Revisiting Decentralized ProxSkip: Achieving Linear Speedup
arxiv(2023)
摘要
The ProxSkip algorithm for decentralized and federated learning is gaining
increasing attention due to its proven benefits in accelerating communication
complexity while maintaining robustness against data heterogeneity. However,
existing analyses of ProxSkip are limited to the strongly convex setting and do
not achieve linear speedup, where convergence performance increases linearly
with respect to the number of nodes. So far, questions remain open about how
ProxSkip behaves in the non-convex setting and whether linear speedup is
achievable.
In this paper, we revisit decentralized ProxSkip and address both questions.
We demonstrate that the leading communication complexity of ProxSkip is
𝒪(pσ^2/nϵ^2) for non-convex and
convex settings, and 𝒪(pσ^2/nϵ) for
the strongly convex setting, where n represents the number of nodes, p
denotes the probability of communication, σ^2 signifies the level of
stochastic noise, and ϵ denotes the desired accuracy level. This
result illustrates that ProxSkip achieves linear speedup and can asymptotically
reduce communication overhead proportional to the probability of communication.
Additionally, for the strongly convex setting, we further prove that ProxSkip
can achieve linear speedup with network-independent stepsizes.
更多查看译文
AI 理解论文
溯源树
样例
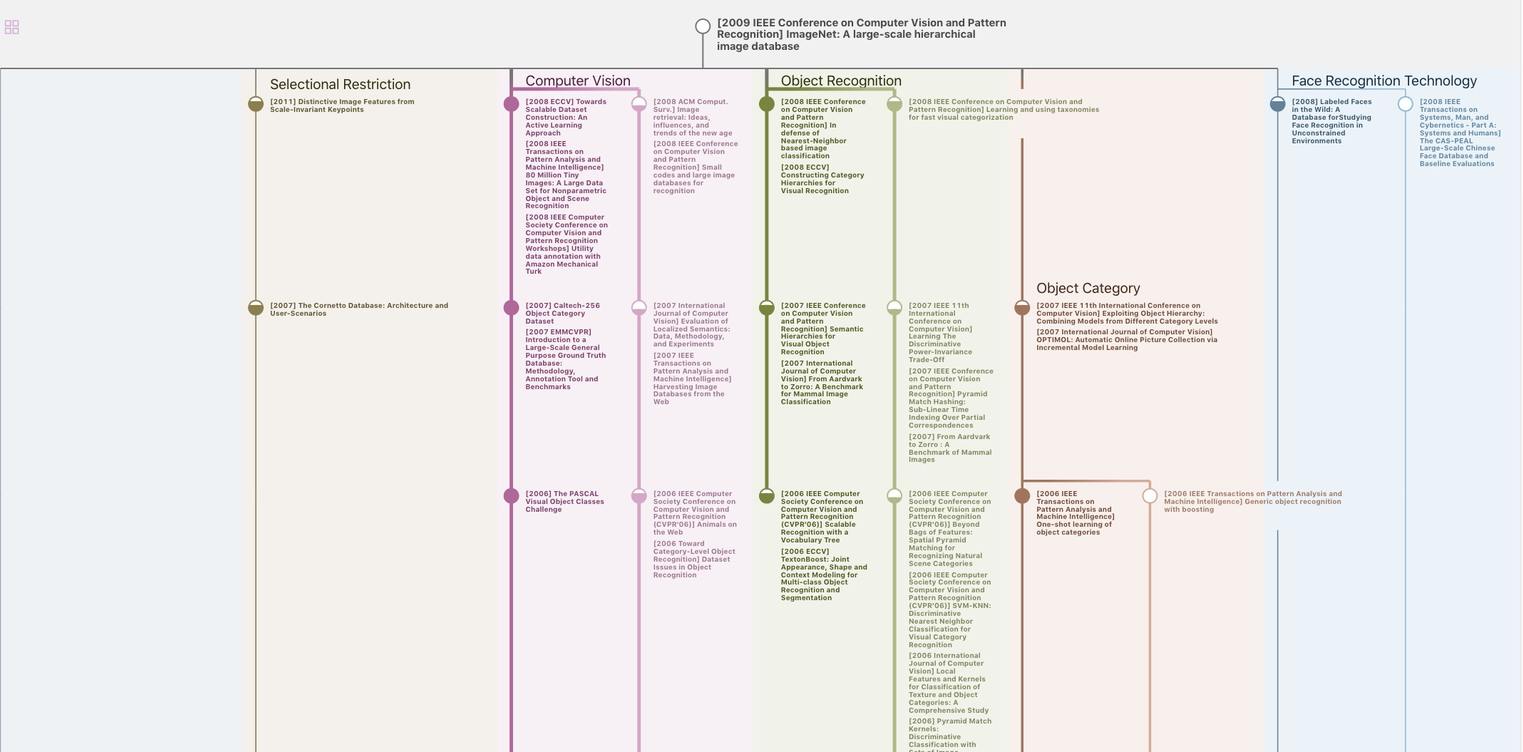
生成溯源树,研究论文发展脉络
Chat Paper
正在生成论文摘要