Lattice real-time simulations with learned optimal kernels
PHYSICAL REVIEW D(2024)
摘要
We present a simulation strategy for the real-time dynamics of quantum fields, inspired by reinforcement learning. It builds on the complex Langevin approach, which it amends with system -specific prior information, a necessary prerequisite to overcome this exceptionally severe sign problem. The optimization process underlying our machine -learning approach is made possible by deploying inherently stable solvers of the complex Langevin stochastic process and a novel optimality criterion derived from insight into socalled boundary terms. This conceptual and technical progress allows us to both significantly extend the range of real-time simulations in 1 + 1d scalar field theory beyond the state of the art and to avoid discretization artifacts that plagued previous real-time field theory simulations. Limitations of and promising future directions are discussed.
更多查看译文
关键词
simulations,real-time
AI 理解论文
溯源树
样例
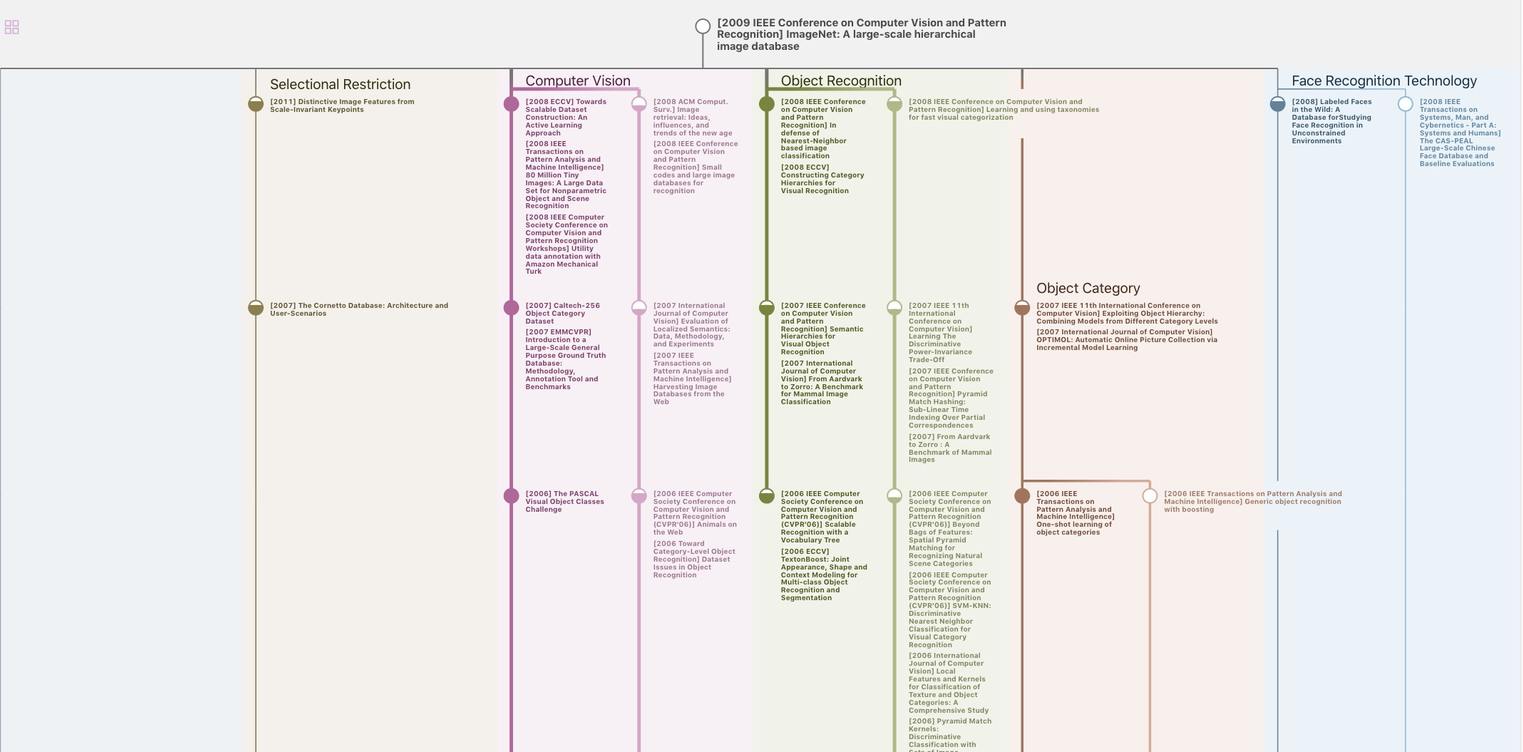
生成溯源树,研究论文发展脉络
Chat Paper
正在生成论文摘要