Response inhibition training as an intervention to modify liking and wanting for foods based on energy density: a proof of concept study
Journal of Behavioral Medicine(2024)
摘要
The ubiquity of energy-dense, processed foods has been implicated as a salient feature of the modern ‘obesogenic’ environment. Cognitive strategies, such as response inhibition training, have been demonstrated to reduce the hedonic value of such foods in previous studies. However, this effect has generally been inconsistent or heterogenous, depending on the outcome measure, characteristics of the sample, and the specificity of food stimuli. Characterising the extent of generalised effects may help define the application of this type of intervention in natural settings. A repeated-measures, proof-of-concept study, using mobile app-based response inhibition training (RIT) versus a control app-based activity ( N = 25), was undertaken to establish the valid application of a food reward measure to assess intervention efficacy. Liking (i.e., affect) and wanting (i.e., motivation) for food stimuli categorised by energy density were taken concurrently pre- and post-training. A statistically significant reduction in explicit liking, but not implicit wanting, for foods irrespective of their energy density was observed during the RIT app-based training session relative to the control ( p = .041, ηp 2 = .16). However, effect sizes associated with devaluation of energy-dense relative to low calorie food stimuli, although non-significant, were higher when measured as implicitly wanting ( p = .098, ηp 2 = .11) than explicit liking ( p = .756, ηp 2 = .00). Trends in explicit stimulus evaluations were empirically discordant from implicit evaluations for low calorie foods in particular. Additional research is needed to investigate whether these trends are reproducible with larger samples, trained and novel food stimuli in outcome measures, and more comprehensive training protocols.
更多查看译文
关键词
Response inhibition,Cognitive training,Food preference,Mobile application,Energy density,Food reward
AI 理解论文
溯源树
样例
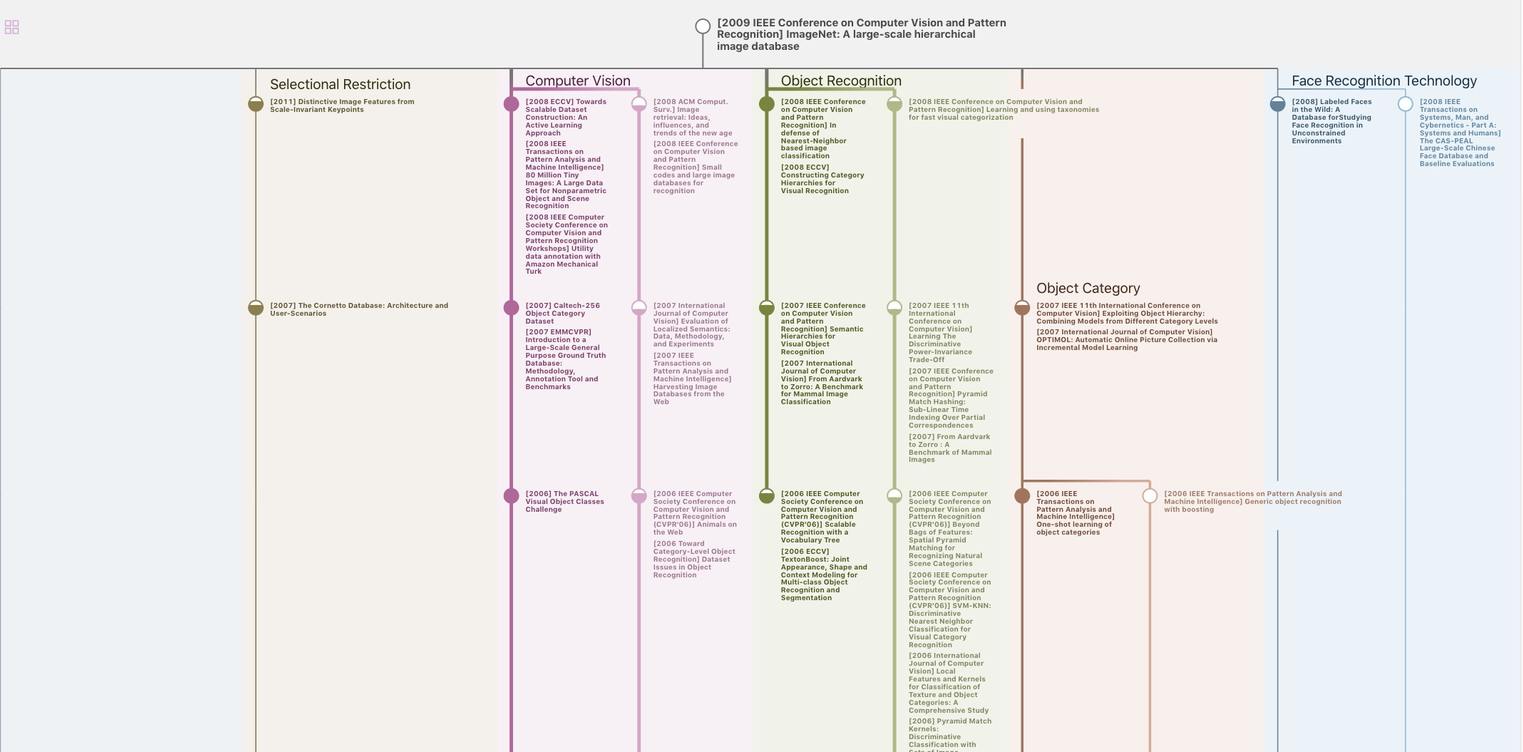
生成溯源树,研究论文发展脉络
Chat Paper
正在生成论文摘要