Training Robust Deep Collaborative Filtering Models via Adversarial Noise Propagation
ACM TRANSACTIONS ON INFORMATION SYSTEMS(2024)
摘要
The recommendation performance of deep collaborative filtering models drops sharply under imperceptible adversarial perturbations. Some methods promote the robustness of recommendation systems by adversarial training. However, these methods only study shallow models and lack the exploration of deep models. Furthermore, the way these methods add adversarial noise to the weight parameters of users and items is not fully applicable to deep collaborative filtering models, because the adversarial noise is not sufficient to fully affect its network structure with multiple hidden layers. In this article, we propose a novel adversarial training framework, Random Layer-wise Adversarial Training (RAT), which trains a robust deep collaborative filtering model via adversarial noise propagation. Specifically, we inject adversarial noise into the output of the hidden layer in a random layer-wise manner. The adversarial noise propagates forward from the injected position to obtain more flexible model parameters during the adversarial training process. We validate the effectiveness of RAT on multilayer perceptron (MLP) and implement RAT on MLP-based and convolutional neural networks-based deep collaborative filtering models. Experiments on three publicly available datasets show that the deep collaborative filtering model trained by RAT not only defends against adversarial noise but also guarantees recommendation performance.
更多查看译文
关键词
Recommendation systems,deep collaborative filtering,adversarial training
AI 理解论文
溯源树
样例
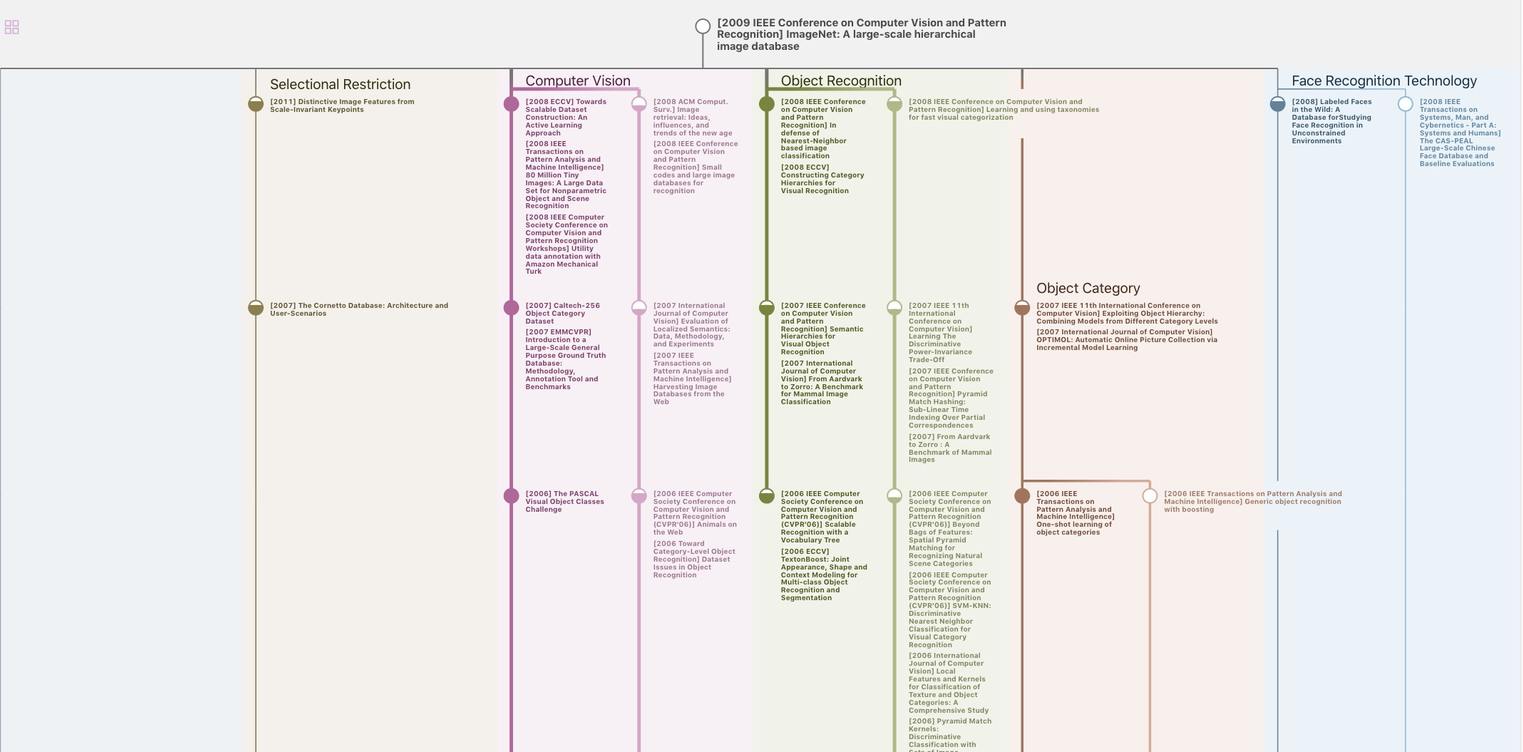
生成溯源树,研究论文发展脉络
Chat Paper
正在生成论文摘要