Jailbreak and Guard Aligned Language Models with Only Few In-Context Demonstrations
arxiv(2023)
摘要
Large Language Models (LLMs) have shown remarkable success in various tasks,
but concerns about their safety and the potential for generating harmful
content have emerged. In this paper, we delve into the potential of In-Context
Learning (ICL) to modulate the alignment of LLMs. Specifically, we propose the
In-Context Attack (ICA), which employs strategically crafted harmful
demonstrations to subvert LLMs, and the In-Context Defense (ICD), which
bolsters model resilience through examples that demonstrate refusal to produce
harmful responses. Through extensive experiments, we demonstrate the efficacy
of ICA and ICD in respectively elevating and mitigating the success rates of
jailbreaking prompts. Moreover, we offer theoretical insights into the
mechanism by which a limited set of in-context demonstrations can pivotally
influence the safety alignment of LLMs. Our findings illuminate the profound
influence of ICL on LLM behavior, opening new avenues for improving the safety
and alignment of LLMs.
更多查看译文
AI 理解论文
溯源树
样例
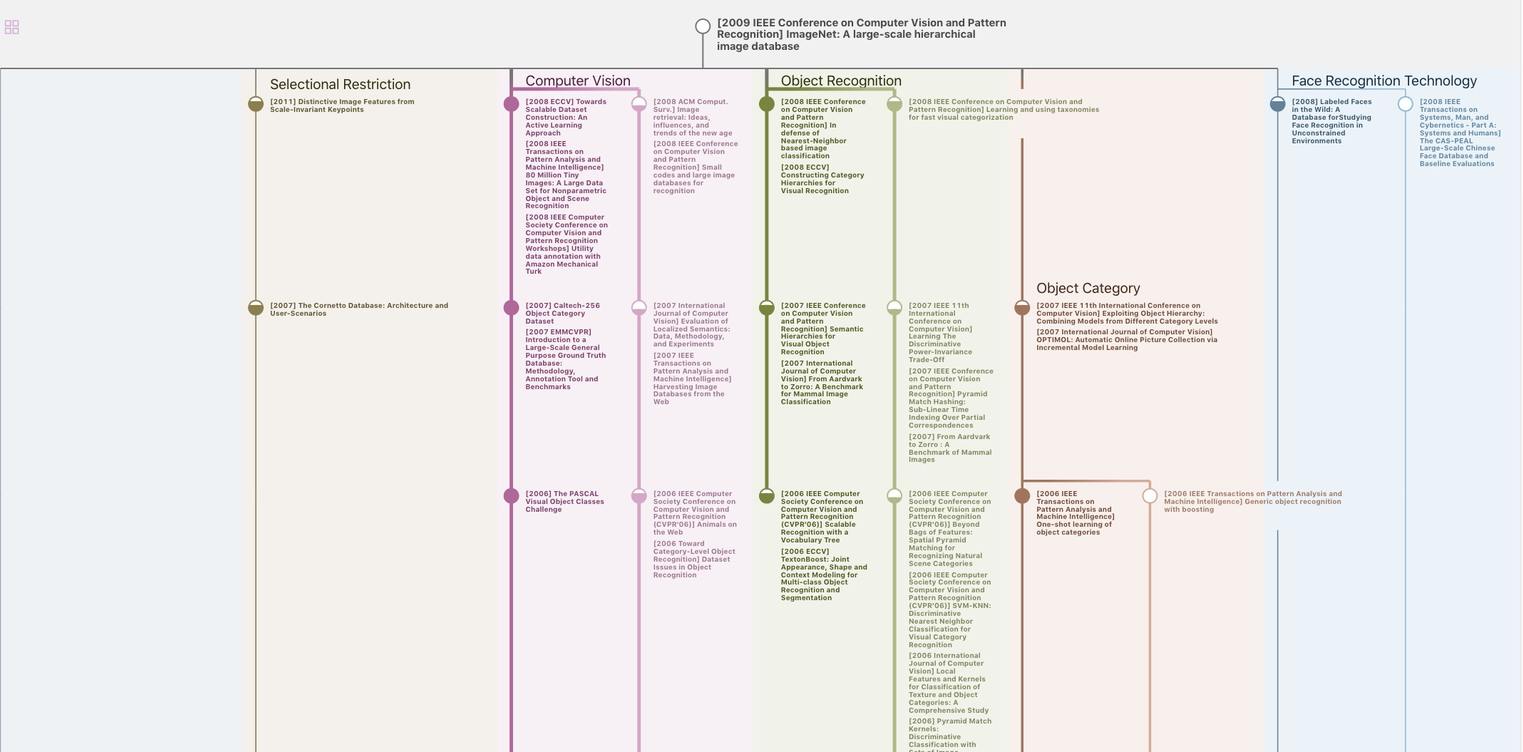
生成溯源树,研究论文发展脉络
Chat Paper
正在生成论文摘要