Deep Learning Models for Serendipity Recommendations: A Survey and New Perspectives
ACM COMPUTING SURVEYS(2024)
摘要
Serendipitous recommendations have emerged as a compelling approach to deliver users with unexpected yet valuable information, contributing to heightened user satisfaction and engagement. This survey presents an investigation of the most recent research in serendipity recommenders, with a specific emphasis on deep learning recommendation models. We categorize these models into three types, distinguishing their integration of the serendipity objective across distinct stages: pre-processing, in-processing, and post-processing. Additionally, we provide a review and summary of the serendipity definition, available ground truth datasets, and evaluation experiments employed in the field. We propose three promising avenues for future exploration: (1) leveraging user reviews to identify and explore serendipity, (2) employing reinforcement learning to construct a model for discerning appropriate timing for serendipitous recommendations, and (3) utilizing cross-domain learning to enhance serendipitous recommendations. With this review, we aim to cultivate a deeper understanding of serendipity in recommender systems and inspire further advancements in this domain.
更多查看译文
关键词
Deep learning,recommendation models,serendipity recommendations
AI 理解论文
溯源树
样例
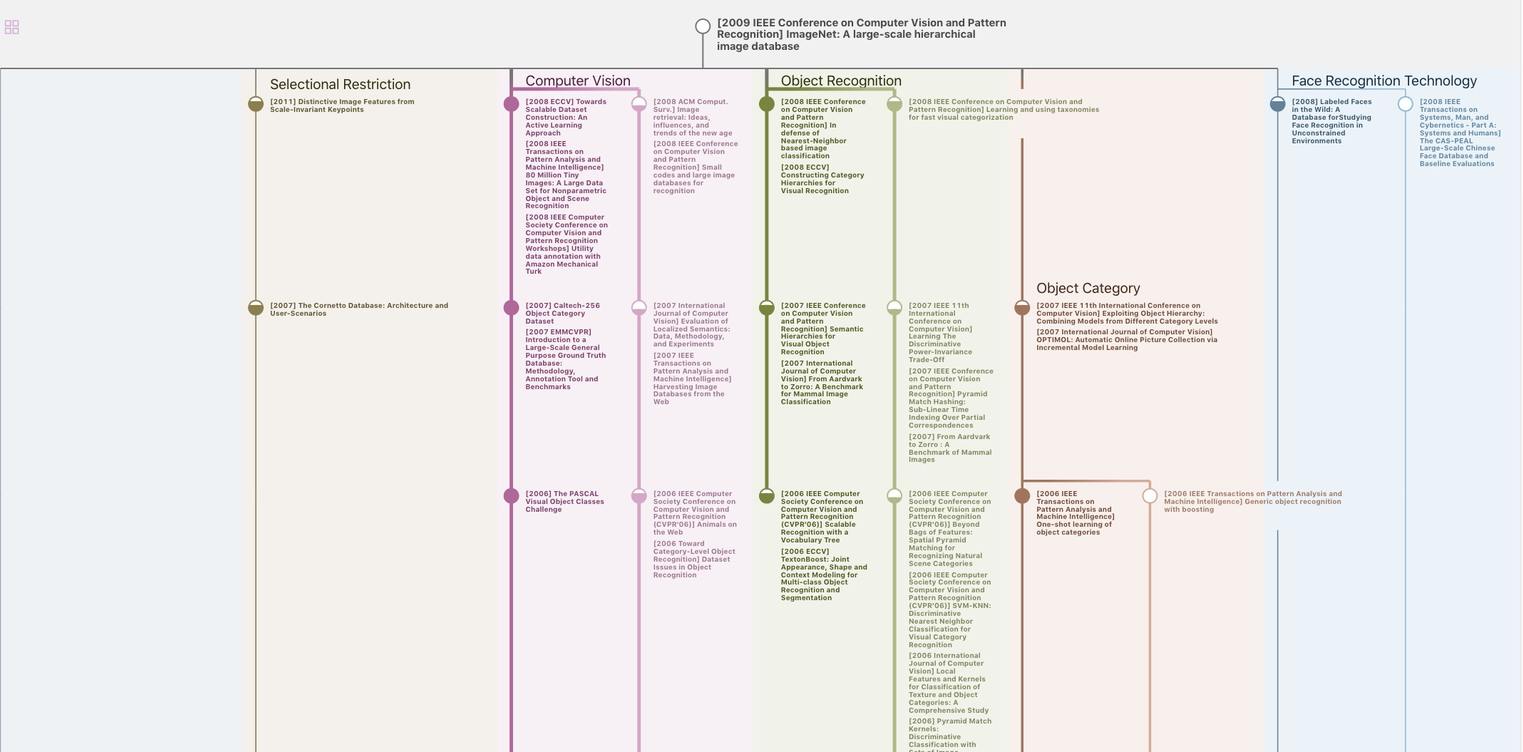
生成溯源树,研究论文发展脉络
Chat Paper
正在生成论文摘要