Wireless Channel Prediction in Different Locations Using Transfer Learning
PROCEEDINGS OF THE 2023 INTERNATIONAL SYMPOSIUM ON THEORY, ALGORITHMIC FOUNDATIONS, AND PROTOCOL DESIGN FOR MOBILE NETWORKS AND MOBILE COMPUTING, MOBIHOC 2023(2023)
摘要
Transfer learning refers to transferring the knowledge of a specific domain to a related domain. In cases where the source and the target learner have similar distribution and parameters, transfer learning can reduce the cost of learning and the construction of the target learner and improve the performance of the target learner. In wireless ad-hoc networks, the users connect to networks based on the service location, and various network channels with different levels of quality-of-service (QoS) are available. The wireless channels represent specific ranges of radio frequencies. When the users move from one location to another, the mobile application may switch channels for good quality of service. This paper predicts the wireless channel based on the user's location. Since channel prediction based on location is feasible in one city, the knowledge of channel prediction in one city can be transferred to another city. Thus, transfer learning is applicable and effective in such applications. The paper uses two cities' wireless mapping datasets to predict network channels and uses transfer learning to predict one city's network channels based on the other city's model. Experiments using different initial learning rates during training and different source and target domain data ratios show that transfer learning is feasible for network prediction among different cities.
更多查看译文
关键词
Cellular networks,deep learning,transfer learning.
AI 理解论文
溯源树
样例
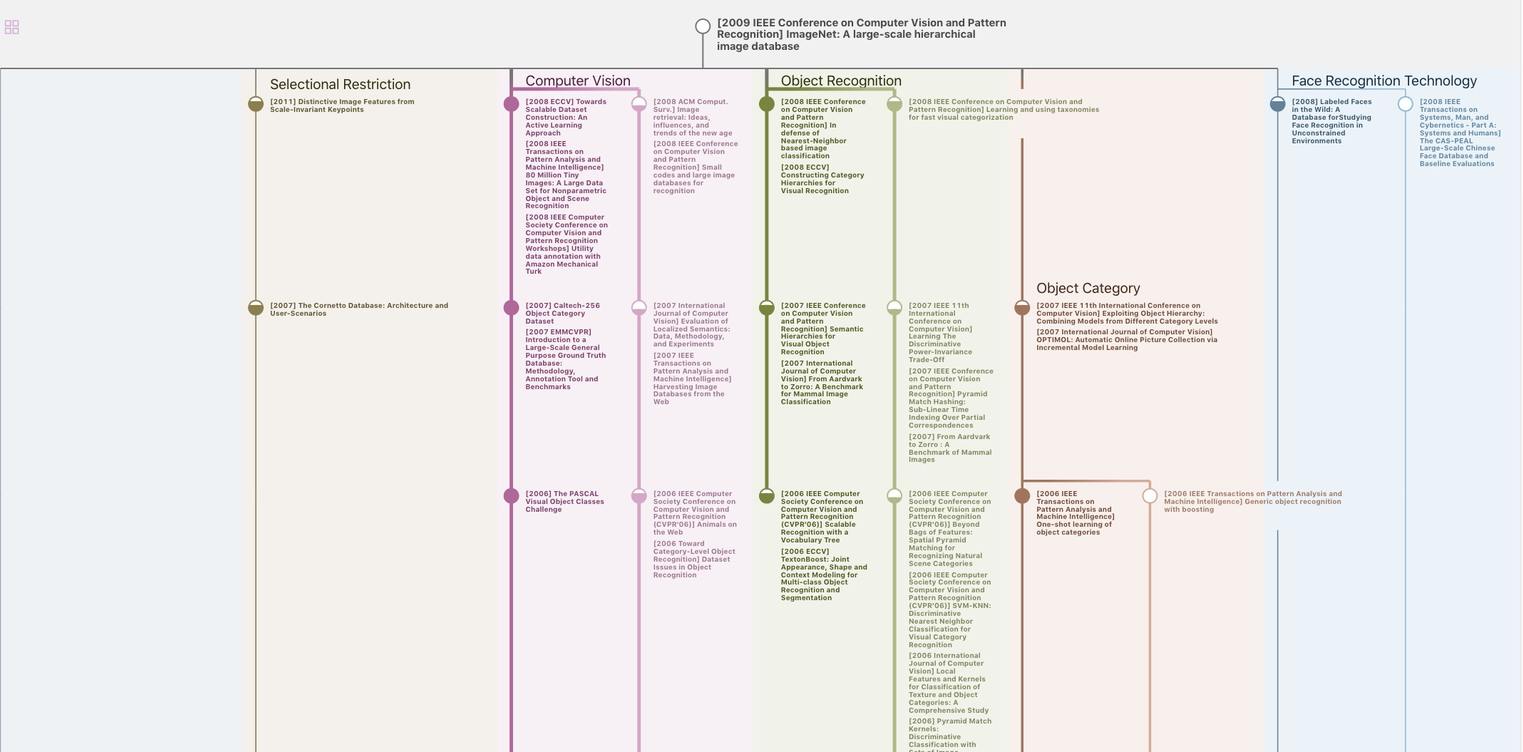
生成溯源树,研究论文发展脉络
Chat Paper
正在生成论文摘要