Humans and language models diverge when predicting repeating text.
CoRR(2023)
摘要
Language models that are trained on the next-word prediction task have been shown to accurately model human behavior in word prediction and reading speed. In contrast with these findings, we present a scenario in which the performance of humans and LMs diverges. We collected a dataset of human next-word predictions for five stimuli that are formed by repeating spans of text. Human and GPT-2 LM predictions are strongly aligned in the first presentation of a text span, but their performance quickly diverges when memory (or in-context learning) begins to play a role. We traced the cause of this divergence to specific attention heads in a middle layer. Adding a power-law recency bias to these attention heads yielded a model that performs much more similarly to humans. We hope that this scenario will spur future work in bringing LMs closer to human behavior.
更多查看译文
关键词
language models,text
AI 理解论文
溯源树
样例
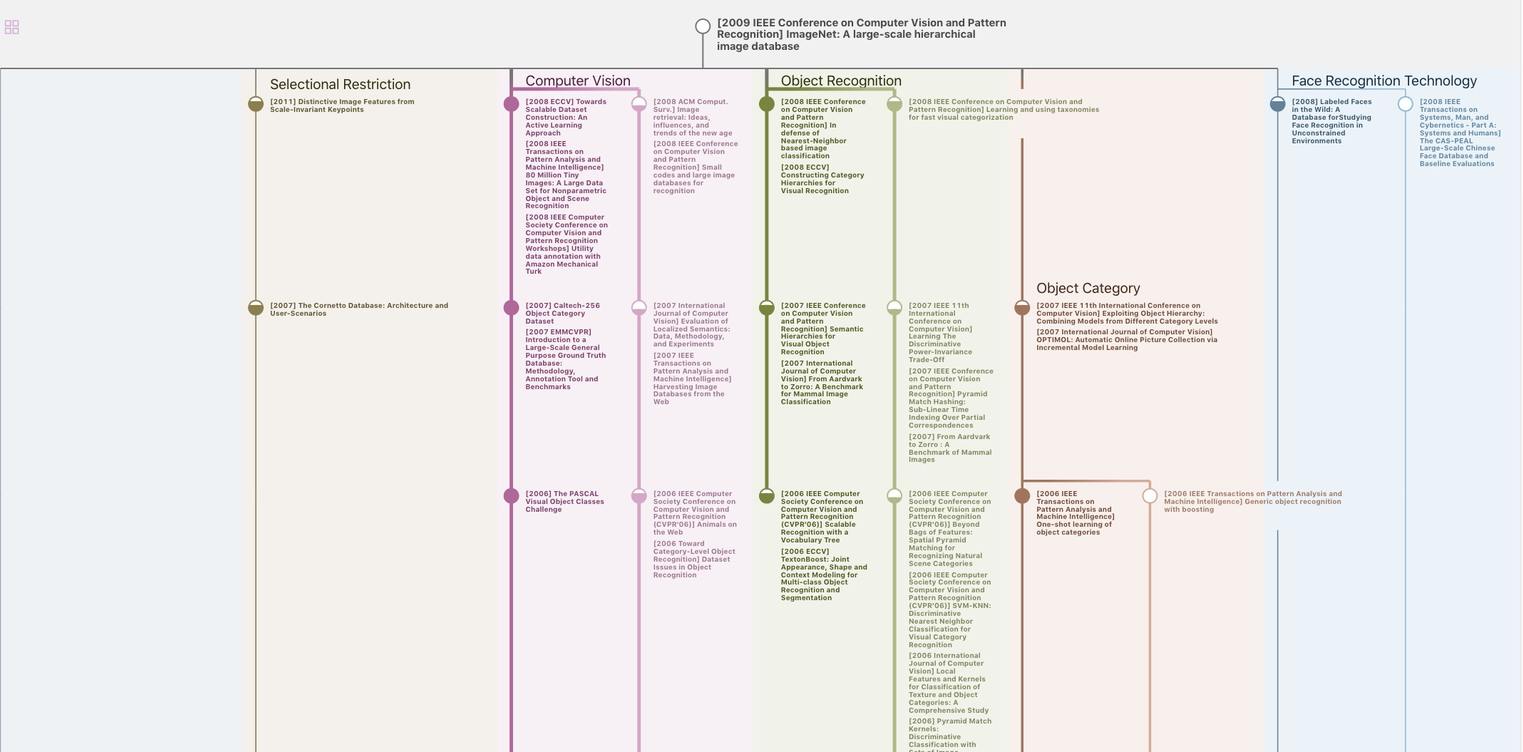
生成溯源树,研究论文发展脉络
Chat Paper
正在生成论文摘要