Fusion-based Representation Learning Model for Multimode User-generated Social Network Content
Journal of Data and Information Quality(2023)
摘要
As mobile networks and APPs are developed, user-generated content (UGC), which includes multi-source heterogeneous data like user reviews, tags, scores, images, and videos, has become an essential basis for improving the quality of personalized services. Due to the multi-source heterogeneous nature of the data, big data fusion offers both promise and drawbacks. With the rise of mobile networks and applications, UGC, which includes multi-source heterogeneous data including ratings, marks, scores, images, and videos, has gained importance. This information is very important for improving the calibre of customized services. The key to the application's success is representational learning of fusing and vectorization on the multi-source heterogeneous UGC. Multi-source text fusion and representation learning have become the key to its application. In this regard, a fusion representation learning for multi-source text and image is proposed. The convolutional fusion technique, in contrast to splicing and fusion, may take into consideration the varied data characteristics in each size. This research proposes a new data feature fusion strategy based on the convolution operation, which was inspired by the convolutional neural network. Using Doc2vec and LDA model, the vectorized representation of multi-source text is given, and the deep convolutional network is used to obtain it. Finally, the proposed algorithm is applied to Amazon's commodity dataset containing UGC content based on the classification accuracy of UGC vectorized representation items and shows the feasibility and impact of the proposed algorithm.
更多查看译文
关键词
User-generated content,social networks,vectorization,fusion mechanism
AI 理解论文
溯源树
样例
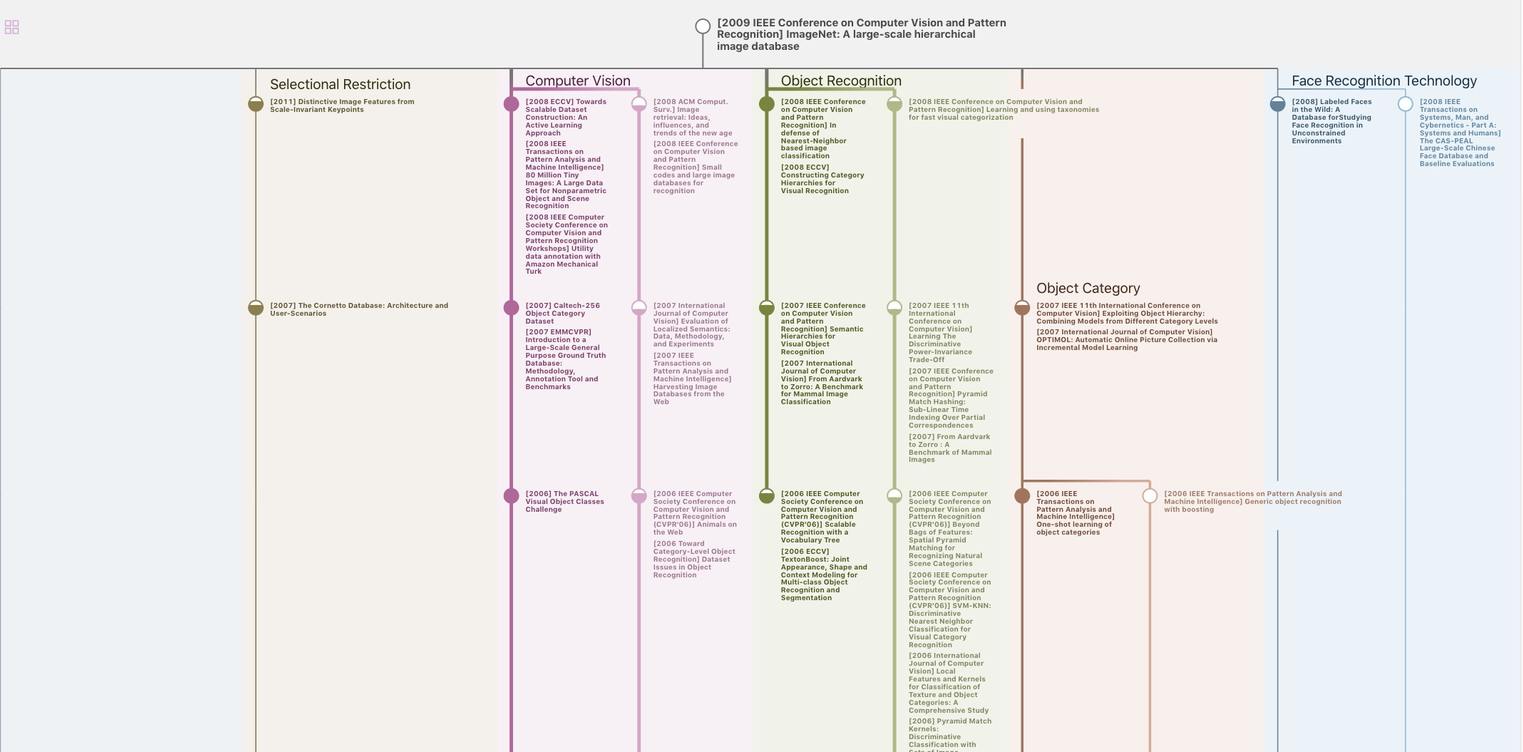
生成溯源树,研究论文发展脉络
Chat Paper
正在生成论文摘要