An automated approach for improving the inference latency and energy efficiency of pretrained CNNs by removing irrelevant pixels with focused convolutions
2024 29th Asia and South Pacific Design Automation Conference (ASP-DAC)(2023)
摘要
Computer vision often uses highly accurate Convolutional Neural Networks (CNNs), but these deep learning models are associated with ever-increasing energy and computation requirements. Producing more energy-efficient CNNs often requires model training which can be cost-prohibitive. We propose a novel, automated method to make a pretrained CNN more energy-efficient without re-training. Given a pretrained CNN, we insert a threshold layer that filters activations from the preceding layers to identify regions of the image that are irrelevant, i.e. can be ignored by the following layers while maintaining accuracy. Our modified focused convolution operation saves inference latency (by up to 25%) and energy costs (by up to 22%) on various popular pretrained CNNs, with little to no loss in accuracy.
更多查看译文
关键词
cnns,irrelevant pixels,inference latency,efficiency,focused
AI 理解论文
溯源树
样例
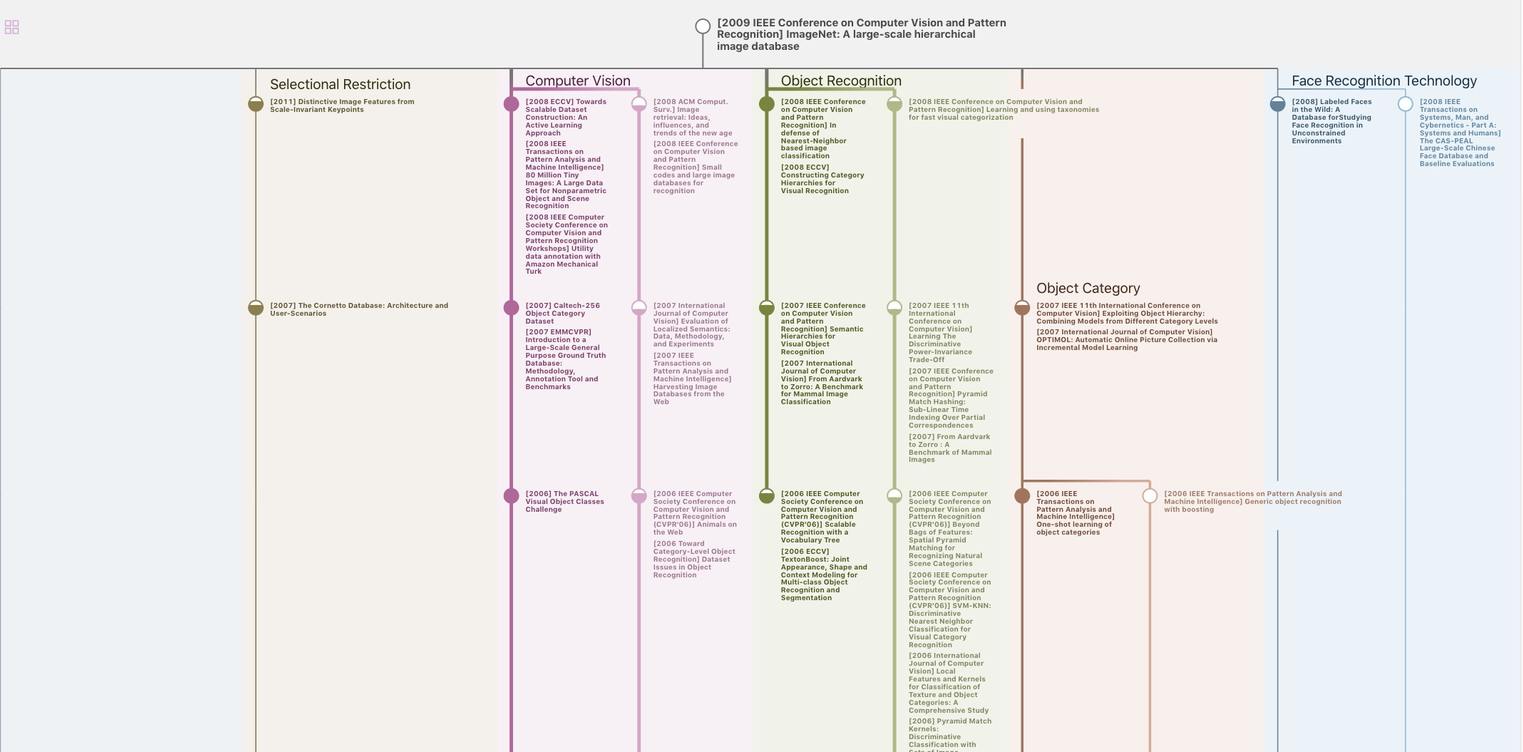
生成溯源树,研究论文发展脉络
Chat Paper
正在生成论文摘要