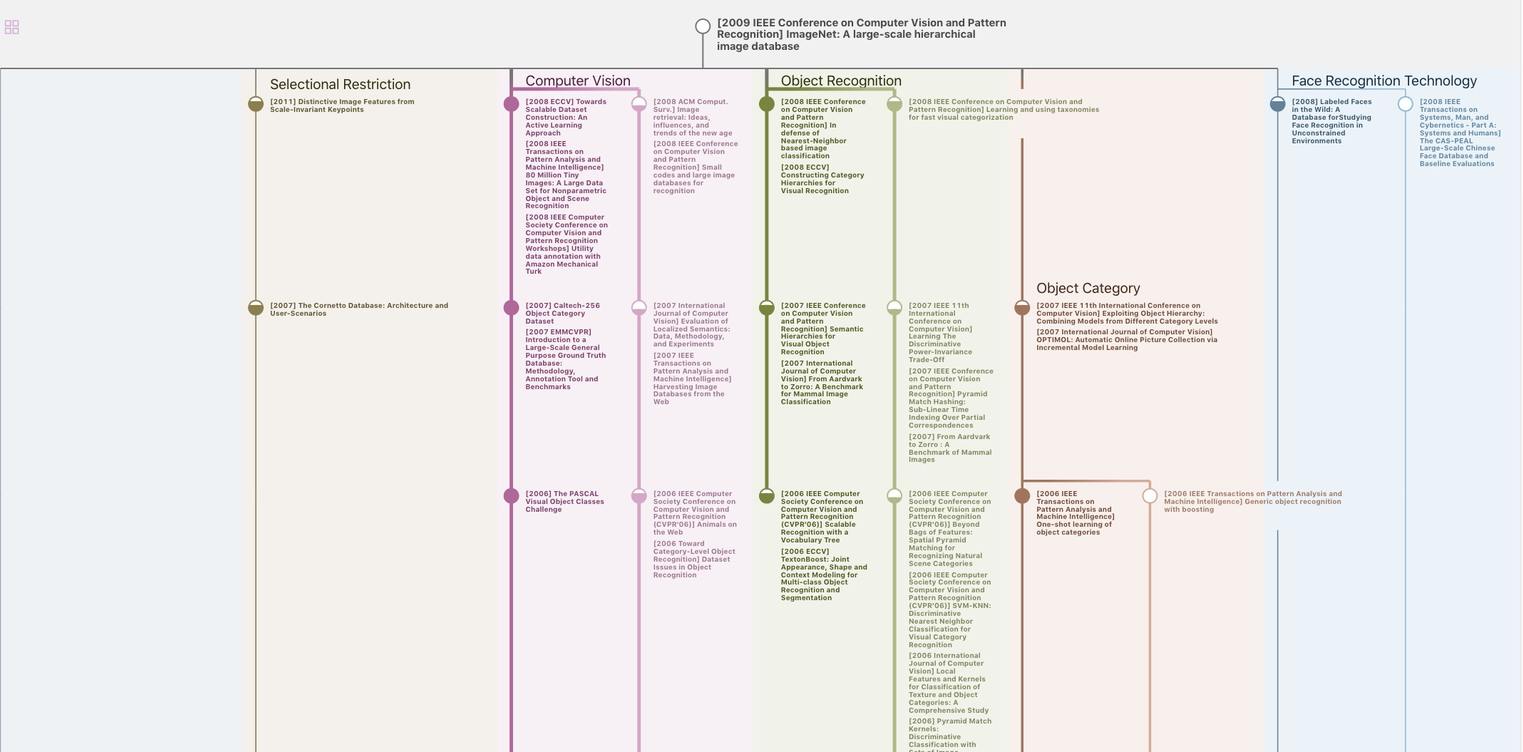
生成溯源树,研究论文发展脉络
Chat Paper
正在生成论文摘要