Pain Forecasting using Self-supervised Learning and Patient Phenotyping: An attempt to prevent Opioid Addiction
CoRR(2023)
摘要
Sickle Cell Disease (SCD) is a chronic genetic disorder characterized by recurrent acute painful episodes. Opioids are often used to manage these painful episodes; the extent of their use in managing pain in this disorder is an issue of debate. The risk of addiction and side effects of these opioid treatments can often lead to more pain episodes in the future. Hence, it is crucial to forecast future patient pain trajectories to help patients manage their SCD to improve their quality of life without compromising their treatment. It is challenging to obtain many pain records to design forecasting models since it is mainly recorded by patients' self-report. Therefore, it is expensive and painful (due to the need for patient compliance) to solve pain forecasting problems in a purely supervised manner. In light of this challenge, we propose to solve the pain forecasting problem using self-supervised learning methods. Also, clustering such time-series data is crucial for patient phenotyping, anticipating patients' prognoses by identifying "similar" patients, and designing treatment guidelines tailored to homogeneous patient subgroups. Hence, we propose a self-supervised learning approach for clustering time-series data, where each cluster comprises patients who share similar future pain profiles. Experiments on five years of real-world datasets show that our models achieve superior performance over state-of-the-art benchmarks and identify meaningful clusters that can be translated into actionable information for clinical decision-making.
更多查看译文
关键词
patient phenotyping
AI 理解论文
溯源树
样例
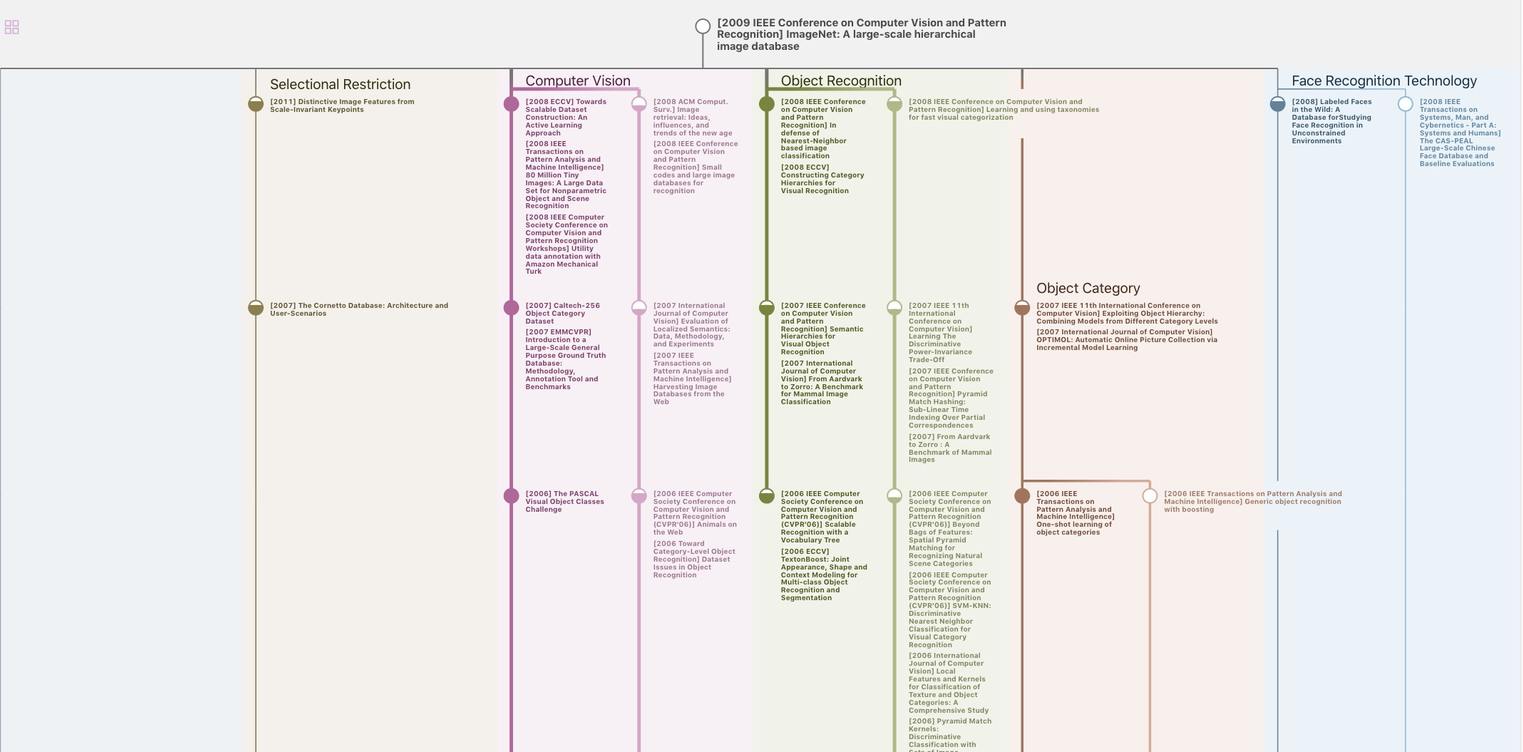
生成溯源树,研究论文发展脉络
Chat Paper
正在生成论文摘要