Can Data Augmentation Improve Daily Mood Prediction from Wearable Data? An Empirical Study
UbiComp/ISWC '23 Adjunct: Adjunct Proceedings of the 2023 ACM International Joint Conference on Pervasive and Ubiquitous Computing & the 2023 ACM International Symposium on Wearable Computing(2023)
摘要
Mobile sensing data, approximating human behavior and physiology, can be processed by machine learning models to predict mental health symptoms. While these models are accurate in smaller samples, their generalization accuracy decreases in larger samples, potentially because it is difficult to collect enough mobile sensing and mental health outcomes data at scale to enable generalization. In this study, we hypothesized that augmenting training data with synthetic data samples could improve the generalizability of these machine learning models. We created a data augmentation system that generated synthetic mobile sensing and mental health outcomes data, and evaluated the utility of this system via the downstream machine learning task of predicting daily mood from wearable sensing data. We experimented with both simple (e.g. noise addition) and novel generative data augmentation methods, based upon conditional generative adversarial networks and multi-task learning. Our initial findings suggest that the data augmentation system generated realistic synthetic data, but did not improve mood prediction. We propose future work to validate our findings and test other methods to improve the generalizability of mental health symptom prediction models.
更多查看译文
AI 理解论文
溯源树
样例
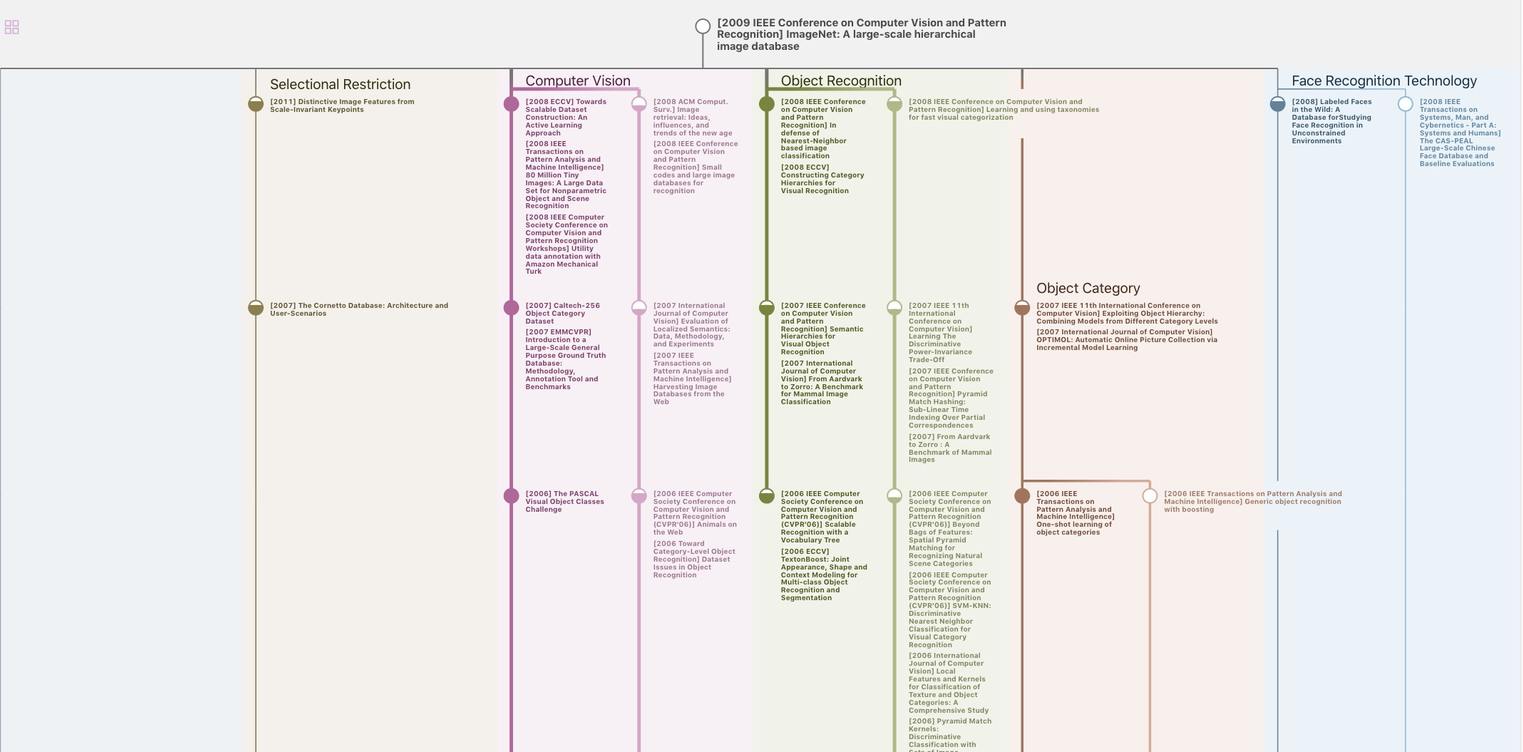
生成溯源树,研究论文发展脉络
Chat Paper
正在生成论文摘要