21-cm foreground removal using AI and frequency-difference technique
arxiv(2023)
摘要
The deep learning technique has been employed in removing foreground
contaminants from 21 cm intensity mapping, but its effectiveness is limited by
the large dynamic range of the foreground amplitude. In this study, we develop
a novel foreground removal technique grounded in U-Net networks. The essence of
this technique lies in introducing an innovative data preprocessing step
specifically, utilizing the temperature difference between neighboring
frequency bands as input, which can substantially reduce the dynamic range of
foreground amplitudes by approximately two orders of magnitude. This reduction
proves to be highly advantageous for the U-Net foreground removal. We observe
that the HI signal can be reliably recovered, as indicated by the
cross-correlation power spectra showing unity agreement at the scale of k <
0.3 h^-1Mpc in the absence of instrumental effects. Moreover, accounting for
the systematic beam effects, our reconstruction displays consistent
auto-correlation and cross-correlation power spectrum ratios at the 1σ
level across scales k ≲ 0.1 h^-1Mpc, with only a 10
observed in the cross-correlation power spectrum at k≃0.2 h^-1Mpc. The
effects of redshift-space distortion are also reconstructed successfully, as
evidenced by the quadrupole power spectra matching. In comparison, our method
outperforms the traditional Principal Component Analysis method, which derived
cross-correlation ratios are underestimated by around 60
white noise levels in the map and found that the mean cross-correlation ratio
R̅_cross≳ 0.8 when the level of the thermal noise is
smaller than or equal to that of the HI signal. We conclude that the proposed
frequency-difference technique can significantly enhance network performance by
reducing the amplitude range of foregrounds and aiding in the prevention of HI
loss.
更多查看译文
AI 理解论文
溯源树
样例
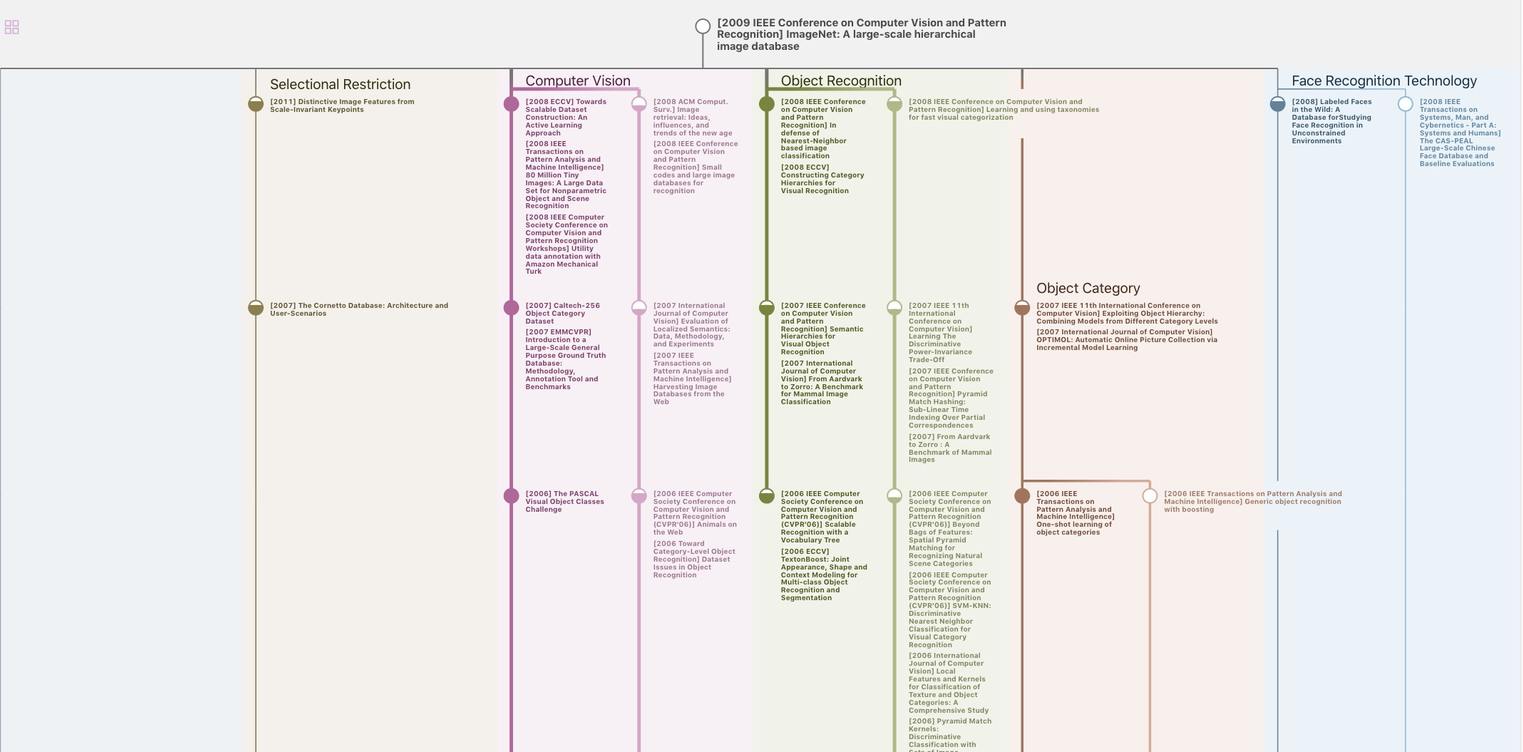
生成溯源树,研究论文发展脉络
Chat Paper
正在生成论文摘要