Learning Layer-wise Equivariances Automatically using Gradients
arXiv (Cornell University)(2023)
摘要
Convolutions encode equivariance symmetries into neural networks leading to better generalisation performance. However, symmetries provide fixed hard constraints on the functions a network can represent, need to be specified in advance, and can not be adapted. Our goal is to allow flexible symmetry constraints that can automatically be learned from data using gradients. Learning symmetry and associated weight connectivity structures from scratch is difficult for two reasons. First, it requires efficient and flexible parameterisations of layer-wise equivariances. Secondly, symmetries act as constraints and are therefore not encouraged by training losses measuring data fit. To overcome these challenges, we improve parameterisations of soft equivariance and learn the amount of equivariance in layers by optimising the marginal likelihood, estimated using differentiable Laplace approximations. The objective balances data fit and model complexity enabling layer-wise symmetry discovery in deep networks. We demonstrate the ability to automatically learn layer-wise equivariances on image classification tasks, achieving equivalent or improved performance over baselines with hard-coded symmetry.
更多查看译文
关键词
learning,layer-wise
AI 理解论文
溯源树
样例
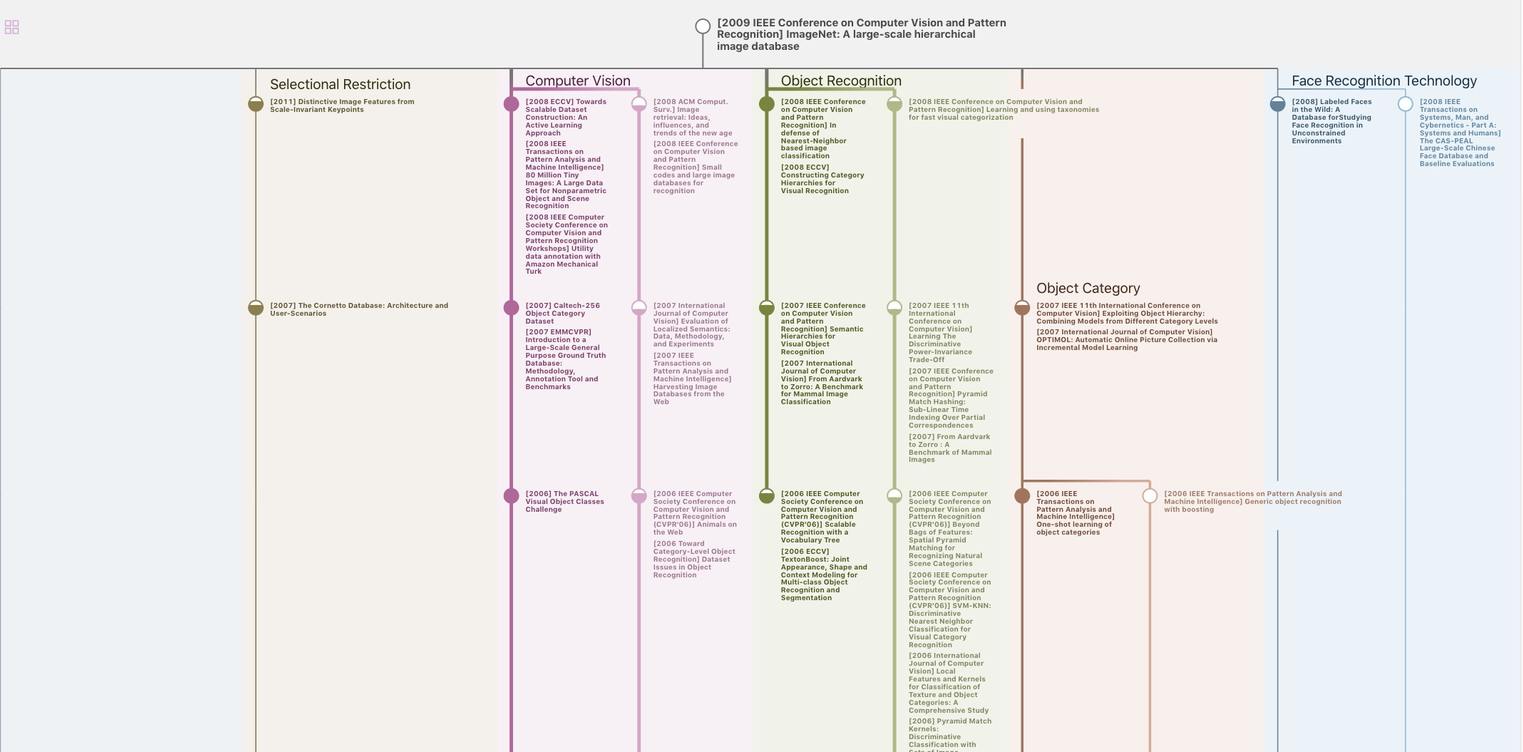
生成溯源树,研究论文发展脉络
Chat Paper
正在生成论文摘要