DUO: Stealthy Adversarial Example Attack on Video Retrieval Systems via Frame-Pixel Search
2023 IEEE 43rd International Conference on Distributed Computing Systems (ICDCS)(2023)
摘要
Massive videos are released every day particularly through video-focused social media apps such as TikTok. This trend has fostered the quick emergence of video retrieval systems, which provide cloud-based services to retrieve similar videos using machine learning techniques. Adversarial example (AE) attacks have been shown to be effective on such systems by perturbing an unaltered video subtly to induce false retrieval results. Such AE attacks can be easily detected because the adversarial perturbations are all over pixels and frames. In this paper, we propose DUO, a stealthy targeted black-box AE attack which uses DUal search Over frame-pixel to generate sparse perturbations and improve stealthiness. DUO is motivated by two observations: only “key frames” in a video decide model predictions, and different pixels and frames contribute far differently to AEs. We implement DUO into a sequential attack pipeline consisting of two components (i.e., SparseTransfer and SparseQuery) built upon such intuitions. In particular, DUO uses SparseTransfer to generate initial perturbations and then SparseQuery to further rectify them. Extensive evaluations on two popular datasets confirm the higher efficacy and stealthiness of DUO over existing AE attacks on video retrieval systems. In particular, we show that DUO achieves higher precision while significantly reducing adversarial perturbations by more than ×100 than the state-of-the-art AE attack.
更多查看译文
关键词
sparse targeted adversarial example attack,video retrieval system,black-box attack,stealthiness
AI 理解论文
溯源树
样例
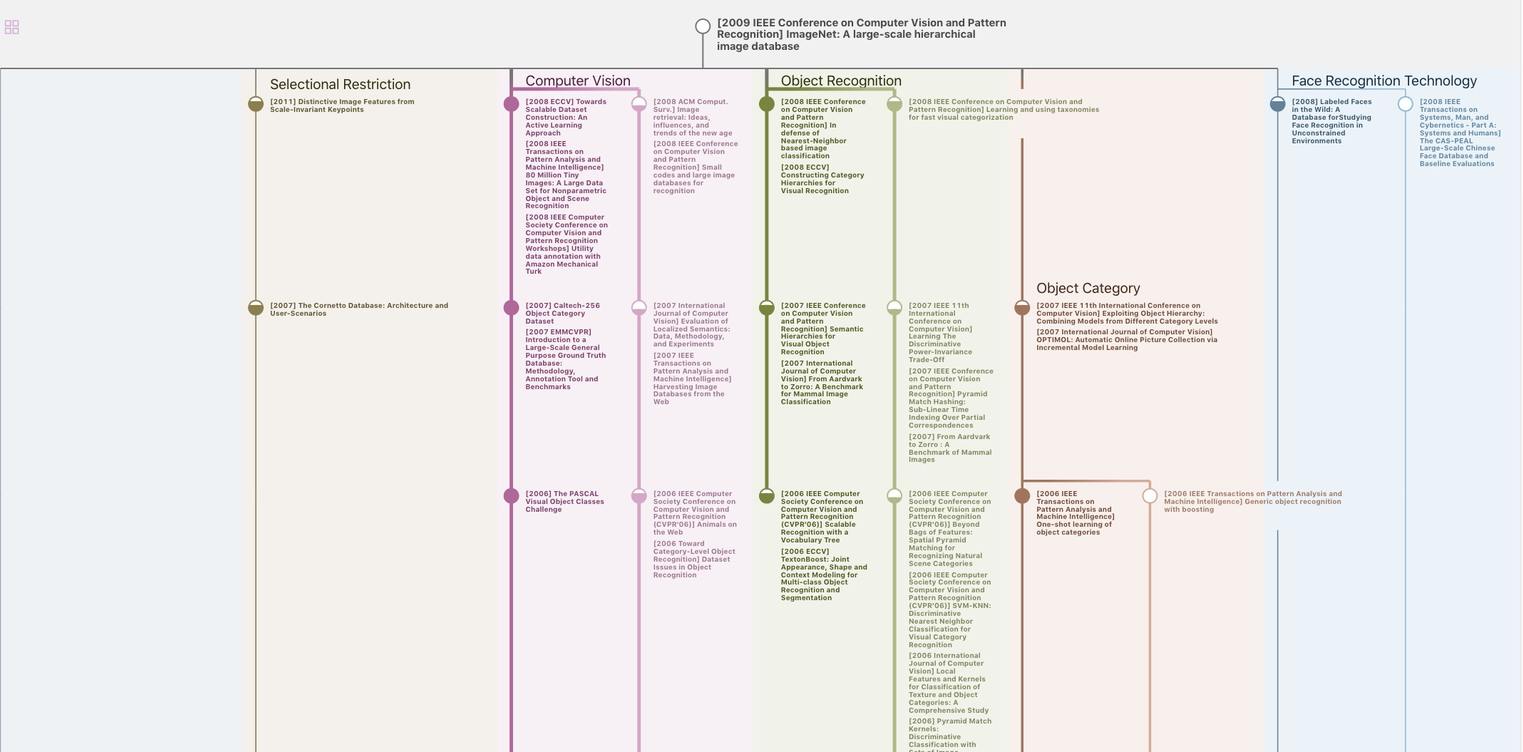
生成溯源树,研究论文发展脉络
Chat Paper
正在生成论文摘要