Deep learning reconstruction with uncertainty estimation for photon interaction in fast scintillator detectors
ENGINEERING APPLICATIONS OF ARTIFICIAL INTELLIGENCE(2024)
摘要
This article presents a physics-informed deep learning method for the quantitative estimation of the spatial coordinates of gamma interactions within a monolithic scintillator, with a focus on Positron Emission Tomography (PET) imaging. A Density Neural Network approach is designed to estimate the 2-dimensional gamma photon interaction coordinates in a fast lead tungstate (PbWO4) monolithic scintillator detector. We introduce a custom loss function to estimate the inherent uncertainties associated with the reconstruction process and to incorporate the physical constraints of the detector. This unique combination allows for more robust and reliable position estimations and the obtained results demonstrate the effectiveness of the proposed approach and highlights the significant benefits of the uncertainties estimation. We discuss its potential impact on improving PET imaging quality and show how the results can be used to improve the exploitation of the model, to bring benefits to the application and how to evaluate the validity of the given prediction and the associated uncertainties. Importantly, our proposed methodology extends beyond this specific use case, as it can be generalized to other applications beyond PET imaging.
更多查看译文
关键词
Deep learning,Neural networks,Uncertainty quantification,Event reconstruction algorithms,Gamma detector,PET imaging
AI 理解论文
溯源树
样例
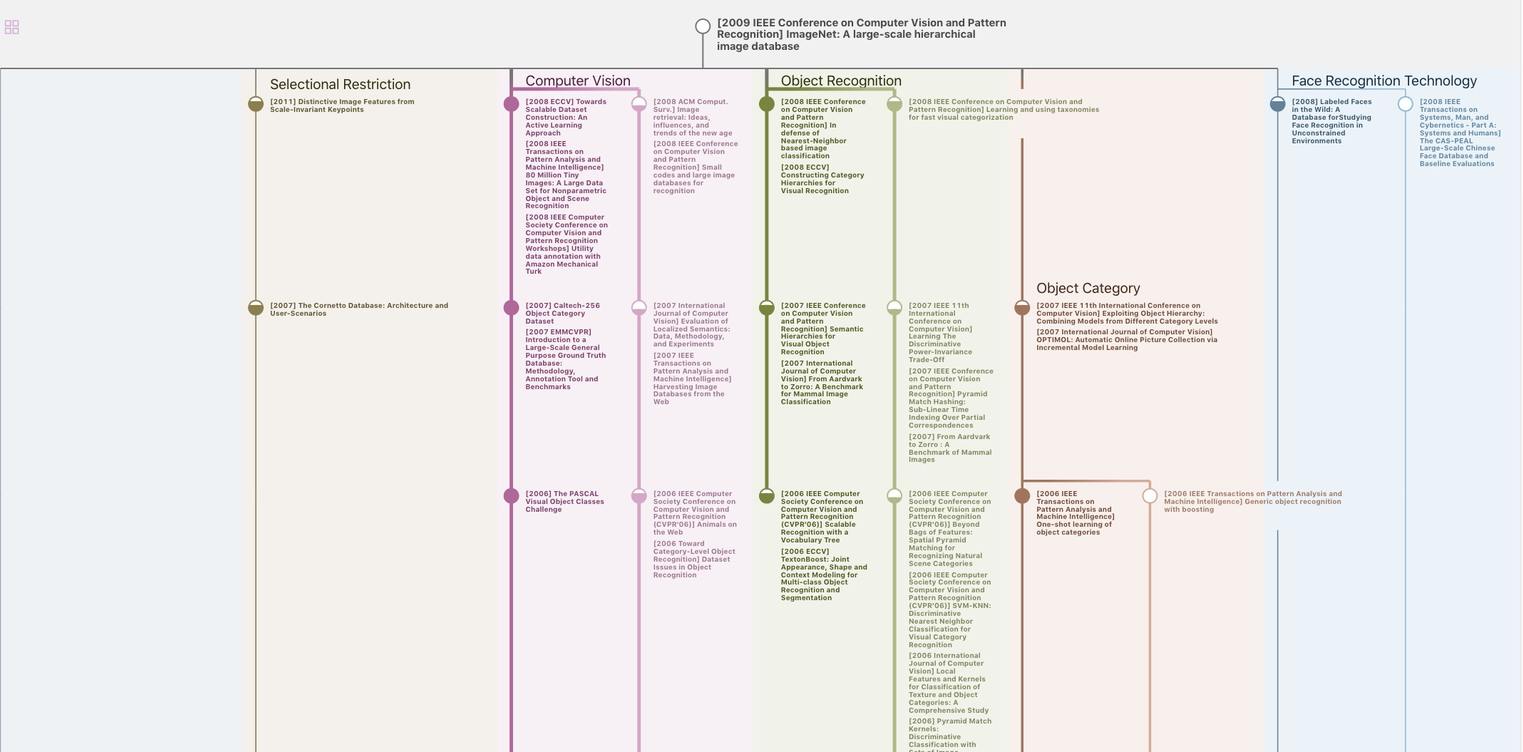
生成溯源树,研究论文发展脉络
Chat Paper
正在生成论文摘要