Deep Learning-Based Wideband Spectrum Sensing: A Low Computational Complexity Approach
IEEE Communications Letters(2023)
摘要
One of the key challenges in wideband spectrum sensing is that it has to be performed with extremely low latency and high accuracy over a large band to detect tiny spectrum holes. Motivated by this, we introduce a parallel convolutional neural network (ParallelCNN)-based wideband spectrum sensing approach, which aims to detect tiny spectrum holes with high sensing accuracy and low latency. The ParallelCNN contains two parallel CNNs, where the I/Q samples are split into two parts and processed concurrently by the two CNNs. Afterwards, the outputs of the two CNNs are combined to improve spectrum sensing performance. We extensively evaluate the accuracy, latency and generality performance of ParallelCNN. Performance evaluations indicate that the ParallelCNN approach outperforms other deep learning (DL)-based approaches such as the DeepSense and TCN, in terms of a higher precision, a higher recall and a lower latency, under a wide range of signal-to-noise (SNR) levels. Strikingly, the latency can be reduced by up to 94%. In addition, the ParallelCNN can be generalized to different protocols such as LTE-M uplink transmissions, 5G NR downlink transmissions and WiFi transmissions.
更多查看译文
关键词
Cognitive radio,wideband spectrum sensing,deep learning,convolutional neural network,latency
AI 理解论文
溯源树
样例
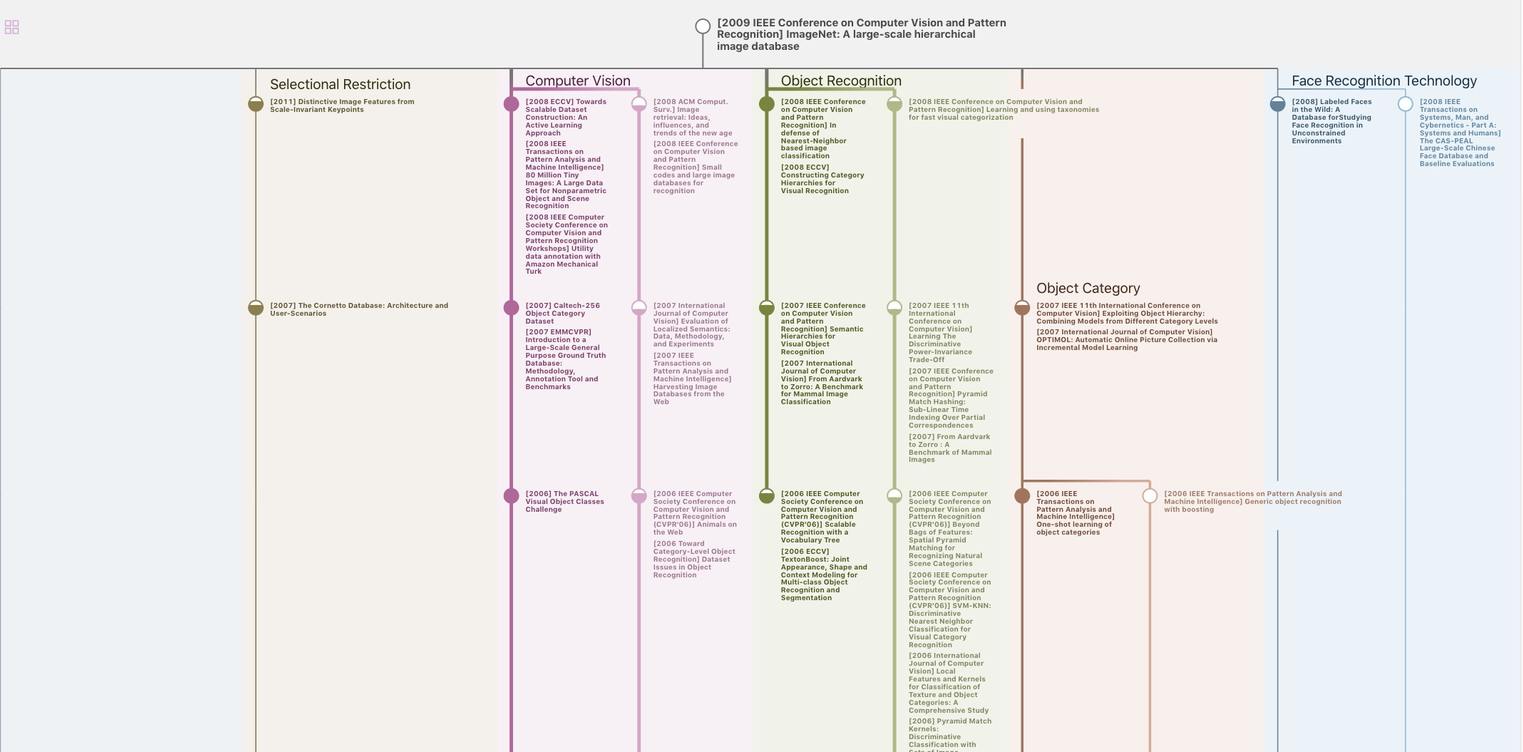
生成溯源树,研究论文发展脉络
Chat Paper
正在生成论文摘要