The Impact of Adversarial Attacks on Federated Learning: A Survey
IEEE TRANSACTIONS ON PATTERN ANALYSIS AND MACHINE INTELLIGENCE(2024)
摘要
Federated learning (FL) has emerged as a powerful machine learning technique that enables the development of models from decentralized data sources. However, the decentralized nature of FL makes it vulnerable to adversarial attacks. In this survey, we provide a comprehensive overview of the impact of malicious attacks on FL by covering various aspects such as attack budget, visibility, and generalizability, among others. Previous surveys have primarily focused on the multiple types of attacks and defenses but failed to consider the impact of these attacks in terms of their budget, visibility, and generalizability. This survey aims to fill this gap by providing a comprehensive understanding of the attacks' effect by identifying FL attacks with low budgets, low visibility, and high impact. Additionally, we address the recent advancements in the field of adversarial defenses in FL and highlight the challenges in securing FL. The contribution of this survey is threefold: first, it provides a comprehensive and up-to-date overview of the current state of FL attacks and defenses. Second, it highlights the critical importance of considering the impact, budget, and visibility of FL attacks. Finally, we provide ten case studies and potential future directions towards improving the security and privacy of FL systems.
更多查看译文
关键词
Adversarial attacks,and security challenges,attack status,attacks & defenses,budget,federated learning,generalizability,impact,online & offline attacks,real-world application domains,visibility
AI 理解论文
溯源树
样例
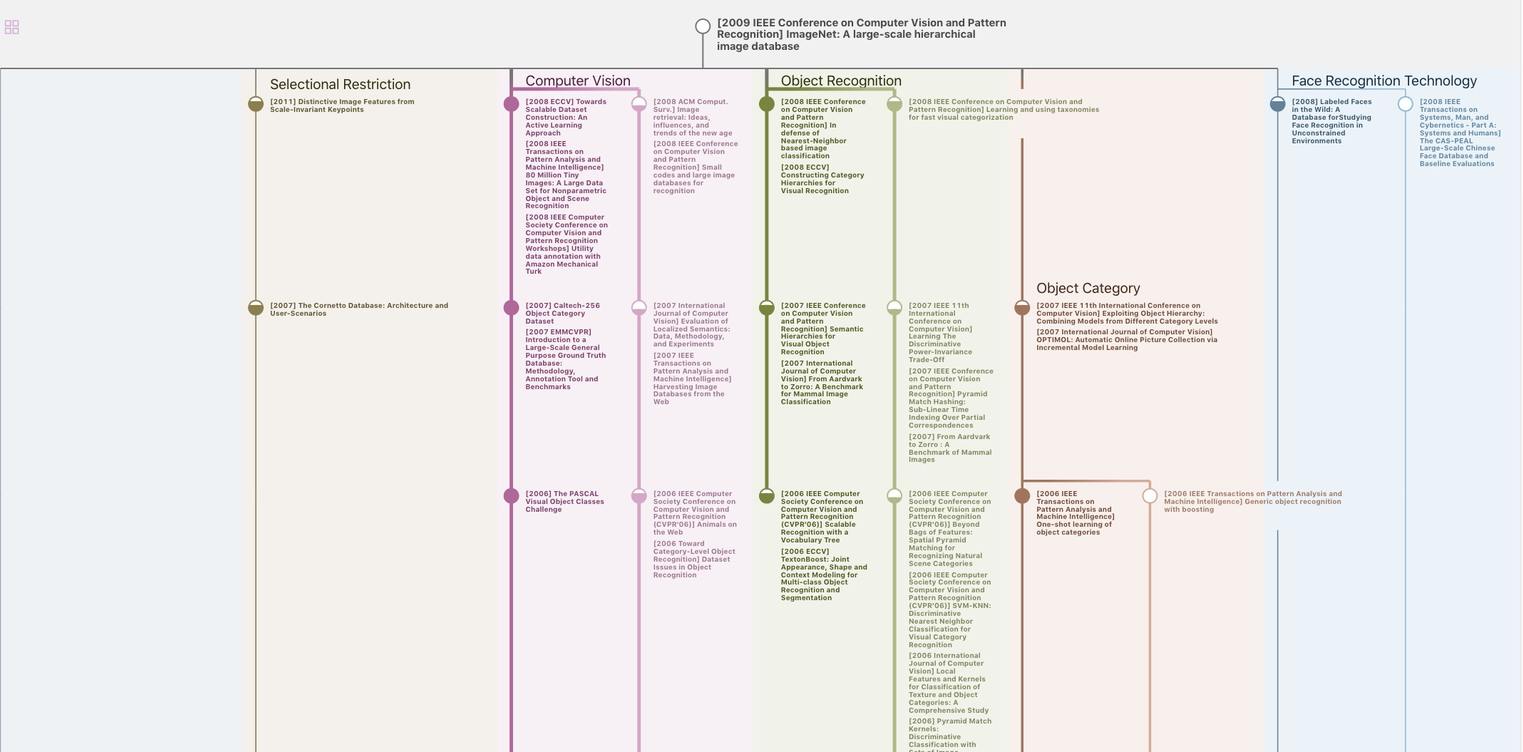
生成溯源树,研究论文发展脉络
Chat Paper
正在生成论文摘要