Synthesizing Missing MRI Sequences from Available Modalities using Generative Adversarial Networks in BraTS Dataset
arXiv (Cornell University)(2023)
摘要
Glioblastoma is a highly aggressive and lethal form of brain cancer. Magnetic resonance imaging (MRI) plays a significant role in the diagnosis, treatment planning, and follow-up of glioblastoma patients due to its non-invasive and radiation-free nature. The International Brain Tumor Segmentation (BraTS) challenge has contributed to generating numerous AI algorithms to accurately and efficiently segment glioblastoma sub-compartments using four structural (T1, T1Gd, T2, T2-FLAIR) MRI scans. However, these four MRI sequences may not always be available. To address this issue, Generative Adversarial Networks (GANs) can be used to synthesize the missing MRI sequences. In this paper, we implement and utilize an open-source GAN approach that takes any three MRI sequences as input to generate the missing fourth structural sequence. Our proposed approach is contributed to the community-driven generally nuanced deep learning framework (GaNDLF) and demonstrates promising results in synthesizing high-quality and realistic MRI sequences, enabling clinicians to improve their diagnostic capabilities and support the application of AI methods to brain tumor MRI quantification.
更多查看译文
关键词
generative adversarial networks,mri sequences,dataset
AI 理解论文
溯源树
样例
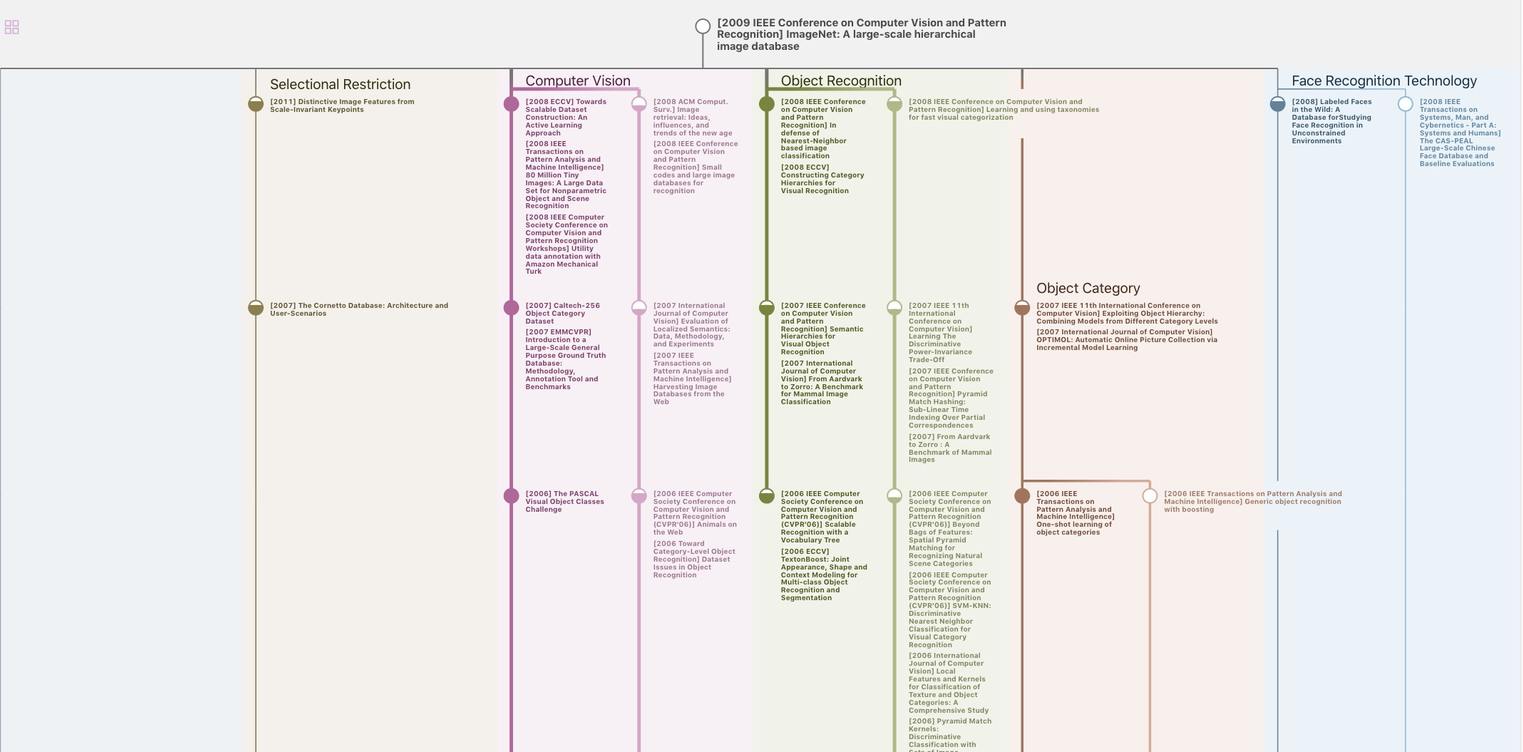
生成溯源树,研究论文发展脉络
Chat Paper
正在生成论文摘要