Non-Stationary Contextual Bandit Learning via Neural Predictive Ensemble Sampling
CoRR(2023)
摘要
Real-world applications of contextual bandits often exhibit non-stationarity due to seasonality, serendipity, and evolving social trends. While a number of non-stationary contextual bandit learning algorithms have been proposed in the literature, they excessively explore due to a lack of prioritization for information of enduring value, or are designed in ways that do not scale in modern applications with high-dimensional user-specific features and large action set, or both. In this paper, we introduce a novel non-stationary contextual bandit algorithm that addresses these concerns. It combines a scalable, deep-neural-network-based architecture with a carefully designed exploration mechanism that strategically prioritizes collecting information with the most lasting value in a non-stationary environment. Through empirical evaluations on two real-world recommendation datasets, which exhibit pronounced non-stationarity, we demonstrate that our approach significantly outperforms the state-of-the-art baselines.
更多查看译文
关键词
neural predictive ensemble
AI 理解论文
溯源树
样例
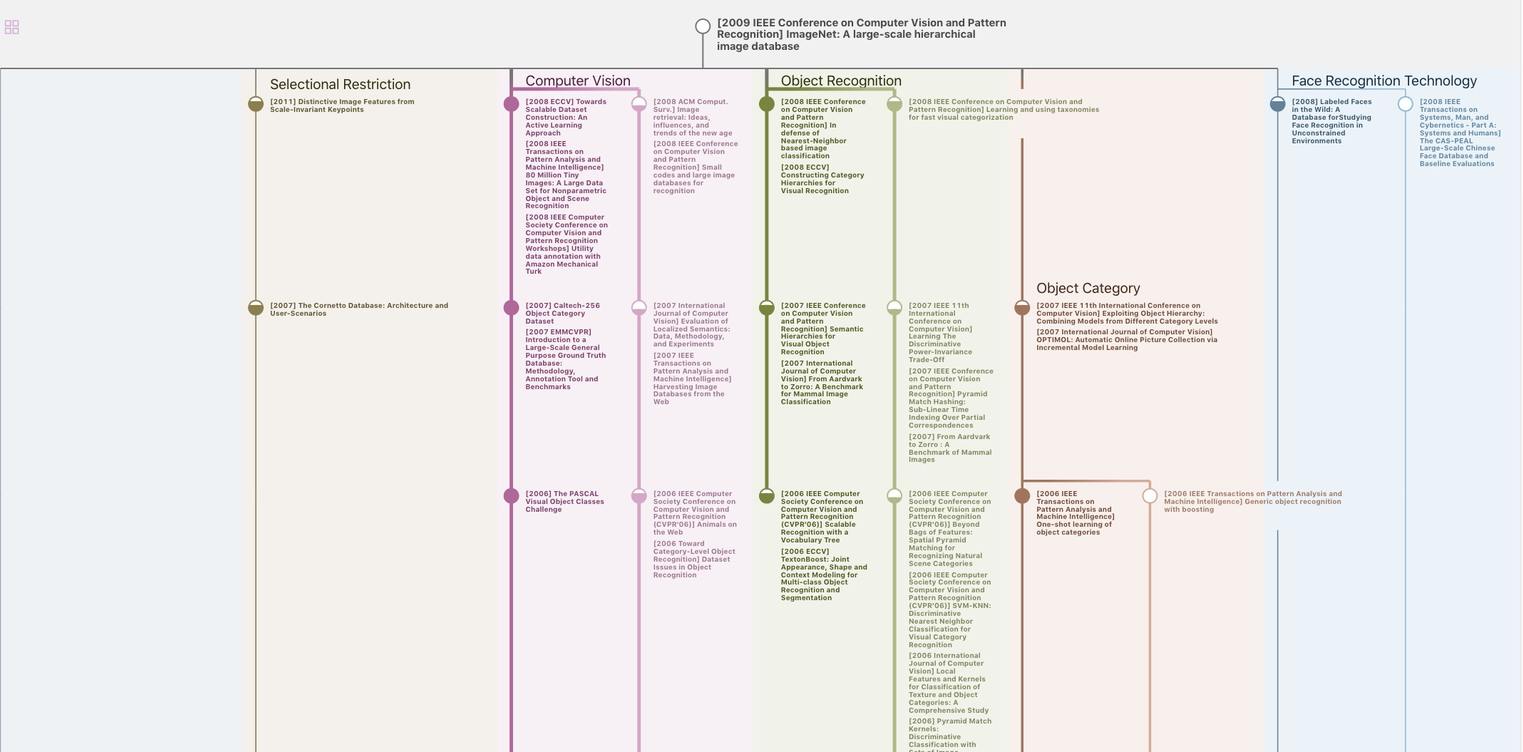
生成溯源树,研究论文发展脉络
Chat Paper
正在生成论文摘要