Training Generative Question-Answering on Synthetic Data Obtained from an Instruct-tuned Mo
CoRR(2023)
摘要
This paper presents a simple and cost-effective method for synthesizing data to train question-answering systems. For training, fine-tuning GPT models is a common practice in resource-rich languages like English, however, it becomes challenging for non-English languages due to the scarcity of sufficient question-answer (QA) pairs. Existing approaches use question and answer generators trained on human-authored QA pairs, which involves substantial human expenses. In contrast, we use an instruct-tuned model to generate QA pairs in a zero-shot or few-shot manner. We conduct experiments to compare various strategies for obtaining QA pairs from the instruct-tuned model. The results demonstrate that a model trained on our proposed synthetic data achieves comparable performance to a model trained on manually curated datasets, without incurring human costs.
更多查看译文
关键词
synthetic data,question-answering,instruct-tuned
AI 理解论文
溯源树
样例
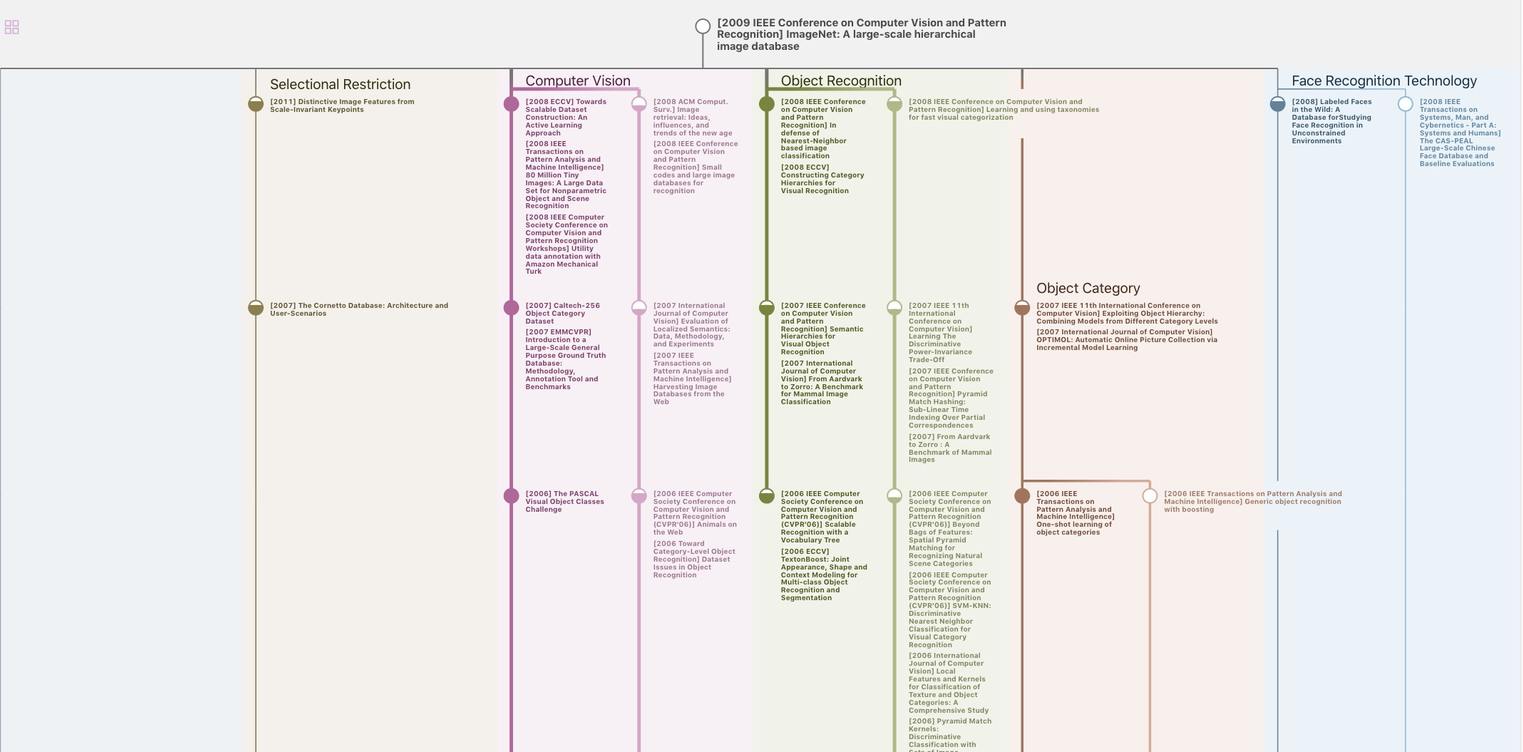
生成溯源树,研究论文发展脉络
Chat Paper
正在生成论文摘要