Key Indicators to Assess the Performance of LiDAR-Based Perception Algorithms: A Literature Review
IEEE Access(2023)
摘要
Perception algorithms are essential for autonomous or semi-autonomous vehicles to perceive the semantics of their surroundings, including object detection, panoptic segmentation, and tracking. Decision-making in case of safety-critical situations, like autonomous emergency braking and collision avoidance, relies on the outputs of these algorithms. This makes it essential to correctly assess such perception systems before their deployment and to monitor their performance when in use. It is difficult to test and validate these systems, particularly at runtime, due to the high-level and complex representations of their outputs. This paper presents an overview of different existing metrics used for the evaluation of LiDAR-based perception systems, emphasizing particularly object detection and tracking algorithms due to their importance in the final perception outcome. Along with generally used metrics, we also discuss the impact of Planning KL-Divergence (PKL), Timed Quality Temporal Logic (TQTL), and Spatio-temporal Quality Logic (STQL) metrics on object detection algorithms. In the case of panoptic segmentation, Panoptic Quality (PQ) and Parsing Covering (PC) metrics are analysed resorting to some pretrained models. Finally, it addresses the application of diverse metrics to evaluate different pretrained models with the respective perception algorithms on publicly available datasets. Besides the identification of the various metrics being proposed, their performance and influence on models are also assessed after conducting new tests or reproducing the experimental results of the reference under consideration.
更多查看译文
关键词
Perception algorithms, metrics, deep learning, object detection, panoptic segmentation, autonomous driving
AI 理解论文
溯源树
样例
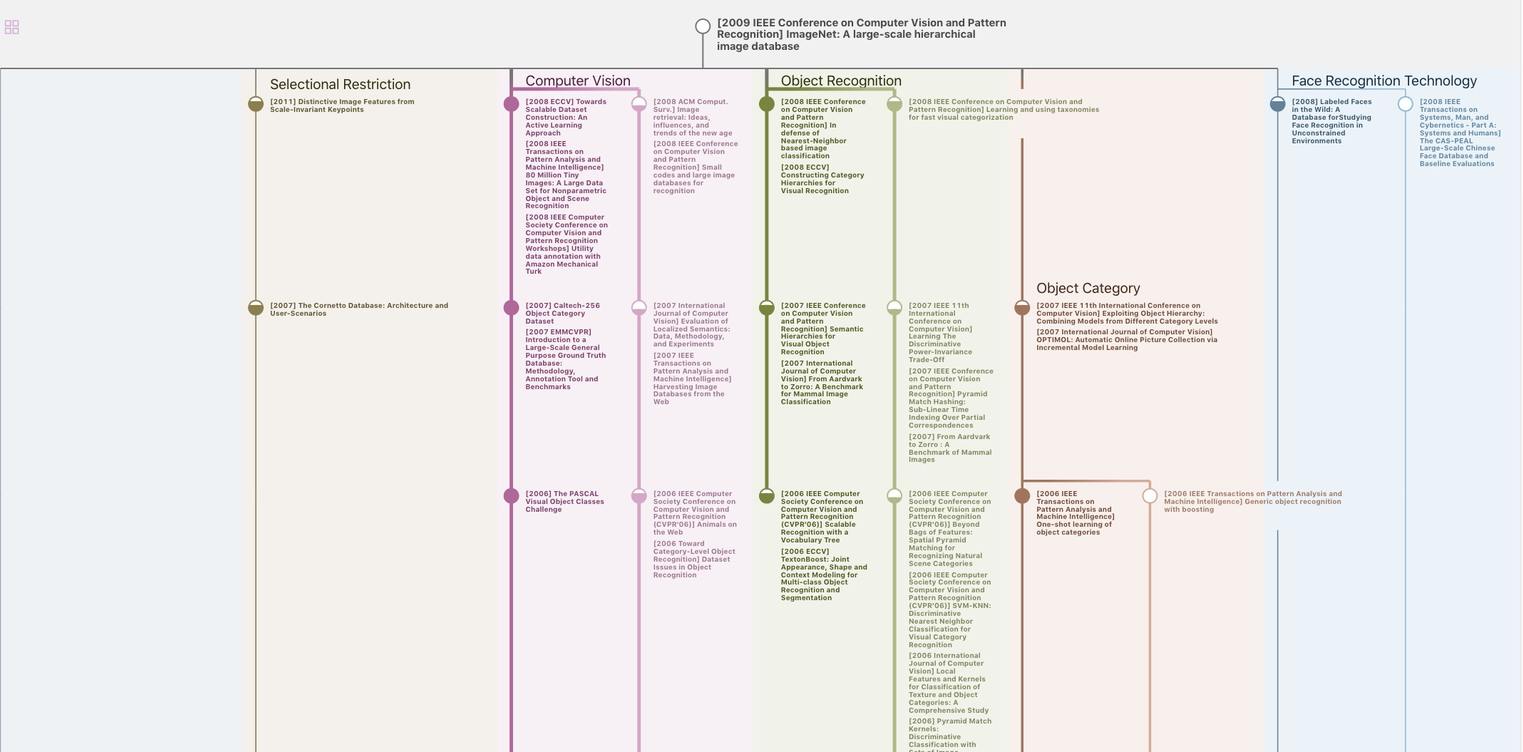
生成溯源树,研究论文发展脉络
Chat Paper
正在生成论文摘要