Saving Energy and Maintaining Indoor Comfort Level: A Reinforcement Learning Based Approach
2023 IEEE 11th International Conference on Smart Energy Grid Engineering (SEGE)(2023)
摘要
Studying building Occupant Behaviors (OB) for energy-saving presents an intriguing research problem. However, it appears that machine learning techniques have not been extensively investigated in this field. One particularly promising and underexplored approach is Reinforcement Learning (RL), which leverages rewards as a core concept. RL holds the potential to educate occupants about energy conservation and facilitate behavior adjustments. In our paper, we propose the application of RL and its variants to empower building occupants in optimizing their electricity consumption while maintaining indoor comfort. Our envisioned RL framework involves an agent interacting with the building environment through tailored states, rewards, and actions. The primary objective is to derive an optimal policy for OB, enabling occupants to make well-informed decisions and adopt energy-efficient behaviors. To evaluate the effectiveness of our approach, we conducted an empirical study using various energy consumption scenarios reflecting different environment complexities. The results were promising, demonstrating a significant reduction in consumption while preserving maximum comfort levels.
更多查看译文
关键词
occupant behaviors,reinforcement learning,Double DQN,energy consumption optimization,indoor comfort
AI 理解论文
溯源树
样例
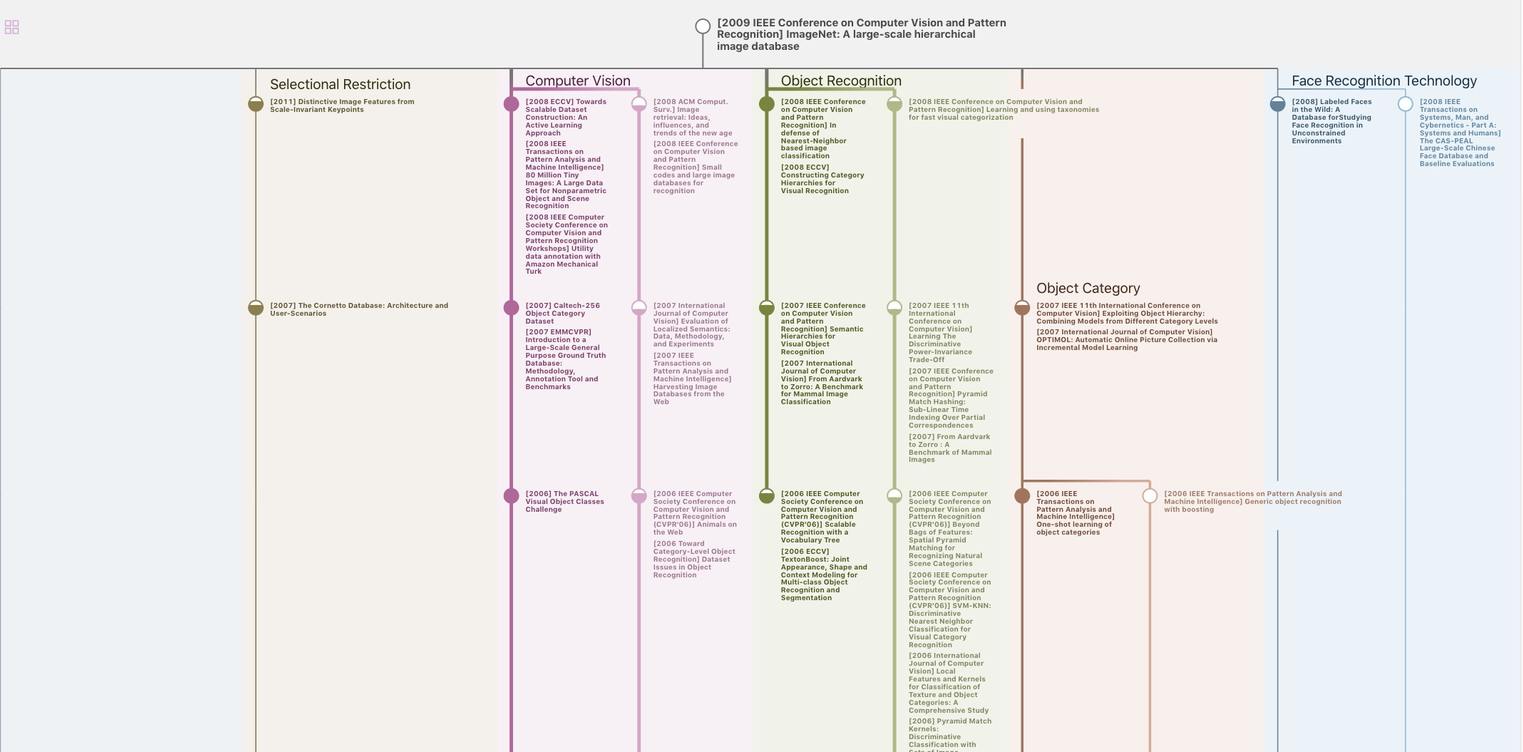
生成溯源树,研究论文发展脉络
Chat Paper
正在生成论文摘要